Scaling Digital Screen Reading With One-Shot Learning And Re-Identification
2021 IEEE WINTER CONFERENCE ON APPLICATIONS OF COMPUTER VISION WACV 2021(2021)
摘要
Using only a mobile phone app, our objective is to cheaply retro-fit digital meters (e.g blood pressure, blood glucose or industrial gauges) with 'smart' data transfer capabilities. Using the mobile phone camera we build an app to securely and accurately transcribe information from digital meter screens. Only a single labelled training image of a target meter is required to build a custom screen reading module. Here we show how this can scale to potentially hundreds of different meters by learning to recognising the meter type so that the reading module can be automatically selected. This makes the system very easy for a user who would need to scan multiple different meter types. To this end, we build a CNN based system which runs in real-time on mobile device with very high read accuracy and meter recognition. Our contributions include (i) a method of one-shot training by synthesis through domain shift reduction, (ii) a deep embedding network for scale, translation and rotation invariant re-identification of digital meters, (iii) a highly accurate and efficient mobile phone app for recognising and parsing digital meter screens and (iv) release of a new digital meter re-identification dataset.
更多查看译文
关键词
Screen reading,One-shot learning,Computer vision,Scaling,Computer science,Artificial intelligence,Re identification
AI 理解论文
溯源树
样例
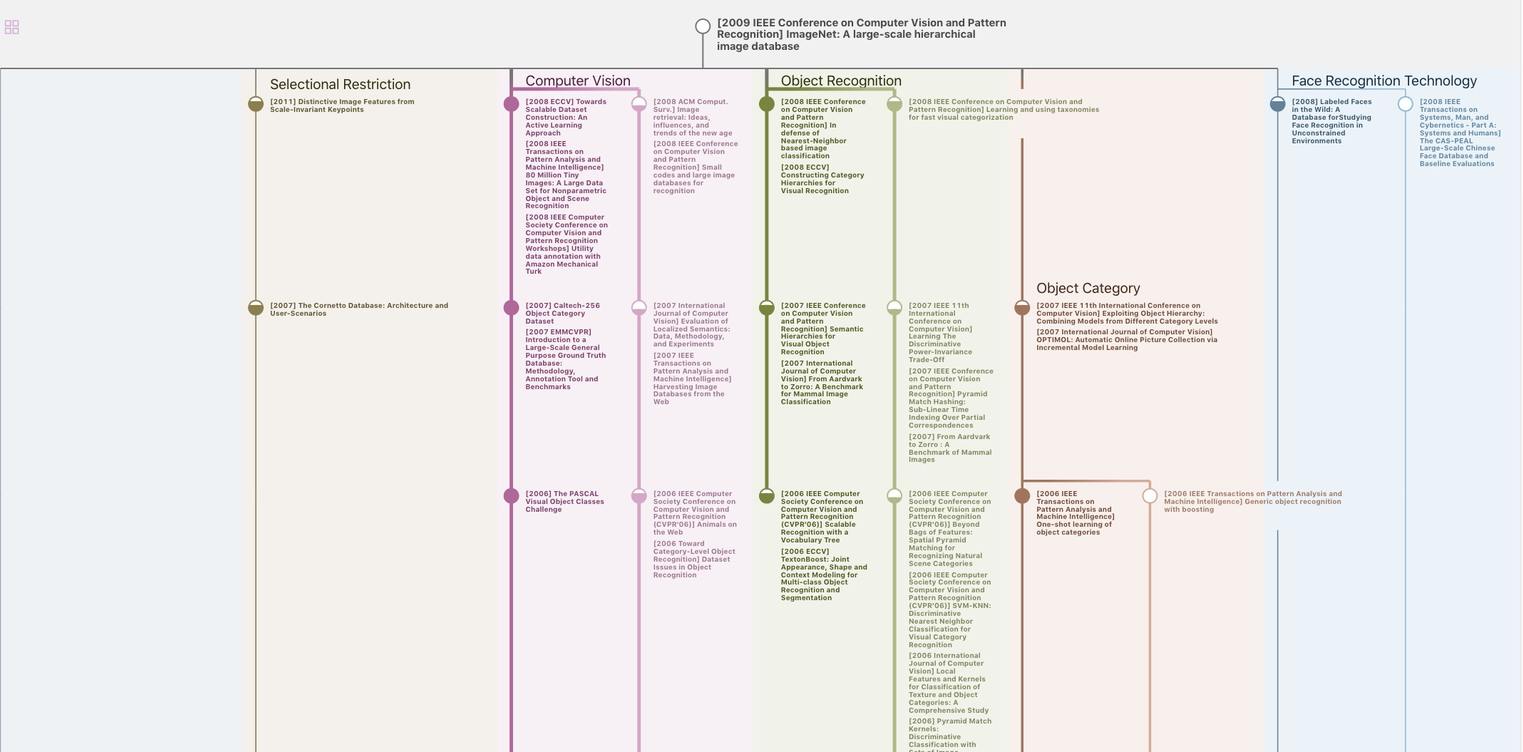
生成溯源树,研究论文发展脉络
Chat Paper
正在生成论文摘要