Online sequential extreme studentized deviate tests for anomaly detection in streaming data with varying patterns
CLUSTER COMPUTING-THE JOURNAL OF NETWORKS SOFTWARE TOOLS AND APPLICATIONS(2021)
摘要
In the new era of big data, numerous information and technology systems can store huge amounts of streaming data in real time, for example, in server-access logs on web application servers. The importance of anomaly detection in voluminous quantities of streaming data from such systems is rapidly increasing. One of the biggest challenges in the detection task is to carry out real-time contextual anomaly detection in streaming data with varying patterns that are visually detectable but unsuitable for a parametric model. Most anomaly detection algorithms have weaknesses in dealing with streaming time-series data containing such patterns. In this paper, we propose a novel method for online contextual anomaly detection in streaming time-series data using generalized extreme studentized deviates (GESD) tests. The GESD test is relatively accurate and efficient because it performs statistical hypothesis testing but it is unable to handle streaming time-series data. Thus, focusing on streaming time-series data, we propose an online version of the test capable of detecting outliers under varying patterns. We perform extensive experiments with simulated data, syntactic data, and real online traffic data from Yahoo Webscope, showing a clear advantage of the proposed method, particularly for analyzing streaming data with varying patterns.
更多查看译文
关键词
Anomaly detection,Generalized extreme studentized deviate (GESD) test,Time series,Streaming data
AI 理解论文
溯源树
样例
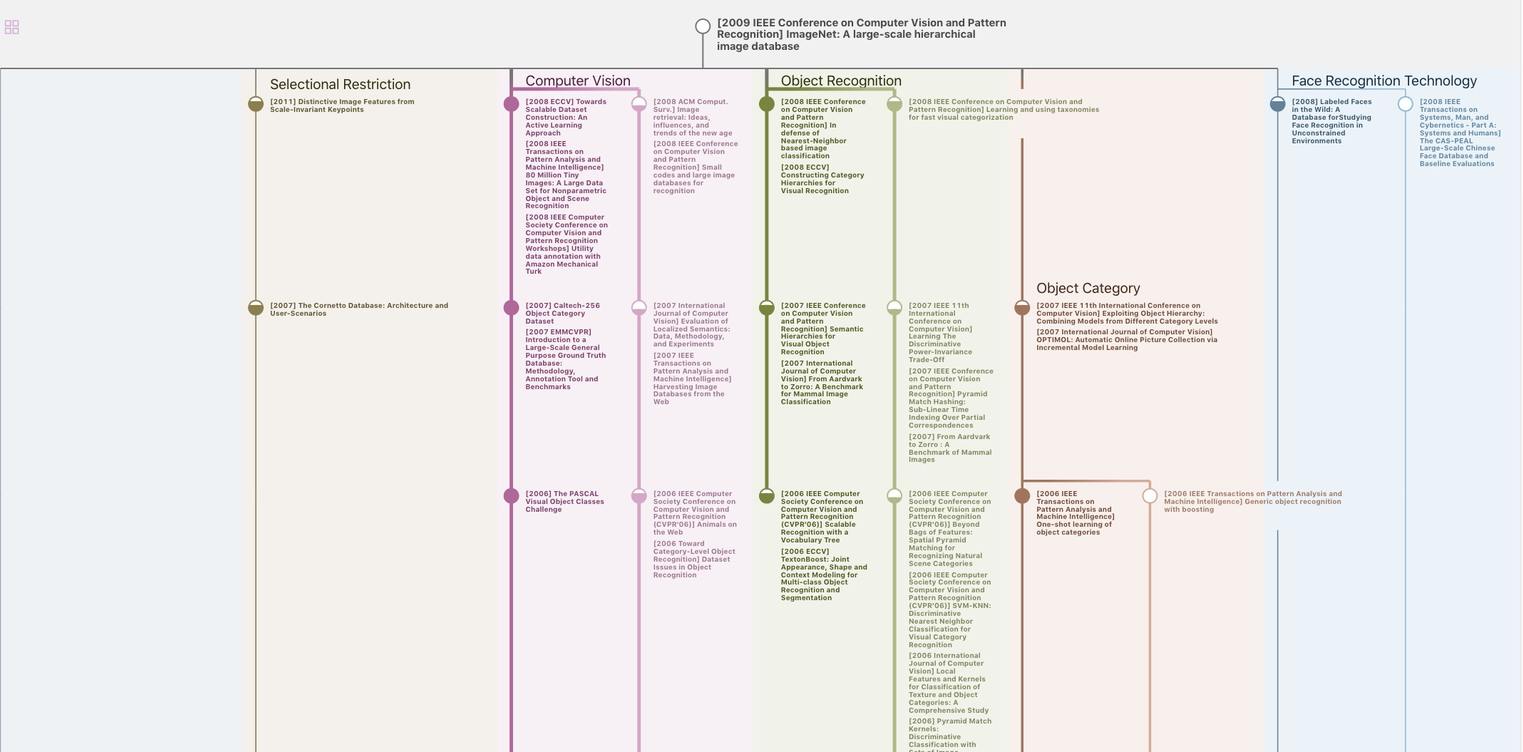
生成溯源树,研究论文发展脉络
Chat Paper
正在生成论文摘要