Fast Tracing of Microtubule Centerlines in Electron Tomograms
user-5fe1a78c4c775e6ec07359f9(2011)
摘要
The organization and role of the cytoskeleton, particularly the network formed by microtubules, is an active topic of research in cell biology. To study the role of these tubular shaped macromolecules, 25nm in diameter, information about their length, number, spatial distribution and dynamics must be gathered. Currently, the only technique that provides the needed resolution is electron microscopy. To gather 3d spatial information, samples are cut in sections of 300nm thickness to allow a projection with an electron beam. The volume is then reconstructed from projections with different tilt angles, a technique called electron tomography. Unfortunately, samples cannot be tilted up to 90◦ in the microscope, which results in an artifact in electron tomograms known as ‘missing wedge’. This artifact as well as angular sampling, imperfections in the sample preparation and destructive effects caused by the electron beam, result in a low signal-to-noise ratio in electron tomograms making their automatic processing particularly challenging. Fig. 1 shows examples of microtubules in electron tomograms. To draw reliable conclusions about the structure of the microtubule network, e.g. during cell division, microtubules need to be identified and their end types classified precisely in a large number of specimens. Manual analysis is often unfeasible: The midsection of a centrosome in the mitotic spindle of C. Elegans, for example, contains between 600 and 1000 microtubules. With current methods, manual segmentation of centerlines in one of these sections takes 10 to 30 hours depending on the image quality. Therefore, biological research on microtubules using electron tomography is currently often based on single observations as opposed to deriving statistics from many samples. On the other hand, automatic extraction and classification on the centerlines remains an open problem and has not yet been solved with the needed quality (see [2]). The major goal for any attempt to solve this problem is to reduce the time spent manually without compromising quality. For large scale analyses at least one order of magnitude of speedup is needed. Segmentation and classification can be divided into two tasks: The tracing of the centerlines and the classification of microtubule ends as being open or closed not a trivial assignment as can be seen in Fig. 1. Since the major bottleneck is the tracing of the centerlines, we describe how the time spent manually on this task can already
更多查看译文
关键词
Electron tomography,Tracing,Segmentation,Image quality,Tomography,Speedup,Microscope,Spatial analysis,Computer vision,Artificial intelligence
AI 理解论文
溯源树
样例
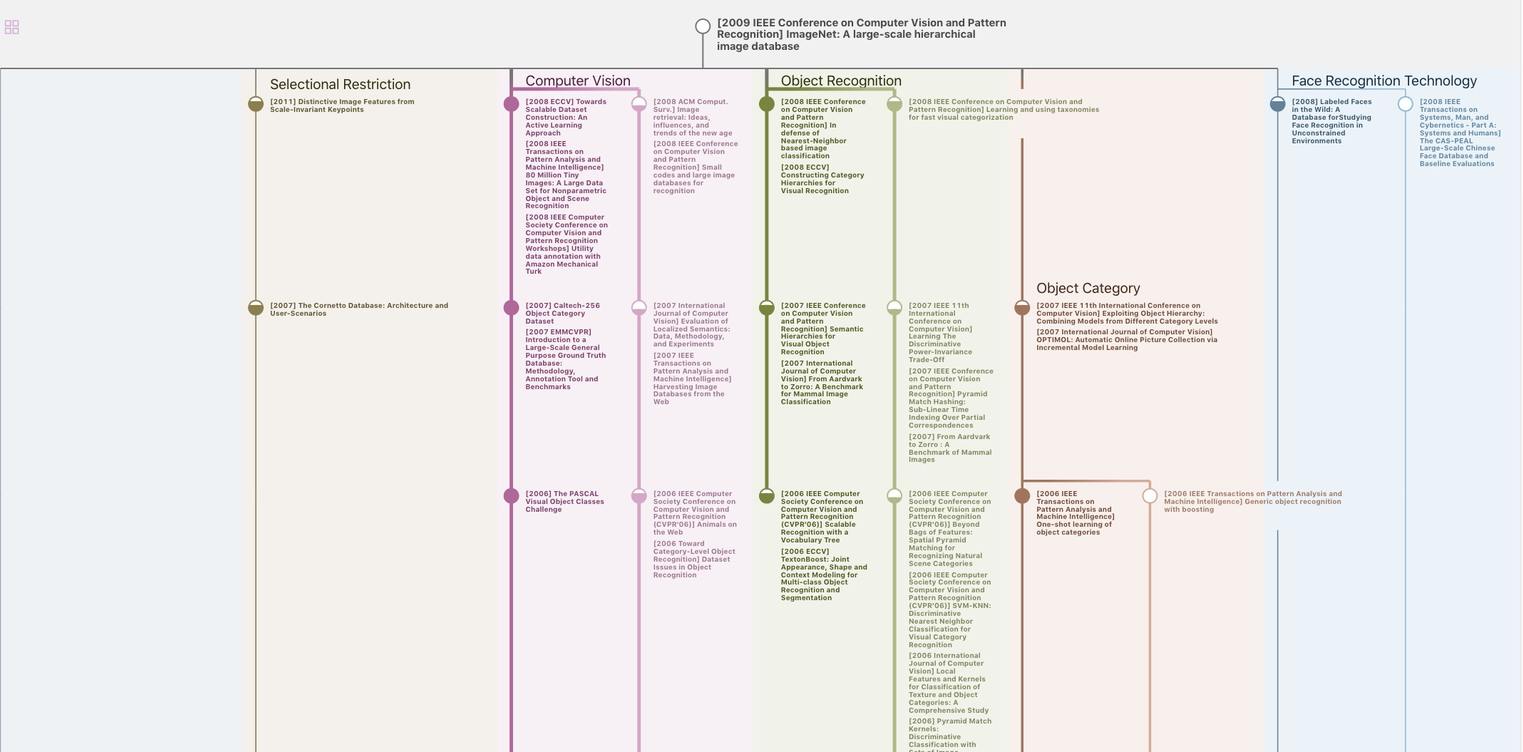
生成溯源树,研究论文发展脉络
Chat Paper
正在生成论文摘要