RiftNet: Radio Frequency Classification for Large Populations
2021 IEEE 18th Annual Consumer Communications & Networking Conference (CCNC)(2021)
Abstract
Radio transmitters can be identified by their unique radio frequency (RF) fingerprints. Hardware differences and manufacturing variations between different RF devices impart the unique RF fingerprints to their transmitted waveforms, which can be exploited to accurately distinguish them. Most previous work has focused on using expert-defined physics-based features to distinguish between 100 or less individual devices, with more recent trends turning to machine learning, and deep learning in particular. In this paper, we present a deep learning approach that scales to 10,000 devices, across different signal types, with upwards of 95% accuracy, which we call RiftNet (Resampling in Frequency and Time Network). RiftNet is particularly suited to pulling out generic signal features that may be relevant to other RF machine learning tasks, beyond just fingerprinting.
MoreTranslated text
Key words
RF,Communications,RF Fingerprint,Deep Learning,Machine Learning,Dilated Causal Convolution
AI Read Science
Must-Reading Tree
Example
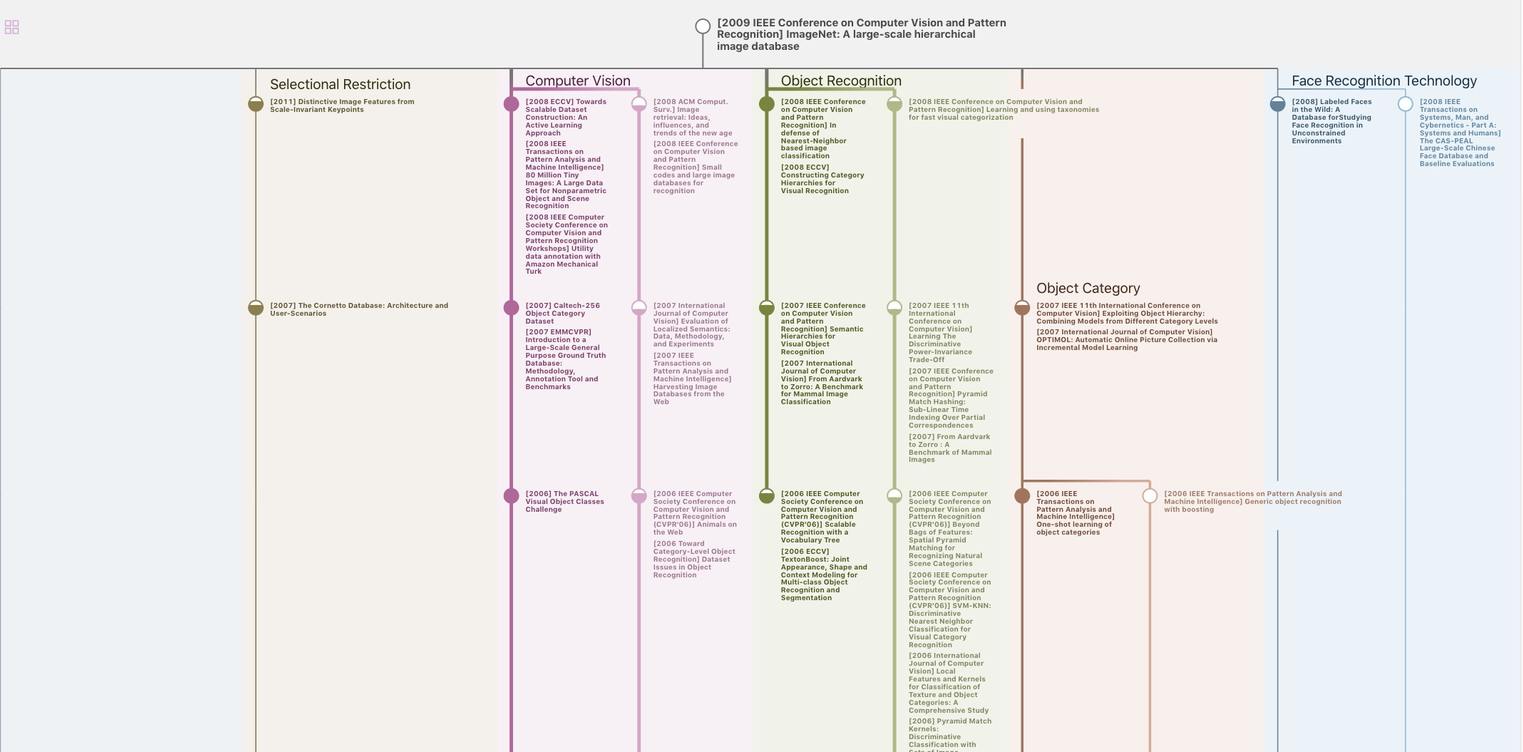
Generate MRT to find the research sequence of this paper
Chat Paper
Summary is being generated by the instructions you defined