Generating Synthetic Handwritten Historical Documents with OCR Constrained GANs
DOCUMENT ANALYSIS AND RECOGNITION, ICDAR 2021, PT III(2021)
摘要
We present a framework to generate synthetic historical documents with precise ground truth using nothing more than a collection of unlabeled historical images. Obtaining large labeled datasets is often the limiting factor to effectively use supervised deep learning methods for Document Image Analysis (DIA). Prior approaches towards synthetic data generation either require human expertise or result in poor accuracy in the synthetic documents. To achieve high precision transformations without requiring expertise, we tackle the problem in two steps. First, we create template documents with user-specified content and structure. Second, we transfer the style of a collection of unlabeled historical images to these template documents while preserving their text and layout. We evaluate the use of our synthetic historical documents in a pre-training setting and find that we outperform the baselines (randomly initialized and pre-trained). Additionally, with visual examples, we demonstrate a high-quality synthesis that makes it possible to generate large labeled historical document datasets with precise ground truth.
更多查看译文
关键词
OCR, CycleGAN, Synthetic data, Historical documents
AI 理解论文
溯源树
样例
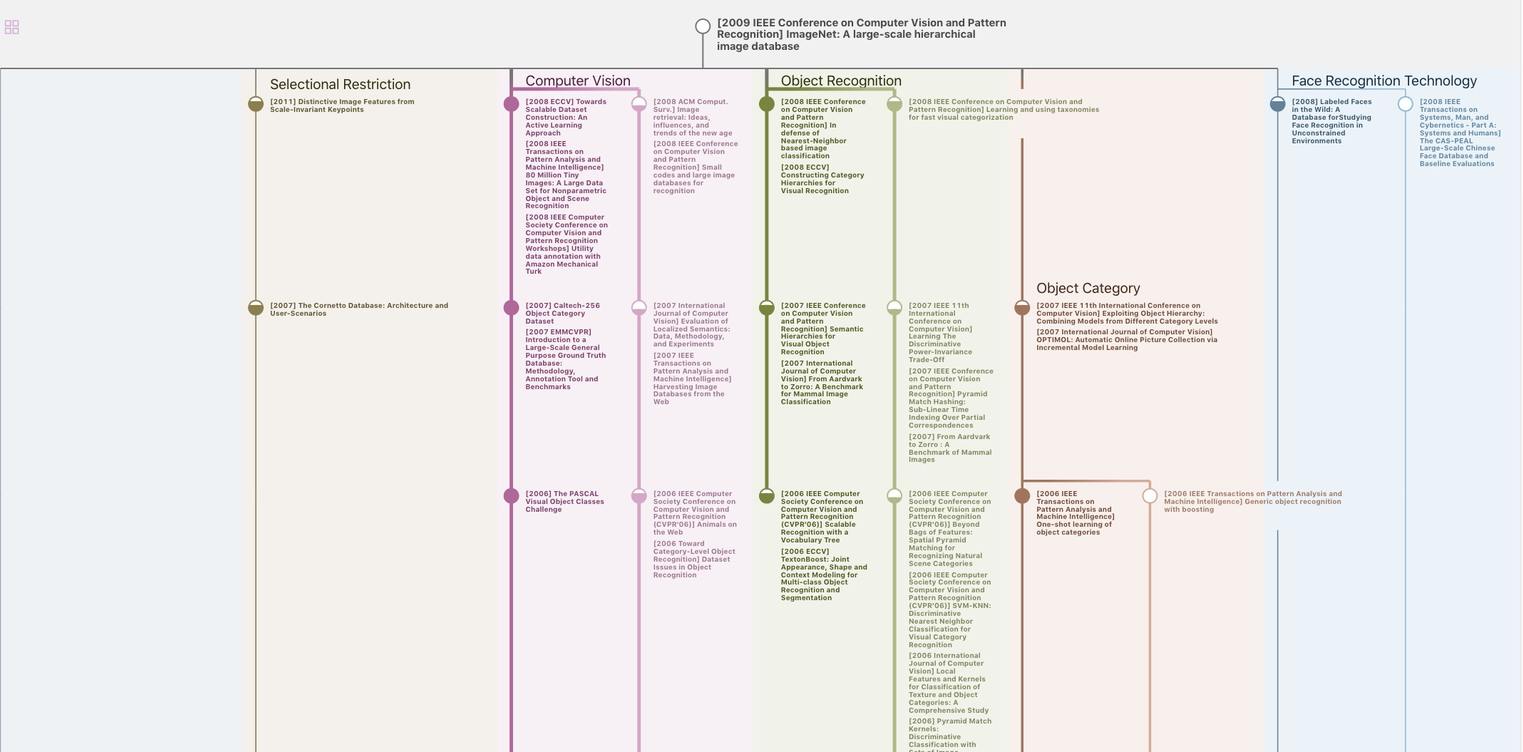
生成溯源树,研究论文发展脉络
Chat Paper
正在生成论文摘要