Self-Supervised And Supervised Joint Training For Resource-Rich Machine Translation
INTERNATIONAL CONFERENCE ON MACHINE LEARNING, VOL 139(2021)
摘要
Self-supervised pre-training of text representations has been successfully applied to low-resource Neural Machine Translation (NMT). However, it usually fails to achieve notable gains on resource-rich NMT. In this paper, we propose a joint training approach, F-2-XEnDec, to combine self-supervised and supervised learning to optimize NMT models. To exploit complementary self-supervised signals for supervised learning, NMT models are trained on examples that are interbred from monolingual and parallel sentences through a new process called crossover encoder-decoder. Experiments on two resource-rich translation benchmarks, WMT' 14 English-German and WMT' 14 English-French, demonstrate that our approach achieves substantial improvements over several strong baseline methods and obtains a new state of the art of 46.19 BLEU on English-French when incorporating back translation. Results also show that our approach is capable of improving model robustness to input perturbations such as code-switching noise which frequently appears on social media.
更多查看译文
关键词
Supervised learning,Machine translation,Transformer (machine learning model),Robustness (computer science),Crossover,Machine learning,Computer science,Exploit,Artificial intelligence,Self supervised learning
AI 理解论文
溯源树
样例
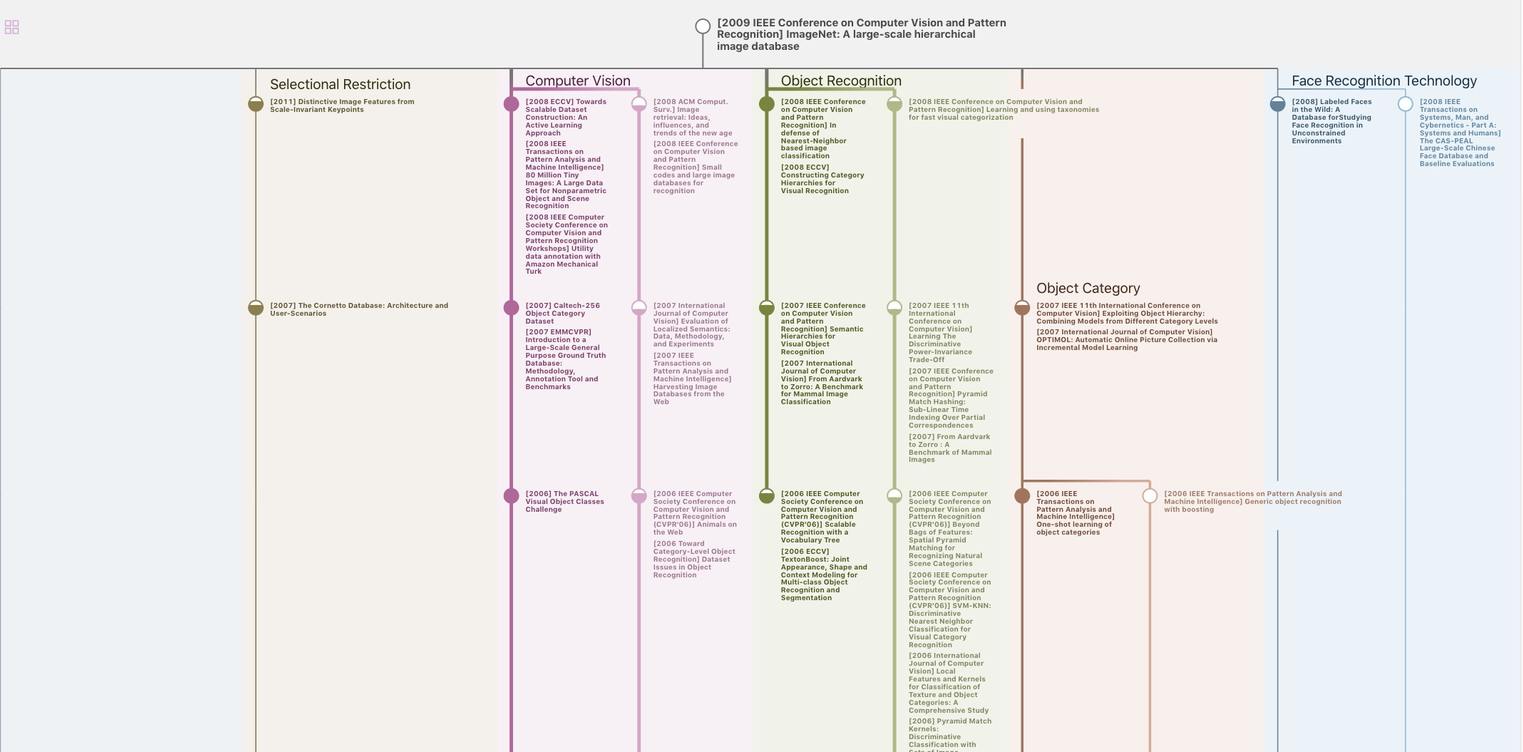
生成溯源树,研究论文发展脉络
Chat Paper
正在生成论文摘要