Deep Reinforcement Learning-based CIO and Energy Control for LTE Mobility Load Balancing
2021 IEEE 18th Annual Consumer Communications & Networking Conference (CCNC)(2021)
摘要
Cellular networks' congestion has been one of the most common problems in cellular networks due to the huge increase in network load resulted from enhancing communication quality as well as increasing the number of users. Since mobile users are not uniformly distributed in the network, the need for load balancing as a cellular networks' self-optimization technique has increased recently. Then, the congestion problem can be handled by evenly distributing the network load among the network resources. Lots of research has been dedicated to developing load balancing models for cellular networks. Most of these models rely on adjusting the Cell Individual Offset (CIO) parameters which are designed for self-optimization techniques in cellular networks. In this paper, a new deep reinforcement learning-based load balancing approach is proposed as a solution for the LTE Downlink congestion problem. This approach does not rely only on adapting the CIO parameters, but it rather has two degrees of control; the first one is adjusting the CIO parameters, and the second is adjusting the eNodeBs' transmission power. The proposed model uses Double Deep Q-Network (DDQN) to learn how to adjust these parameters so that a better load distribution in the overall network is achieved. Simulation results prove the effectiveness of the proposed approach by improving the network overall throughput by up to 21.4% and 6.5% compared to the base-line scheme and the scheme that only adapts CIOs, respectively.
更多查看译文
关键词
Load Balancing (LB),Reinforcement Learning (RL),Double Deep Q-network (DDQN),Cell Individual Offset (CIO)
AI 理解论文
溯源树
样例
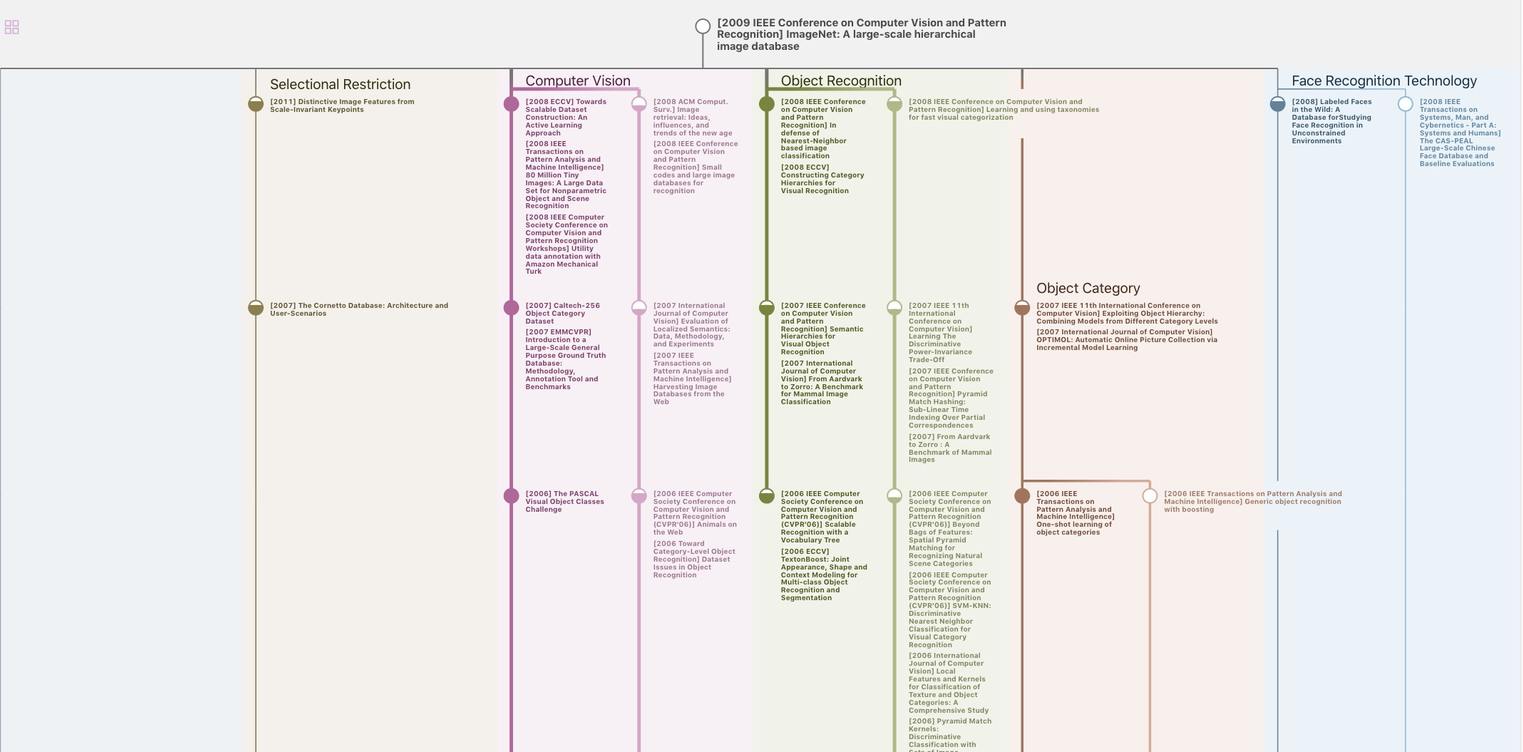
生成溯源树,研究论文发展脉络
Chat Paper
正在生成论文摘要