Efficient Sparse Artificial Neural Networks
arxiv(2021)
摘要
The brain, as the source of inspiration for Artificial Neural Networks (ANN), is based on a sparse structure. This sparse structure helps the brain to consume less energy, learn easier and generalize patterns better than any other ANN. In this paper, two evolutionary methods for adopting sparsity to ANNs are proposed. In the proposed methods, the sparse structure of a network as well as the values of its parameters are trained and updated during the learning process. The simulation results show that these two methods have better accuracy and faster convergence while they need fewer training samples compared to their sparse and non-sparse counterparts. Furthermore, the proposed methods significantly improve the generalization power and reduce the number of parameters. For example, the sparsification of the ResNet47 network by exploiting our proposed methods for the image classification of ImageNet dataset uses 40 % fewer parameters while the top-1 accuracy of the model improves by 12% and 5% compared to the dense network and their sparse counterpart, respectively. As another example, the proposed methods for the CIFAR10 dataset converge to their final structure 7 times faster than its sparse counterpart, while the final accuracy increases by 6%.
更多查看译文
关键词
artificial neural networks,neural networks
AI 理解论文
溯源树
样例
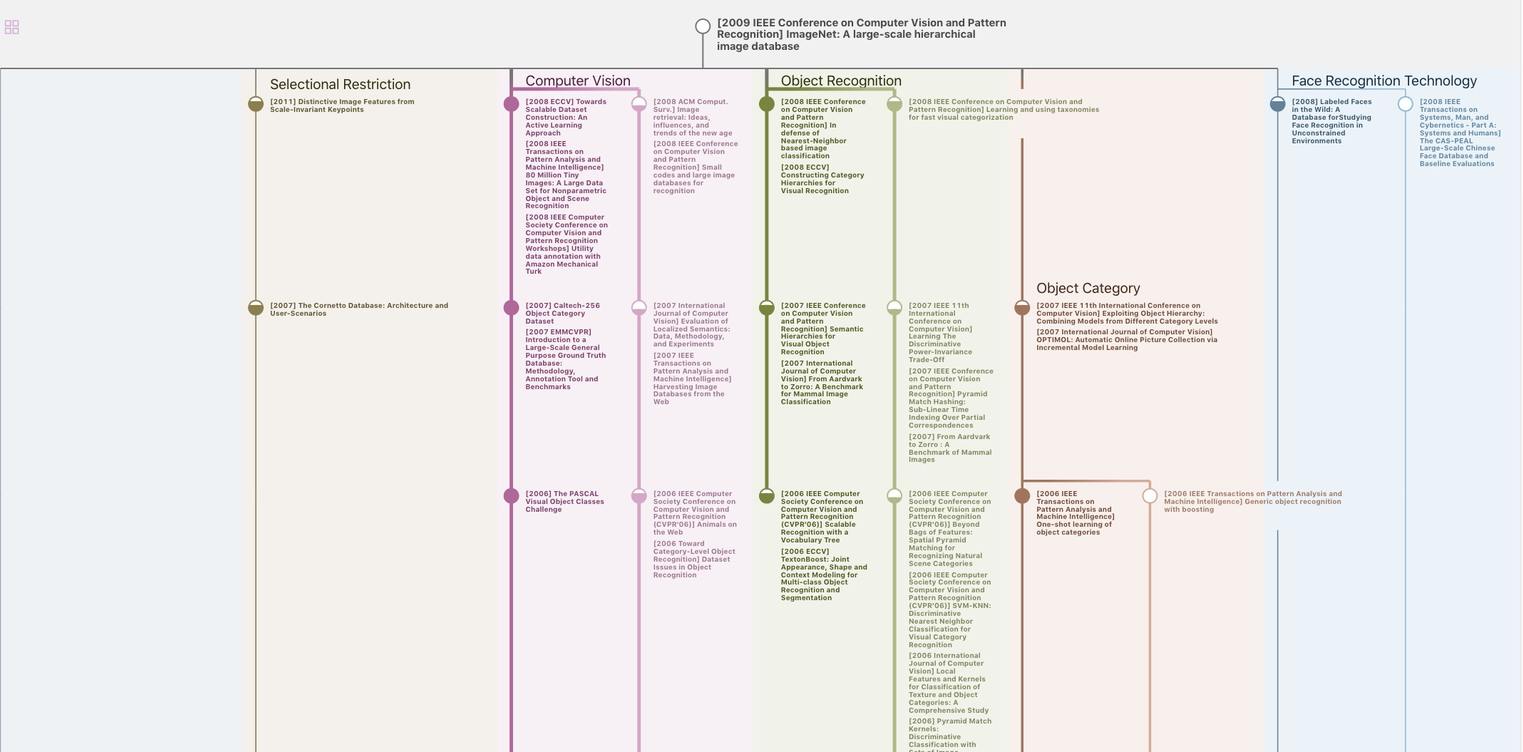
生成溯源树,研究论文发展脉络
Chat Paper
正在生成论文摘要