Projection-Based Qlp Algorithm For Efficiently Computing Low-Rank Approximation Of Matrices
IEEE TRANSACTIONS ON SIGNAL PROCESSING(2021)
Abstract
Matrices with low numerical rank are omnipresent in many signal processing and data analysis applications. The pivoted QLP (p-QLP) algorithm constructs a highly accurate approximation to an input low-rank matrix. However, it is computationally prohibitive for large matrices. In this paper, we introduce a new algorithm termed Projection-based Partial QLP (PbP-QLP) that efficiently approximates the p-QLP with high accuracy. Fundamental in our work is the exploitation of randomization and in contrast to the p-QLP, PbP-QLP does not use the pivoting strategy. As such, PbP-QLP can harness modern computer architectures, even better than competing randomized algorithms. The efficiency and effectiveness of our proposed PbP-QLP algorithm are investigated through various classes of synthetic and real-world data matrices.
MoreTranslated text
Key words
Matrix decomposition, Approximation algorithms, Signal processing algorithms, Sparse matrices, Singular value decomposition, Signal processing, Oceanography, Low-rank approximation, rank-revealing matrix factorization, the pivoted QLP, the singular value decomposition, randomized numerical linear algebra
AI Read Science
Must-Reading Tree
Example
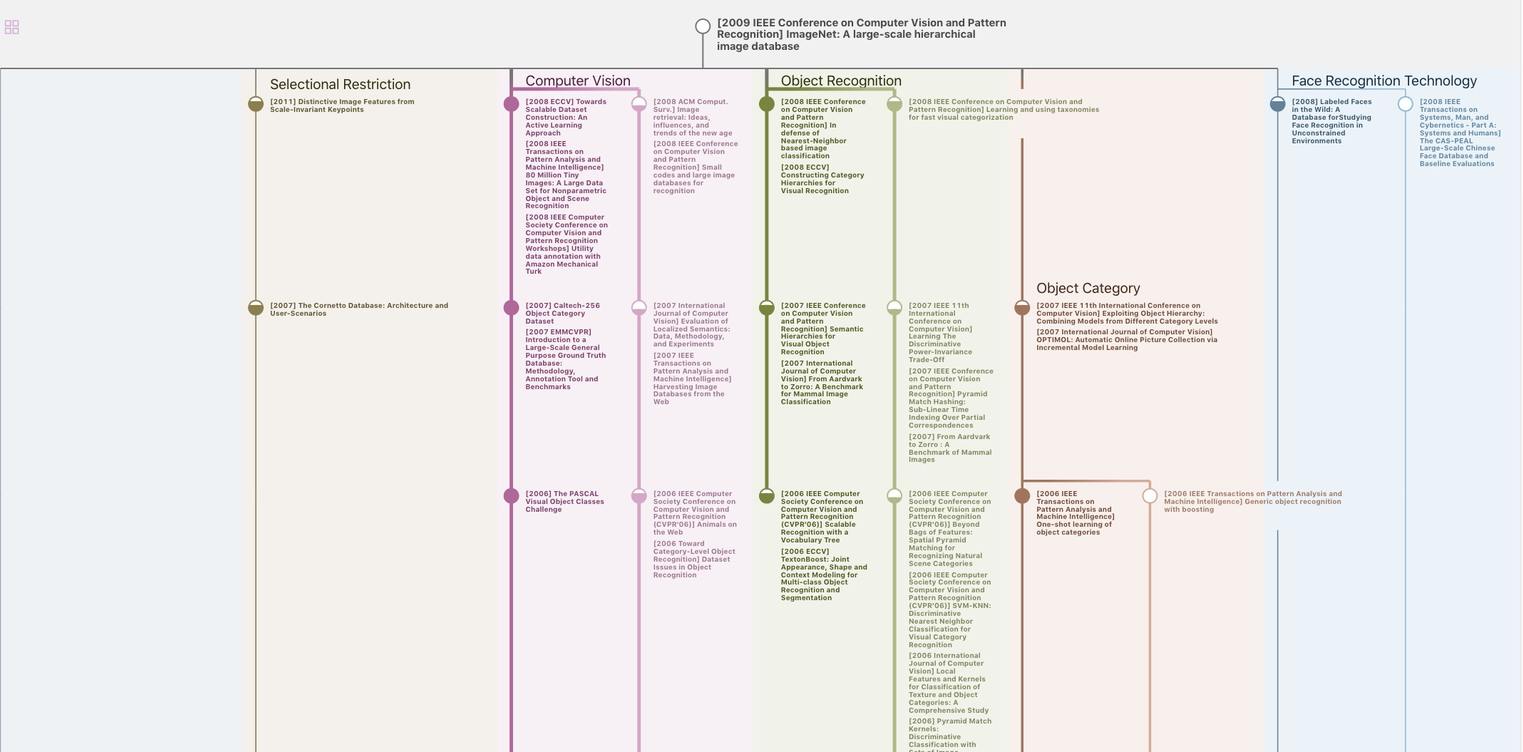
Generate MRT to find the research sequence of this paper
Chat Paper
Summary is being generated by the instructions you defined