Text semantic understanding based on knowledge enhancement and multi-granular feature extraction
2020 Chinese Automation Congress (CAC)(2020)
摘要
The neural network model in natural language processing (NLP) has become the mainstream. Bidirectional Long Short-Term Memory Network (BiLSTM) has proved to be a very effective sequence model in text semantic understanding. The Siamese framework has achieved excellent performance in text semantic similarity calculation. However, the feature granularity obtained from BiLSTM is single, and more extensive information cannot be obtained using traditional neural networks. In order to solve these problems, text semantic understanding model based on knowledge enhancement and multi-granular feature extraction (KE-MGFE) is proposed. KE-MGFE integrates knowledge base and three granular text representations, and an attention mechanism is introduced in the sub-networks of the Siamese framework to enhance information interaction. KE-MGFE is formed to capture the deep entity relationships of text and the features of each granularity. Finally, experimental verifications are conducted on two long text datasets. The results clearly show that the proposed model KE-MGFE can achieve higher performance than other latest text similarity calculation methods.
更多查看译文
关键词
knowledge base,long short-term memory,attention mechanism,natural language processing,semantic similarity
AI 理解论文
溯源树
样例
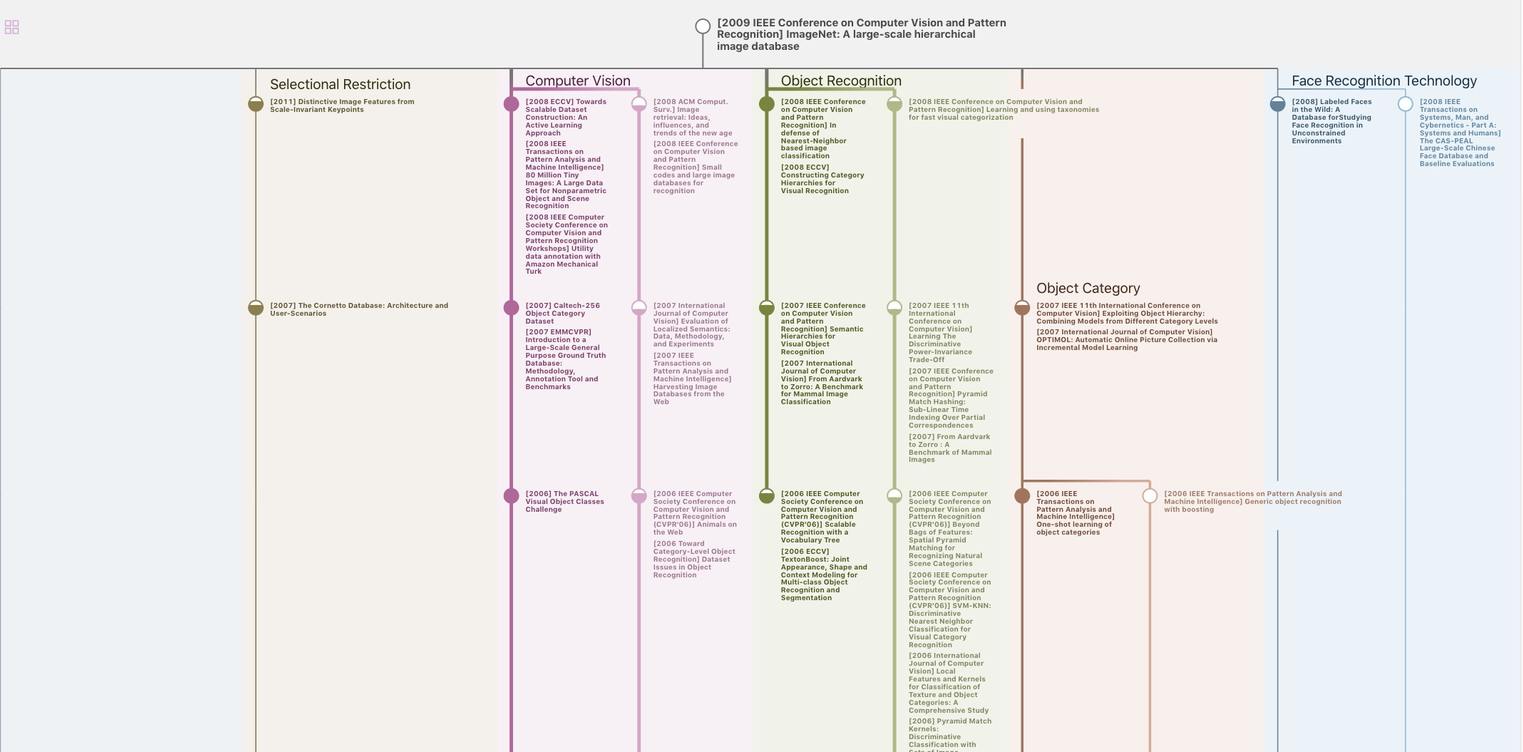
生成溯源树,研究论文发展脉络
Chat Paper
正在生成论文摘要