OmniPose: A Multi-Scale Framework for Multi-Person Pose Estimation
CoRR(2021)
Abstract
We propose OmniPose, a single-pass, end-to-end trainable framework, that achieves state-of-the-art results for multi-person pose estimation. Using a novel waterfall module, the OmniPose architecture leverages multi-scale feature representations that increase the effectiveness of backbone feature extractors, without the need for post-processing. OmniPose incorporates contextual information across scales and joint localization with Gaussian heatmap modulation at the multi-scale feature extractor to estimate human pose with state-of-the-art accuracy. The multi-scale representations, obtained by the improved waterfall module in OmniPose, leverage the efficiency of progressive filtering in the cascade architecture, while maintaining multi-scale fields-of-view comparable to spatial pyramid configurations. Our results on multiple datasets demonstrate that OmniPose, with an improved HRNet backbone and waterfall module, is a robust and efficient architecture for multi-person pose estimation that achieves state-of-the-art results.
MoreTranslated text
Key words
estimation,framework,multi-scale,multi-person
AI Read Science
Must-Reading Tree
Example
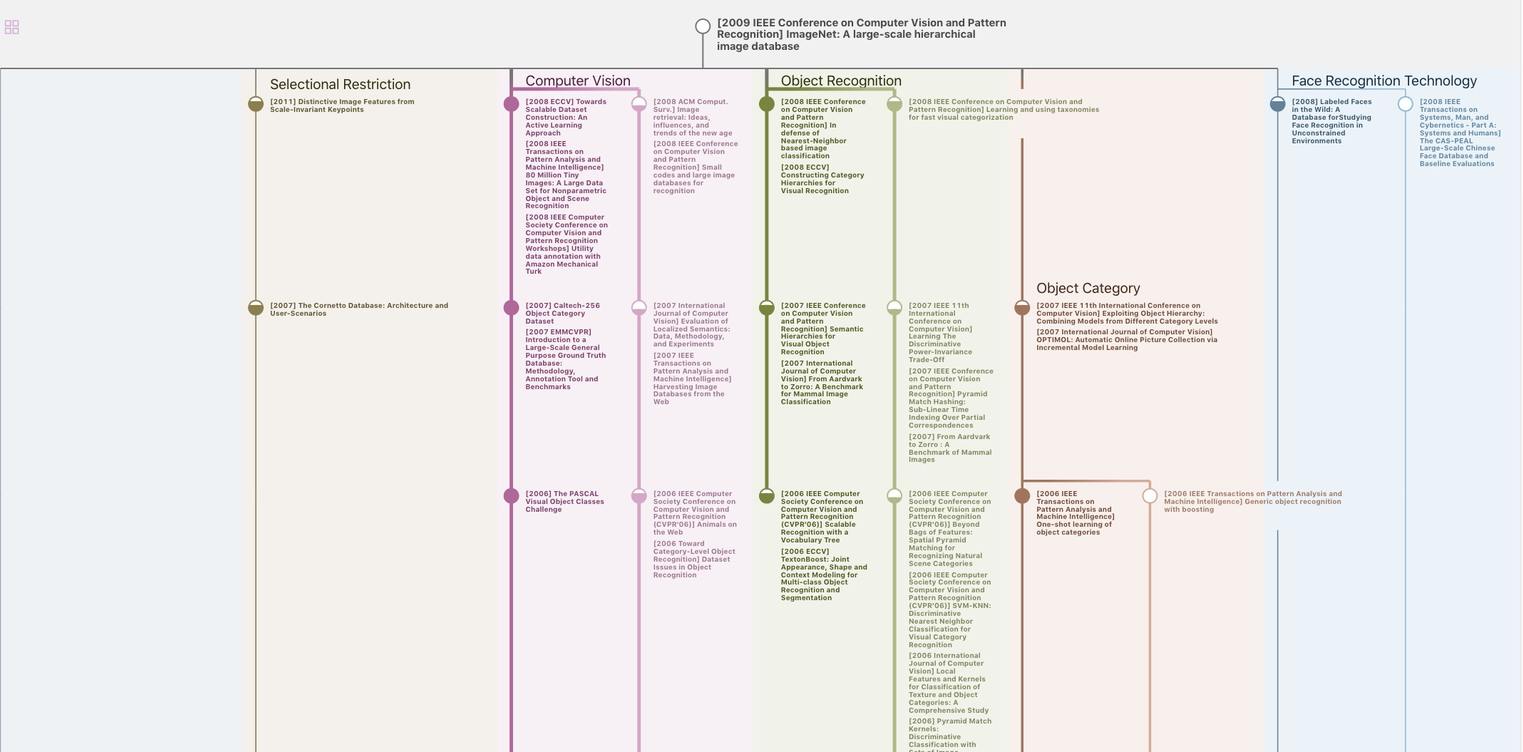
Generate MRT to find the research sequence of this paper
Chat Paper
Summary is being generated by the instructions you defined