Multi-Fault Diagnosis Of Interacting Multiple Model Batteries Based On Low Inertia Noise Reduction
IEEE ACCESS(2021)
Abstract
In view of the problems of declined estimation and diagnostic accuracy, as well as diagnosis delay caused by the fixed model transformation probability of the interacting multiple model (IMM) fault diagnosis algorithm, an IMM algorithm based on low inertia noise reduction (LN-IMM) was presented in this paper. The proposed algorithm realized the multi-fault diagnosis of lithium-ion batteries in combination with strong tracking Kalman filter (STKF). In the non-model transformation stage, a transition probability correction function was constructed using the difference of the n-th order based on model probability to suppress the effect of noise on the estimation accuracy of the algorithm. In the model transformation stage, a model jump threshold was introduced in order to reduce inertia when the matched model was switched and realized quick model transformation. Accordingly, the final experiment proved that the LN-IMM-STKF algorithm efficiently completed the state estimation of lithium-ion batteries and improved the diagnostic accuracy of faults while reducing diagnosis delay and attaining accurate detection as well as rapid separation of battery fault information.
MoreTranslated text
Key words
Batteries, Circuit faults, Fault diagnosis, Integrated circuit modeling, Switches, Kalman filters, Filtering algorithms, Interactive multiple model, low inertia, noise reduction, strong tracking Kalman filter, lithium-ion battery
AI Read Science
Must-Reading Tree
Example
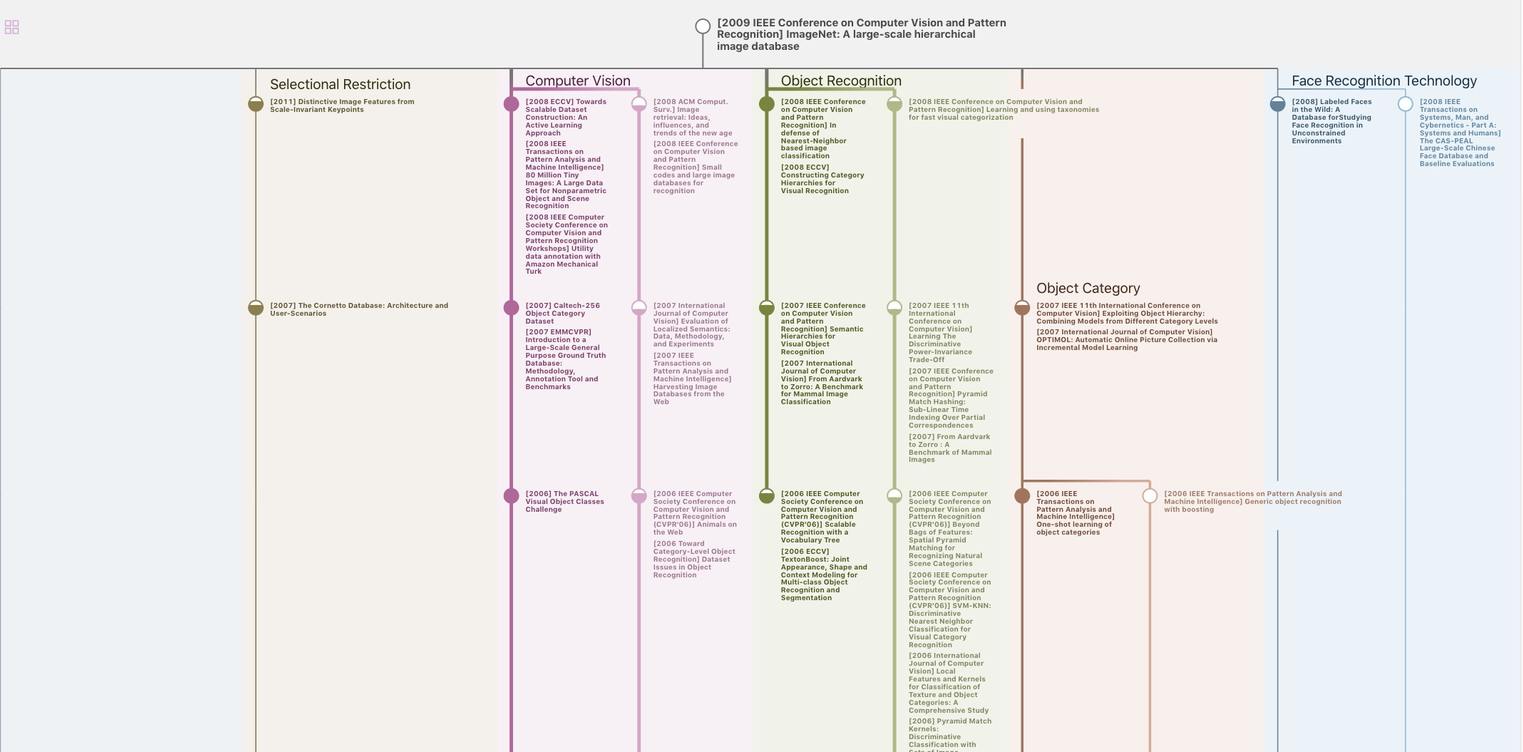
Generate MRT to find the research sequence of this paper
Chat Paper
Summary is being generated by the instructions you defined