An iterative stacked weighted auto-encoder
SOFT COMPUTING(2021)
摘要
The training of stacked auto-encoders (SAEs) consists of an unsupervised layer-wise pre-training and a supervised fine-tuning training. The unsupervised pre-training greedily learns internal data representations, initializes network connection weights and brings high generalization. But in the condition that the saliencies of input data are different, the unsupervised pre-training will preserve unimportant contents, lose useful contents and degrade the performance of SAE. In the light of the problem, an iterative stacked weighted auto-encoder (ISWAE) is proposed. To provide robust and discriminative data representations in the unsupervised pre-training, SAE data weighting is embedded in the SAE network by combining the weighting with data reconstruction in an iterative approach. The SAE weights reflect the global saliencies of input data, which are evaluated through transforming implicit weights to explicit weights based on a trained SAE. The weights gained from the current trained SAE are fed into the next SAE through a weighted data reconstruction function. Further, two iterations can yield satisfactory results and an ISWAE is simplified to a two-iteration stacked weighted auto-encoder. Experiments are carried out on MNIST database, CIFAR-10 database and UCI repository. The results show that ISWAE is superior to state-of-art methods: the classification accuracies of ISWAE are in the first place on the chosen data sets; the iterative SAE weighting can be combined with other SAE variant models, producing higher-performance models; it can effectively resolve the problem of data weighting, feasibly to implement without the need of extra coefficients; its computation complexity is controllable, twice that of SAE.
更多查看译文
关键词
Iterative stacked weighted auto-encoder, Data weight transform, Stacked auto-encoder data weighting, Weighted data reconstruction
AI 理解论文
溯源树
样例
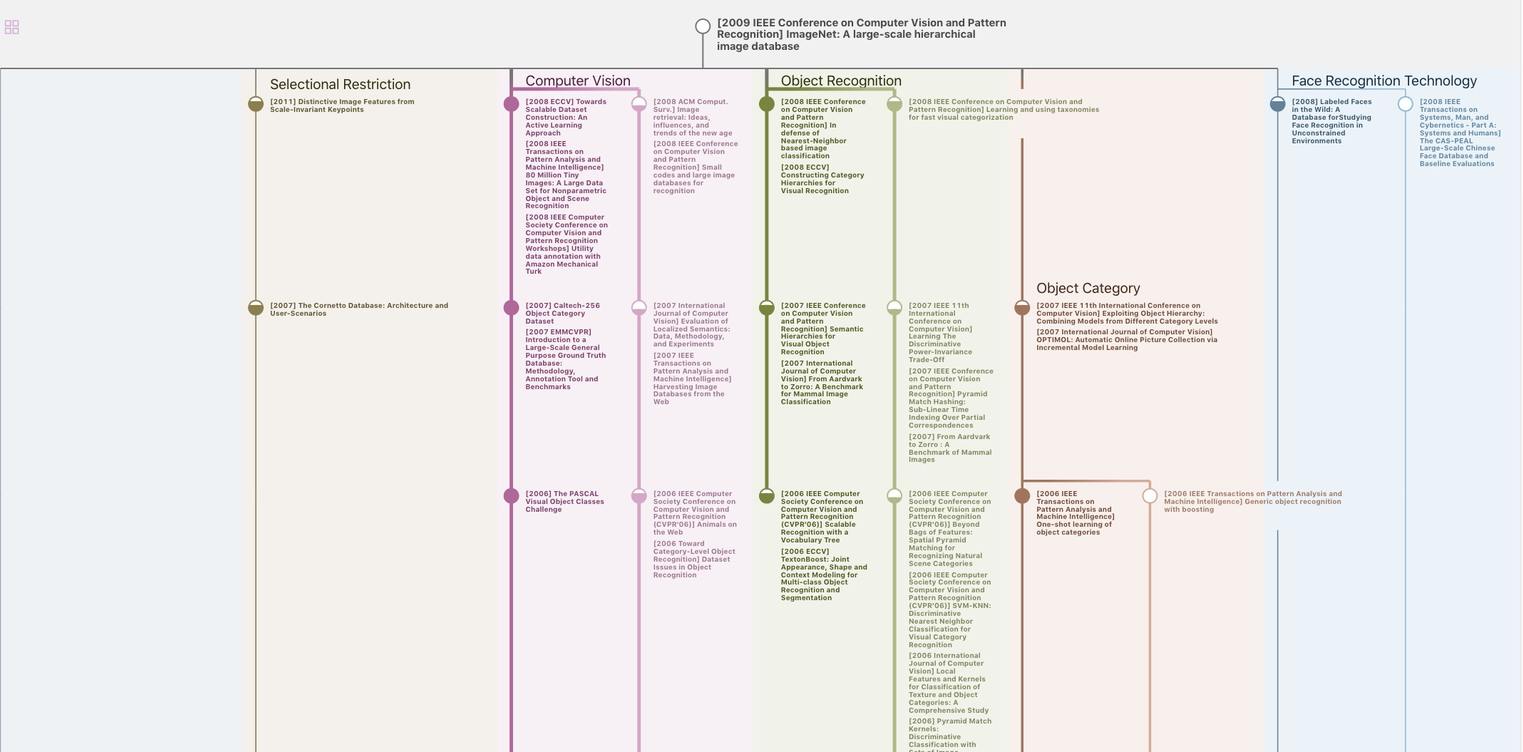
生成溯源树,研究论文发展脉络
Chat Paper
正在生成论文摘要