Accelerating HEP simulations with Neural Importance Sampling
Journal of High Energy Physics(2024)
摘要
Many high-energy-physics (HEP) simulations for the LHC rely on Monte Carlo using importance sampling by means of the VEGAS algorithm. However, complex high-precision calculations have become a challenge for the standard toolbox, as this approach suffers from poor performance in complex cases. As a result, there has been keen interest in HEP for modern machine learning to power adaptive sampling. While previous studies have shown the potential of normalizing-flow-powered neural importance sampling (NIS) over VEGAS, there remains a gap in accessible tools tailored for non-experts. In response, we introduce ZüNIS, a fully automated NIS library designed to bridge this divide, while at the same time providing the infrastructure to customise the algorithm for dealing with challenging tasks. After a general introduction on NIS, we first show how to extend the original formulation of NIS to reuse samples over multiple gradient steps while guaranteeing a stable training, yielding a significant improvement for slow functions. Next, we introduce the structure of the library, which can be used by non-experts with minimal effort and is extensivly documented, which is crucial to become a mature tool for the wider HEP public. We present systematic benchmark results on both toy and physics examples, and stress the benefit of providing different survey strategies, which allows higher performance in challenging cases. We show that ZüNIS shows high performance on a range of problems with limited fine-tuning.
更多查看译文
关键词
Automation,Higher-Order Perturbative Calculations,Scattering Amplitudes
AI 理解论文
溯源树
样例
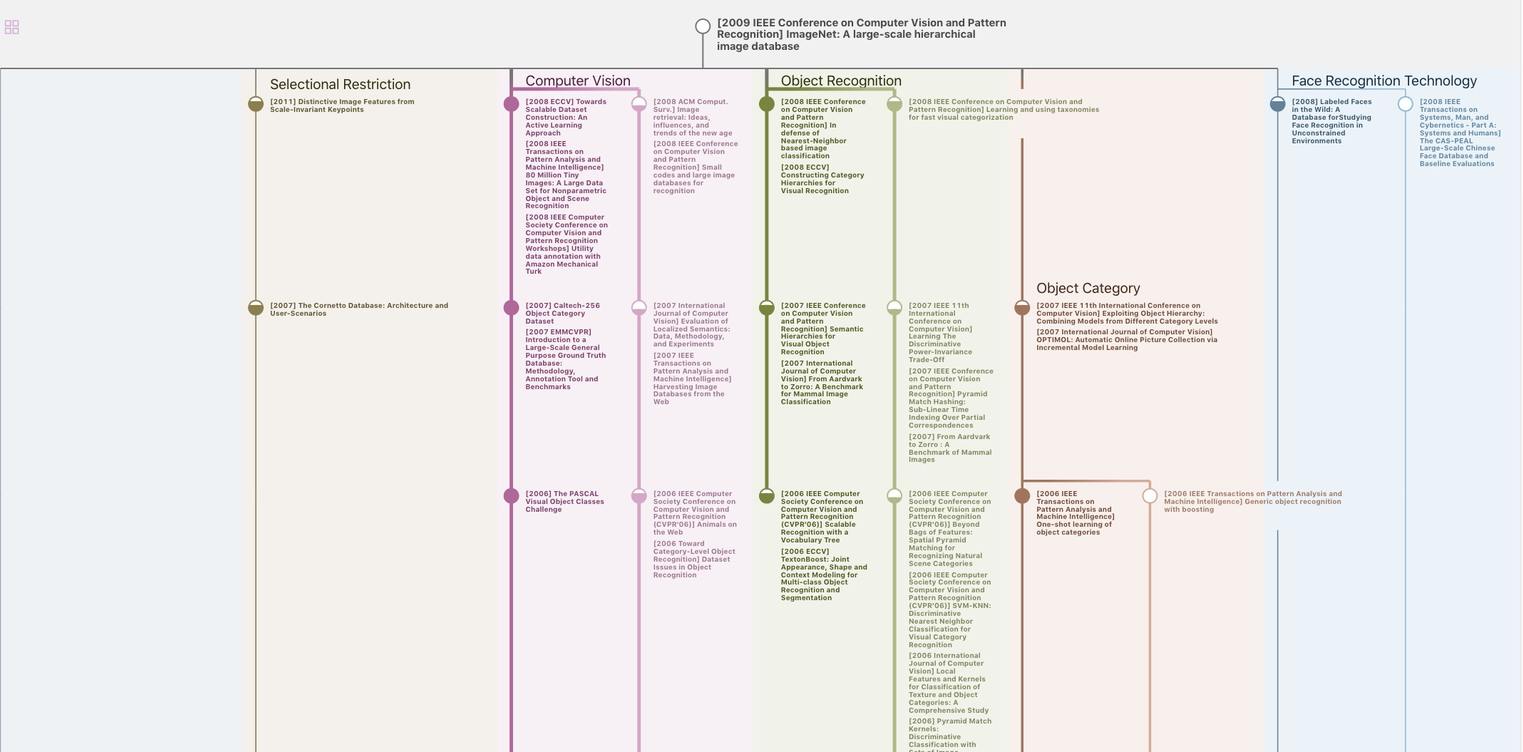
生成溯源树,研究论文发展脉络
Chat Paper
正在生成论文摘要