GAMSNet: Deblurring via Generative Adversarial and Multi-Scale Networks
chinese automation congress(2020)
Abstract
Traditional deblurring algorithms such as blur kernel estimation and gradient prior methods, these methods can only deal with a single type of blurry image, and such methods are very time-consuming. Due to the excellent performance of deep learning neural networks in the field of image generation, this paper regards the traditional image deblurring problem as a special image transformation problem. First, a multi-scale network is used to restore the blurry image, the network can restore the image in an end-to-end manner. Then, the multi-scale network will output a restored image. Finally, the generative adversarial network (GAN) can make the restored image closer to the real clear image in the sense. In terms of loss function, perceptual loss in content loss is adopted to ensure the consistency of the generated image and clear image in content. The results of deblurring will be evaluated by testing the peak signal to noise ratio (PSNR) and structural similarity (SSIM) between the generated image and the clear image. The results show that the PSNR and SSIM of the algorithm in the GoPro dataset have reached 28.01 and 0.8538, respectively, and can recover some details lost due to shake blur.
MoreTranslated text
Key words
shake blur,blind deblurring,generative adversarial network (GAN),multi-scale network,perceptual loss
AI Read Science
Must-Reading Tree
Example
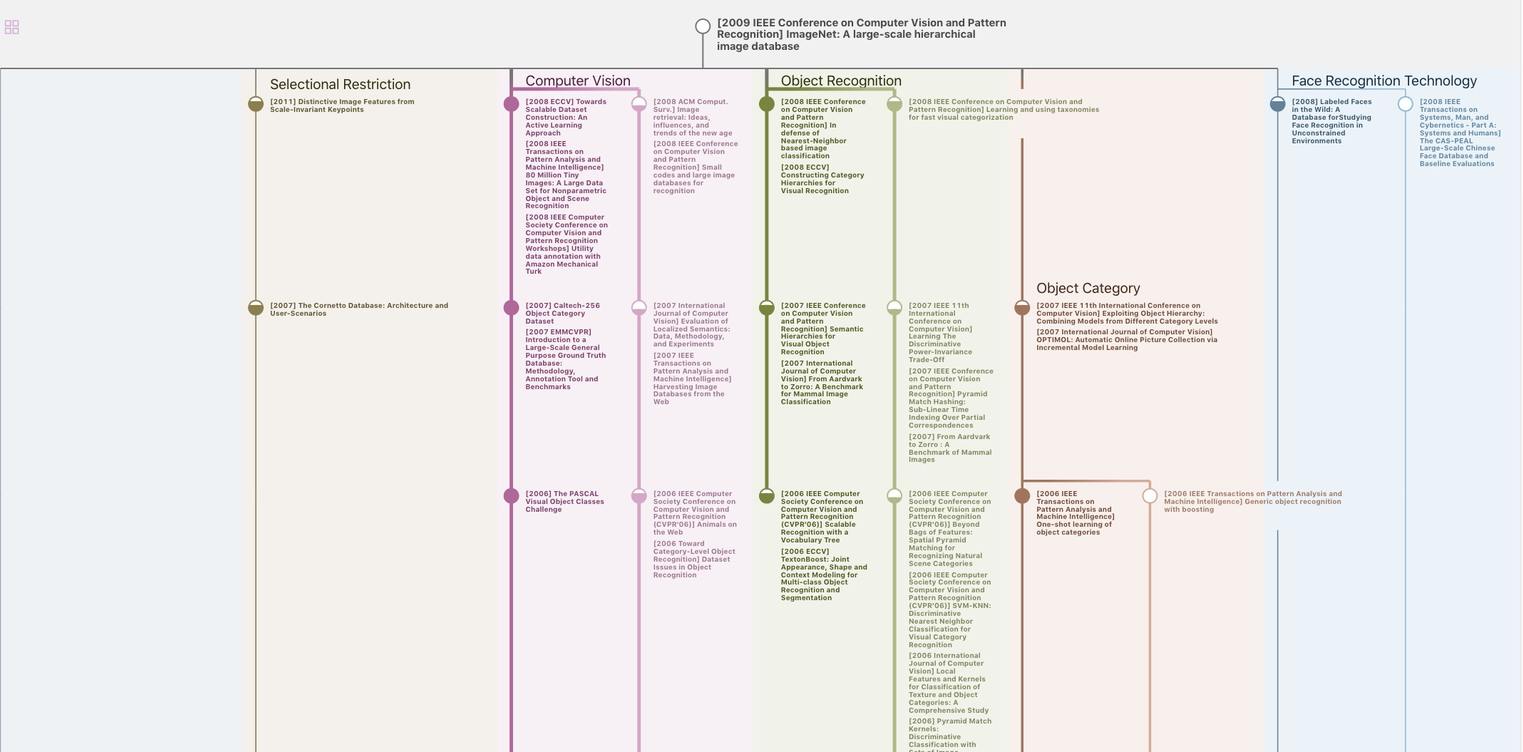
Generate MRT to find the research sequence of this paper
Chat Paper
Summary is being generated by the instructions you defined