Testing QA Systems’ ability in Processing Synonym Commonsense Knowledge
2020 24th International Conference Information Visualisation (IV)(2020)
摘要
'Synonym' is an imperative instrument of commonsense knowledge that we apply to make a good sense and sound judgement of our reading. To investigate the ability of machine comprehension models in handling the synonym commonsense knowledge, we developed an innovative approach to automatically generate a dataset based on the Stanford Question Answering Dataset (SQuAD 2.0). The brand-new dataset consists of additional distracting sentences or questions spawned using synonym commonsense knowledge. We formulated new questions by replacing noun entities of the original ones in SQuAD 2.0 with their synonyms. This approach followed the two fundamental principles of SQuAD 2.0 dataset: relevancy and plausibility (incorrect answers are more challenging if they are relevant and plausible). It improves the robustness/abstraction of the question set. To improve the synonym selection strategy in Word Sense Disambiguation (WSD) problem, we designed a new algorithm Multiple Source Adapted Lesk Algorithm (MSALA). Rather than only using WordNet as the source of gloss for adapted Lesk algorithm, we used both lexical database WordNet and commonsense database ConceptNet. This fusion provides a rich hierarchy of semantic relations for the MSALA algorithm. Using this method, we devised 11,000 questions and evaluated the performance of the state-of-the-art question answering system-BERT. Our result shows that the accuracy of the contemporary BERT-Base model dropped from 74.98% to 63.24%. This 10+% accuracy drop revealed the limitations of BERT in handling synonym commonsense knowledge.
更多查看译文
关键词
Commonsense knowledge,QA Systems,Word Sense Disambiguation,Machine Reading Comprehension
AI 理解论文
溯源树
样例
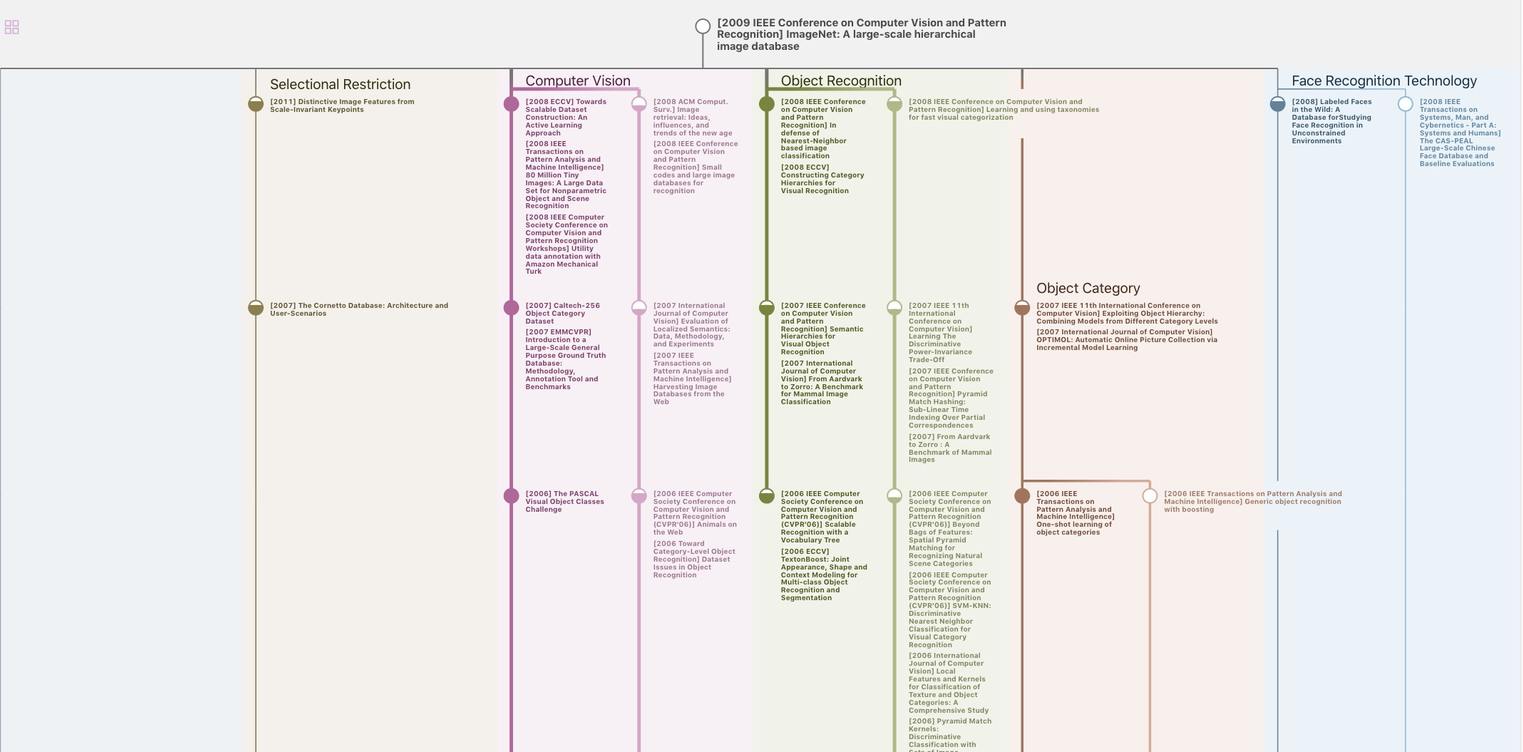
生成溯源树,研究论文发展脉络
Chat Paper
正在生成论文摘要