A Text GAN for Language Generation with Non-Autoregressive Generator
user-5f8cf7e04c775ec6fa691c92(2021)
摘要
Despite the great success of Generative Adversarial Networks (GANs) in generating high-quality images, GANs for text generation still face two major challenges: first, most text GANs are unstable in training mainly due to ineffective optimization of the generator, and they heavily rely on maximum likelihood pretraining; second, most text GANs adopt autoregressive generators without latent variables, which largely limits the ability to learn latent representations for natural language text. In this paper, we propose a novel text GAN, named NAGAN, which incorporates a non-autoregressive generator with latent variables. The non-autoregressive generator can be effectively trained with gradient-based methods and free of pretraining. The latent variables facilitate representation learning for text generation applications. Experiments show that our model is competitive comparing with existing text GANs in unconditional text generation, and it outperforms existing methods on sentence manipulation in latent space and unsupervised text decipherment.
更多查看译文
关键词
Latent variable,Feature learning,Natural language,Autoregressive model,Sentence,Decipherment,Natural language processing,Generative grammar,Computer science,Artificial intelligence,Maximum likelihood
AI 理解论文
溯源树
样例
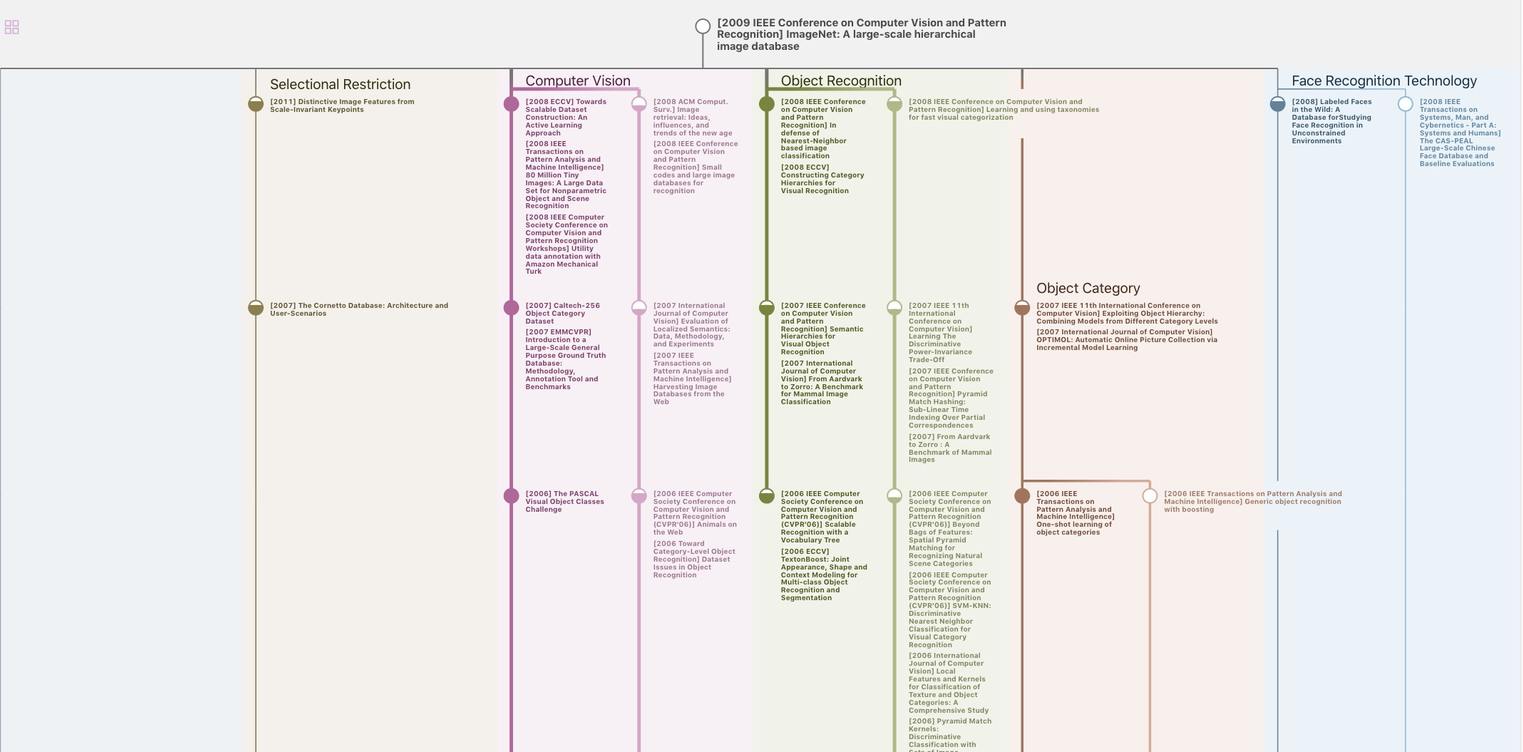
生成溯源树,研究论文发展脉络
Chat Paper
正在生成论文摘要