Sample-efficient Reinforcement Learning Representation Learning with Curiosity Contrastive Forward Dynamics Model
2021 IEEE/RSJ INTERNATIONAL CONFERENCE ON INTELLIGENT ROBOTS AND SYSTEMS (IROS)(2021)
摘要
Developing an agent in reinforcement learning (RL) that is capable of performing complex control tasks directly from high-dimensional observation such as raw pixels is a challenge as efforts still need to be made towards improving sample efficiency and generalization of RL algorithm. This paper considers a learning framework for a Curiosity Contrastive Forward Dynamics Model (CCFDM) to achieve a more sample-efficient RL based directly on raw pixels. CCFDM incorporates a forward dynamics model (FDM) and performs contrastive learning to train its deep convolutional neural network-based image encoder (IE) to extract conducive spatial and temporal information to achieve a more sample efficiency for RL. In addition, during training, CCFDM provides intrinsic rewards, produced based on FDM prediction error, and encourages the curiosity of the RL agent to improve exploration. The diverge and less-repetitive observations provided by both our exploration strategy and data augmentation available in contrastive learning improve not only the sample efficiency but also the generalization. Performance of existing model-free RL methods such as Soft Actor-Critic built on top of CCFDM outperforms prior state-of-the-art pixel-based RL methods on the DeepMind Control Suite benchmark.
更多查看译文
关键词
forward,learning,representation,dynamics,sample-efficient
AI 理解论文
溯源树
样例
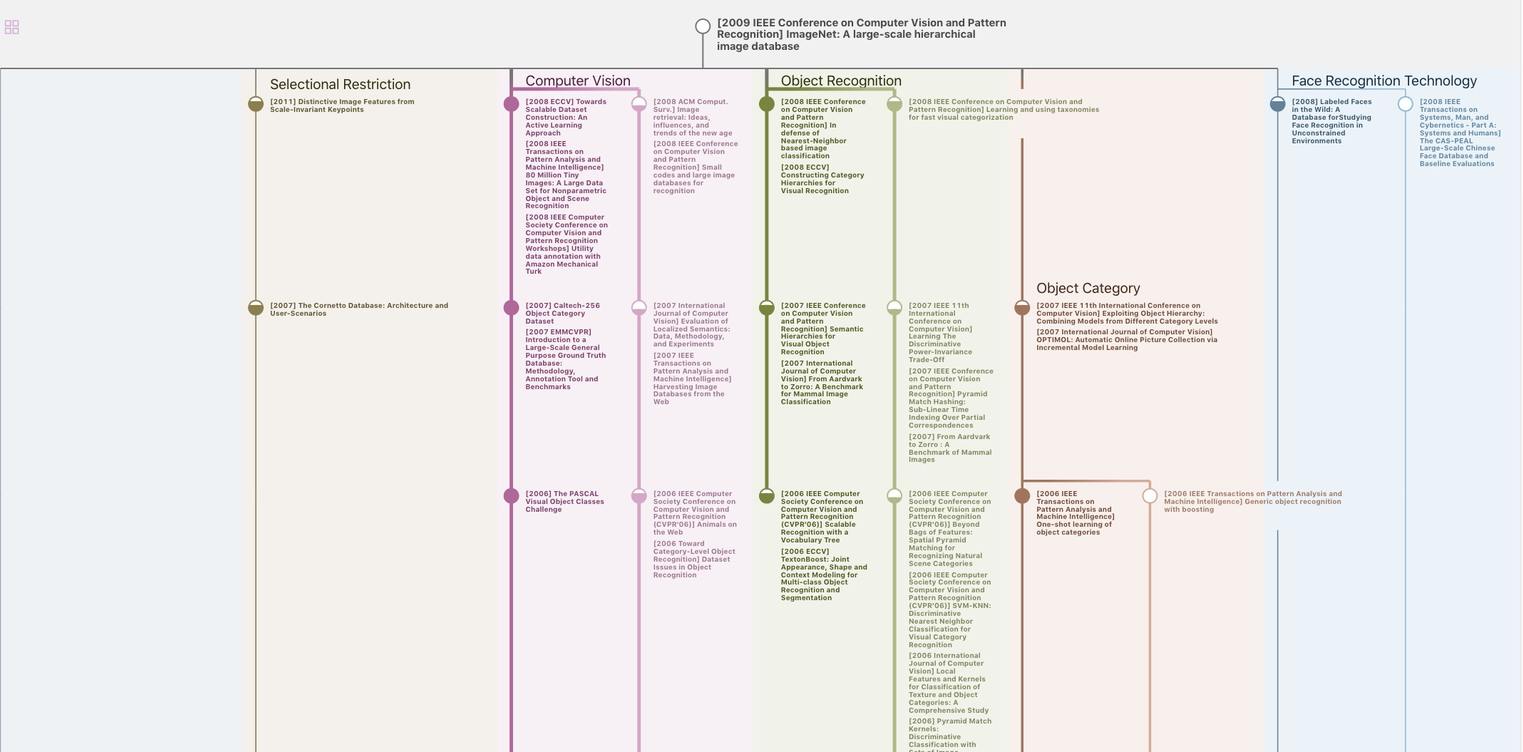
生成溯源树,研究论文发展脉络
Chat Paper
正在生成论文摘要