Machine Learning Methods for the Prediction of Micromagnetic Magnetization Dynamics
IEEE TRANSACTIONS ON MAGNETICS(2022)
摘要
Machine learning (ML) entered the field of computational micromagnetics only recently. The main objective of these new approaches is the automatization of solutions of parameter-dependent problems in micromagnetism, such as fast response curve estimation modeled by the Landau-Lifschitz-Gilbert (LLG) equation. Data-driven models for the solution of time- and parameter-dependent partial differential equations require high-dimensional training data structures. ML, in this case, is by no means a straightforward trivial task; it needs algorithmic and mathematical innovation. Our work introduces theoretical and computational conceptions of the certain kernel and neural network (NN)-based dimensionality reduction approaches for efficient prediction of solutions via the notion of low-dimensional feature space integration. We introduce efficient treatment of kernel ridge regression and kernel principal component analysis via low-rank approximation. The second line follows NN autoencoders as a nonlinear data-dependent dimensional reduction for the training data with a focus on accurate latent space variable description suitable for a feature space integration scheme. We verify and compare numerically by means of a NIST standard problem. The low-rank kernel method approach is fast and surprisingly accurate, while the NN scheme can even exceed this level of accuracy at the expense of significantly higher costs.
更多查看译文
关键词
Kernel, Magnetization, Training, Mathematical model, Principal component analysis, Magnetic domains, Computational modeling, Computational micromagnetism, deep neural networks (NNs), kernel methods, low-rank approximation, nonlinear model order and dimensionality reduction, regularized autoencoders (AEs)
AI 理解论文
溯源树
样例
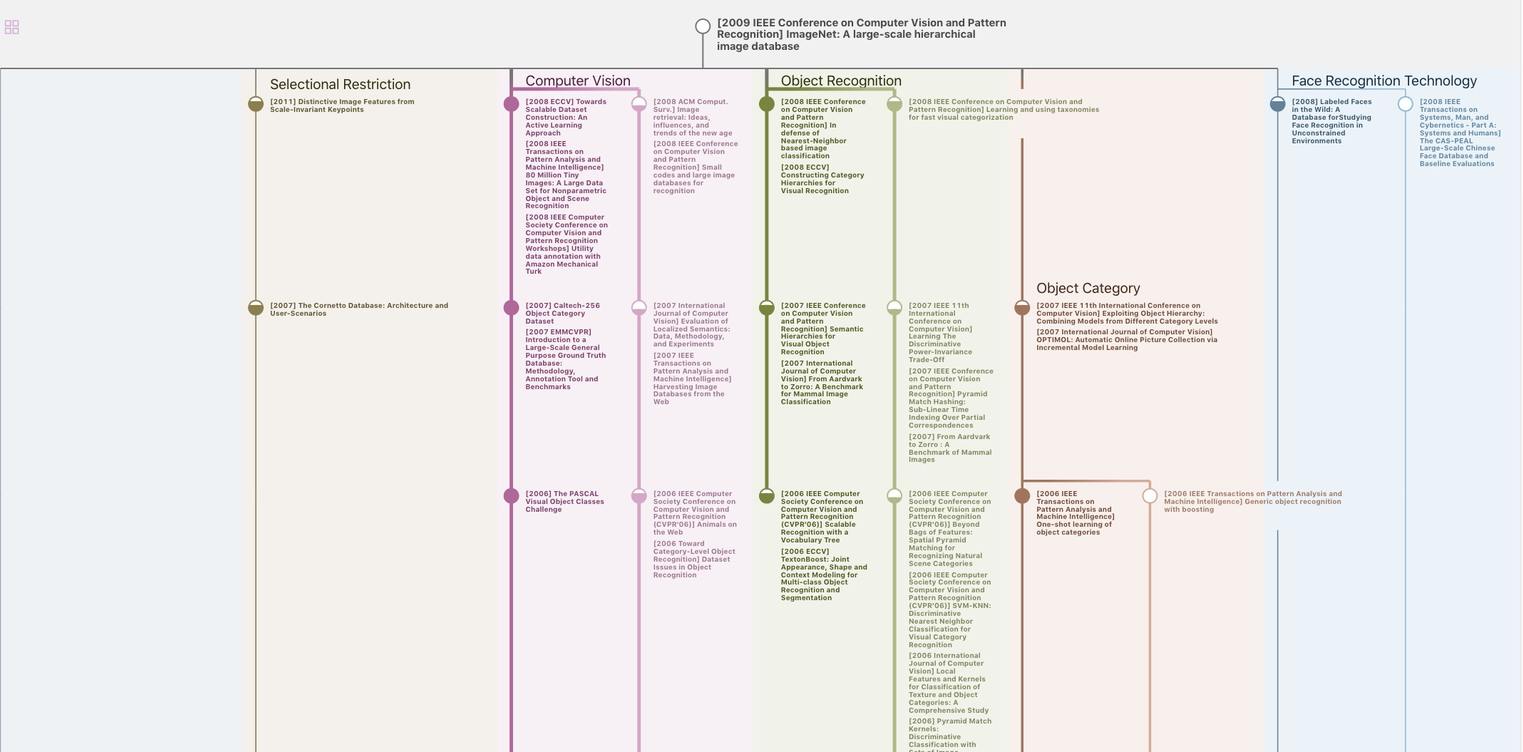
生成溯源树,研究论文发展脉络
Chat Paper
正在生成论文摘要