Automated Defect Recognition on X-ray Radiographs of Solid Propellant Using Deep Learning Based on Convolutional Neural Networks
JOURNAL OF NONDESTRUCTIVE EVALUATION(2021)
摘要
For defense applications, rapid X-ray inspection of propellant samples is essential for the identification and assessment of defects. Automation of this process using artificial intelligence is possible by properly training a neural network model. Convolution Neural Networks (CNNs) have recently demonstrated excellent success in both the tasks of image recognition and localisation using an adequate amount of data. In real-world, it’s not an easy task to produce the correct amount of experimental data required for the deep neural network to operate. In this work, we propose a method for producing synthetic radiographic data that is supported by ray tracing based radiographic simulations for the deep learning algorithms to automatically detect anomaly in X-ray images. The simulation results, which are then supplemented by noise extracted from the experimental data, show a good comparison with the measurements. This Simulation assisted Automatic Defect Recognition (Sim-ADR) system simultaneously perform defect detection and defect instance segmentation. The accuracy of the defect detection system is more than 87% on a testing set included 416 images.
更多查看译文
关键词
Ray casting, Radiography, Mask RCNN, Deep learning, Automatic defect recognition (ADR)
AI 理解论文
溯源树
样例
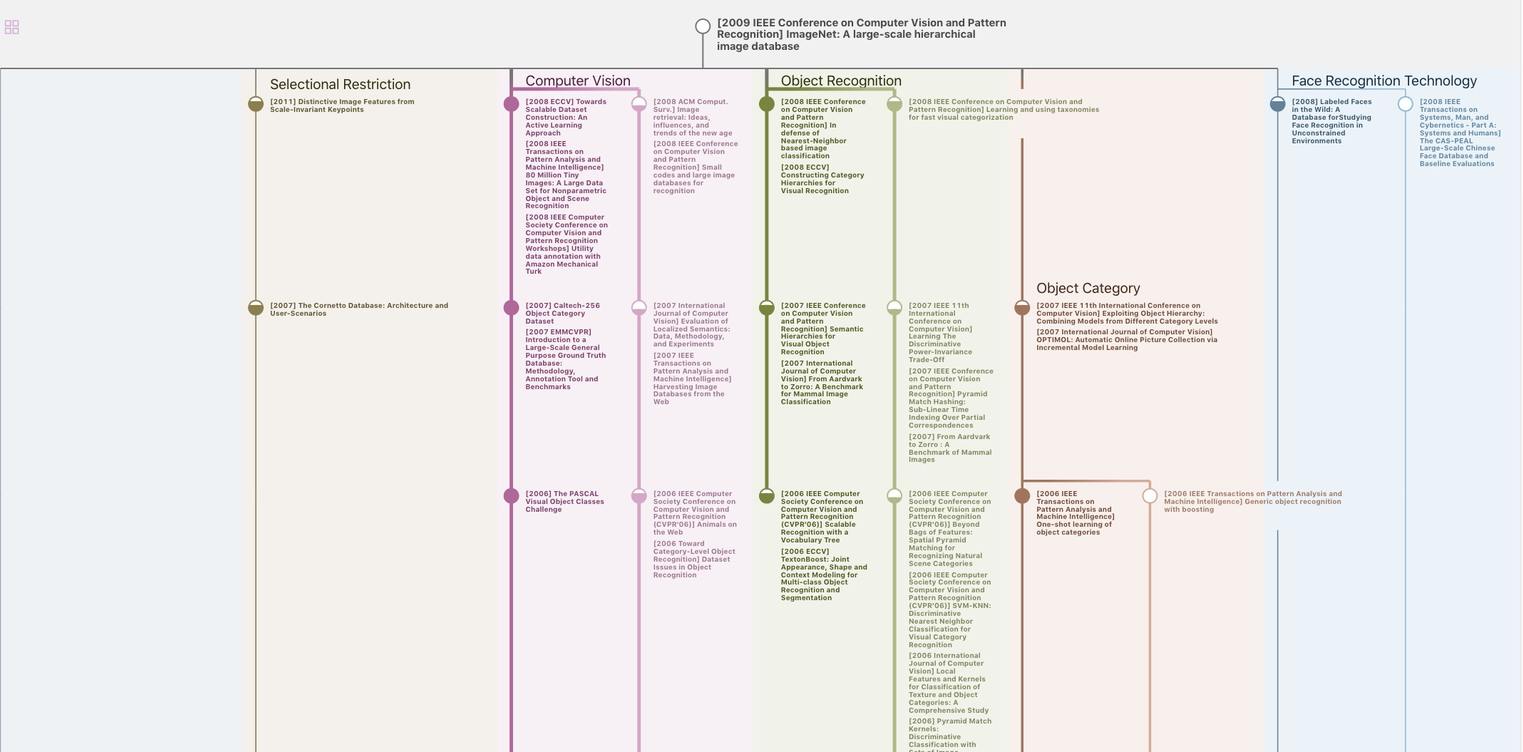
生成溯源树,研究论文发展脉络
Chat Paper
正在生成论文摘要