Deep Reinforcement Learning with Causality-based Intrinsic Reward
user-5f8cf7e04c775ec6fa691c92(2021)
摘要
Reinforcement Learning (RL) has shown great potential to deal with sequential decision-making problems. However, most RL algorithms do not explicitly consider the relations between entities in the environment. This makes the policy learning suffer from the problems of efficiency, effectivity and interpretability. In this paper, we propose a novel deep reinforcement learning algorithm, which firstly learns the causal structure of the environment and then leverages the learned causal information to assist policy learning. The proposed algorithm learns a graph to encode the environmental structure by calculating Average Causal Effect (ACE) between different categories of entities, and an intrinsic reward is given to encourage the agent to interact more with entities belonging to top-ranked categories, which significantly boosts policy learning. Several experiments are conducted on a number of simulation environments to demonstrate the effectiveness and better interpretability of our proposed method.
更多查看译文
关键词
Reinforcement learning,Causal structure,Interpretability,Causality,Artificial intelligence,Computer science,ENCODE,Instrumental and intrinsic value,Causal effect,Causal information
AI 理解论文
溯源树
样例
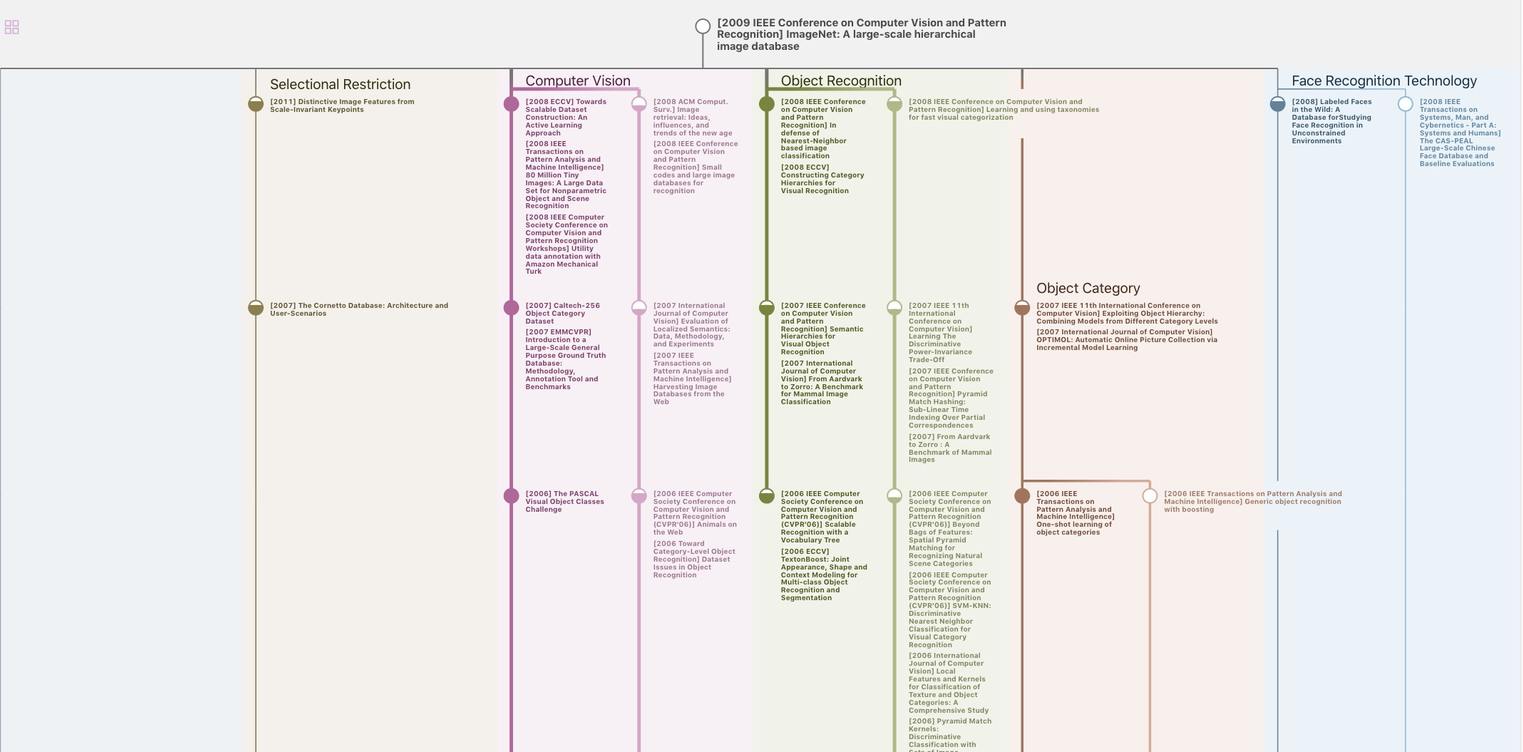
生成溯源树,研究论文发展脉络
Chat Paper
正在生成论文摘要