A Cost-Effective Manufacturing Process Recognition Approach Based On Deep Transfer Learning For Cps Enabled Shop-Floor
ROBOTICS AND COMPUTER-INTEGRATED MANUFACTURING(2021)
摘要
The rapid development of the industrial Internet of Things has promoted manufacturing to develop towards the cyber-physical system, of which highly accurate process recognition plays an important role in achieving proactive monitoring of intelligent manufacturing process. Compared to the traditional handcrafted feature-based method, deep model owns convenience in terms of extracting feature automatically for the recognition. However, training a deep model is time-consuming and also requires large-scale training samples. To solve these problems and obtain high accuracy in the meanwhile, a deep transfer learning-based manufacturing process recognition approach is proposed in this study. A pre-trained model based on a convolutional neural network is used to extract low dimensional features followed by a fine-tuning process to target the specific process recognition task. Experimental verification of two datasets was conducted to demonstrate this cost-effective method. The results showed the proposed method can get better accuracy with less training time and fewer training samples.
更多查看译文
关键词
Manufacturing process recognition, Transfer learning (TL), Cyber-physical systems (CPS), Convolutional neural network (CNN), Deep learning (DL)
AI 理解论文
溯源树
样例
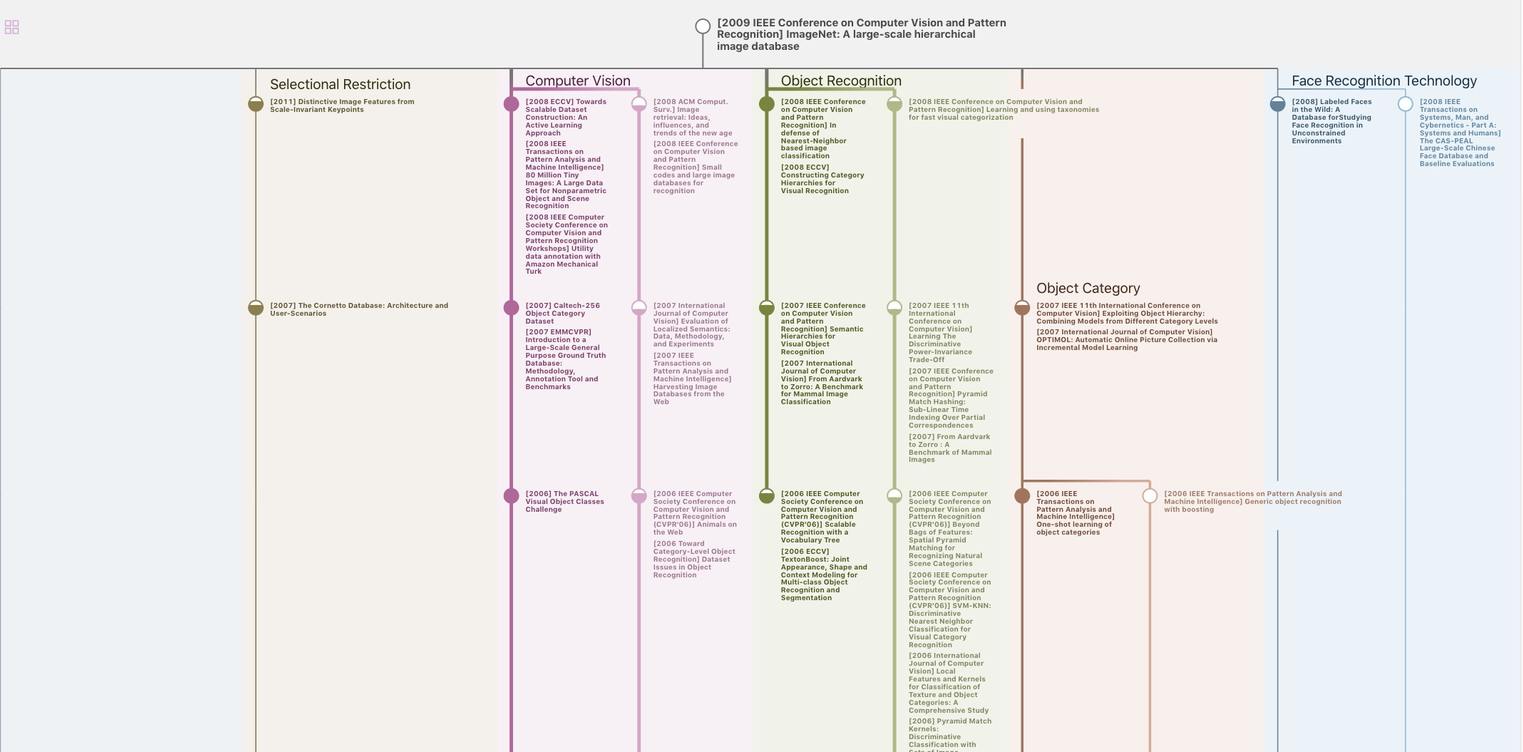
生成溯源树,研究论文发展脉络
Chat Paper
正在生成论文摘要