Fully Convolutional Neural Network For Impervious Surface Segmentation In Mixed Urban Environment
PHOTOGRAMMETRIC ENGINEERING AND REMOTE SENSING(2021)
摘要
The urgency of creating appropriate, high-resolution data products such as impervious cover information has increased as cities face rapid growth as well as climate change and other environmental challenges. This work explores the use of fully convolutional neural networks (FCNNs)-specifically UNet with a ResNet-152 encoder-in mapping impervious surfaces at the pixel level from World View-2 in a mixed urban/residential environment. We investigate three-, four-, and eight-band multispectral inputs to the FCNN. Resulting maps are promising in both qualitative and quantitative assessment when compared to automated land use/land cover products. Accuracy was assessed by F1 and average precision (AP) scores, as well as receiver operating characteristic curves, with area under the curve (AUC) used as an additional accuracy metric. The four-band model shows the highest average test-set accuracies (F1, Al, and AUC of 0.709, 0.82, and 0.807, respectively), with higher AP and AUG than the automated land use/land cover products, indicating the utility of the blue-green-red-infrared channels for the FCNN. Improved performance was seen in residential areas, with worse performance in more densely developed areas.
更多查看译文
AI 理解论文
溯源树
样例
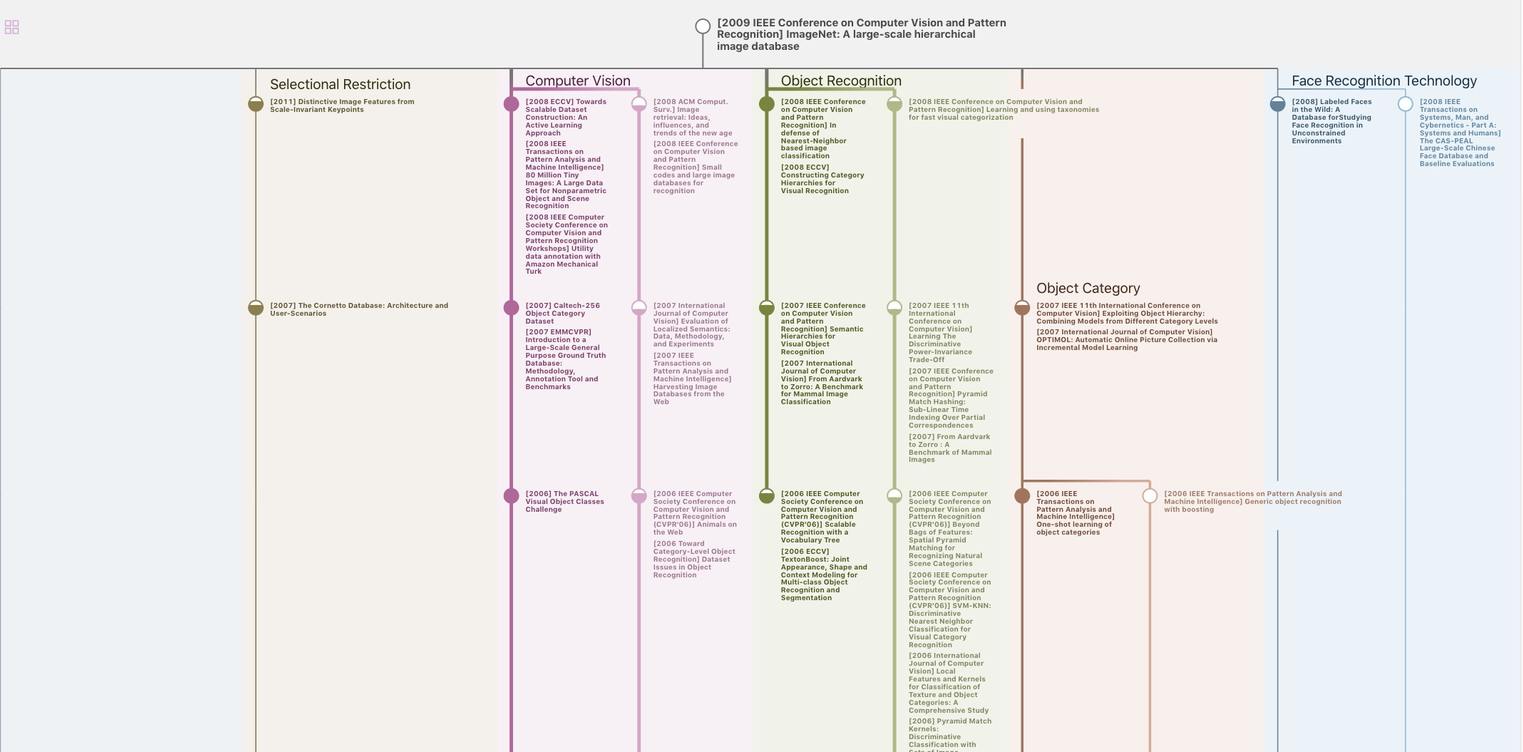
生成溯源树,研究论文发展脉络
Chat Paper
正在生成论文摘要