Dynamic Joint Distribution Alignment Network For Bearing Fault Diagnosis Under Variable Working Conditions
IEEE TRANSACTIONS ON INSTRUMENTATION AND MEASUREMENT(2021)
摘要
An inconsistent distribution between training and testing data caused by complicated and changeable machine working conditions hinders wide applications of traditional deep learning for machine fault diagnosis. In a target domain, in which labeled samples are not available (testing data), transfer learning can adopt a relevant source domain (training data) to identify the similarity between the two domains and subsequently mitigate the negative effects of a domain shift. Previous studies on transfer learning mainly focused on decreasing the marginal distribution distance of two different domains or narrowing the conditional distribution distance even though marginal and conditional distributions provide different contributions to transfer tasks. The relative importance of the two distributions is difficult to dynamically and quantitatively assess. To align the two distributions (joint distribution) of two different domains, in this article, we propose a dynamic joint distribution alignment network (DJDAN) to evaluate the relative importance of marginal and conditional distributions dynamically and quantitatively. Furthermore, compared with common metrics that use pseudo labels to calculate the conditional distribution distance, the proposed DJDAN uses soft pseudo labels to more accurately measure the conditional distribution discrepancy between different domains. Extensive experiments reveal the superiority and generalization of the proposed DJDAN for bearing fault diagnosis under different working conditions.
更多查看译文
关键词
Bearing, distribution alignment, fault diagnosis, soft pseudo labels, unsupervised learning
AI 理解论文
溯源树
样例
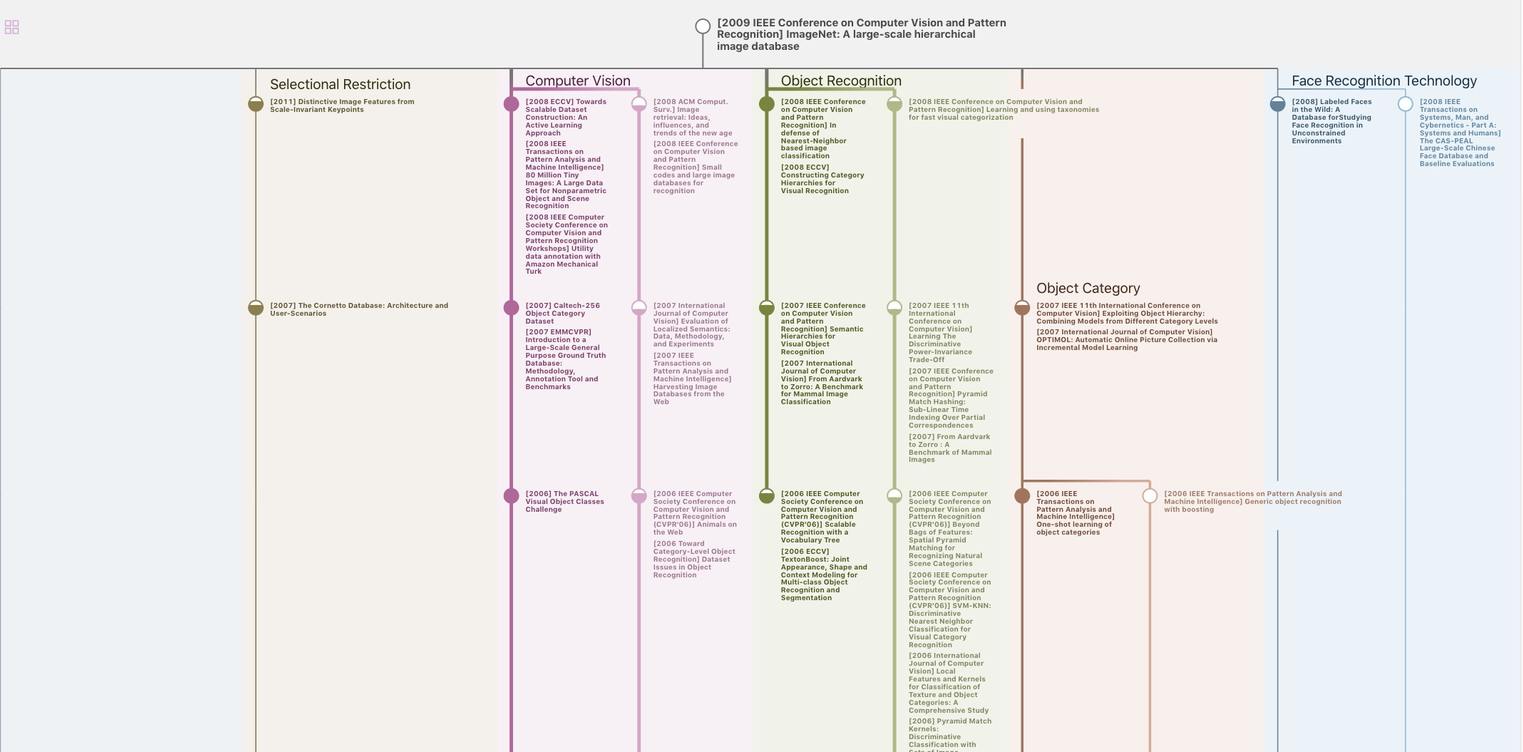
生成溯源树,研究论文发展脉络
Chat Paper
正在生成论文摘要