Downloadable machine learning for compressed radiolocation applications in radio access networks
2020 IEEE Globecom Workshops (GC Wkshps(2020)
摘要
Machine learning (ML) is an important tool for enabling automation in radio access networks. Multiple ML-based applications utilize the device radio-fingerprint, for example to improve network functions such as paging and inter-frequency mobility. The measurements on the plurality of beams in NR can enable the network to get an improved radio-fingerprint in comparison to previous technologies (e.g. LTE), but with the cost of increased overhead in device measurements and signaling. In this paper, the device radio-fingerprint is denoted radiolocation. The increasing use of ML-based applications with detailed radiolocation not only increases the device signaling but also increases the input dimensionality and complexity of the ML model. Hence, it is essential to propose method(s) for reducing the dimensionality by compressing the radiolocation and reducing the overall signaling by downloading such compression method(s) to the device. In this paper, one such solution is proposed, and the challenges in downloading a compression model to the device is further highlighted. Moreover, the performances for two ML applications, namely secondary carrier prediction and beam prediction, with and without compressed radiolocation are compared. The results indicate that a compressed version of radiolocation can achieve prediction performance close to the uncompressed one.
更多查看译文
关键词
Machine learning,radiolocation,radio fingerprint
AI 理解论文
溯源树
样例
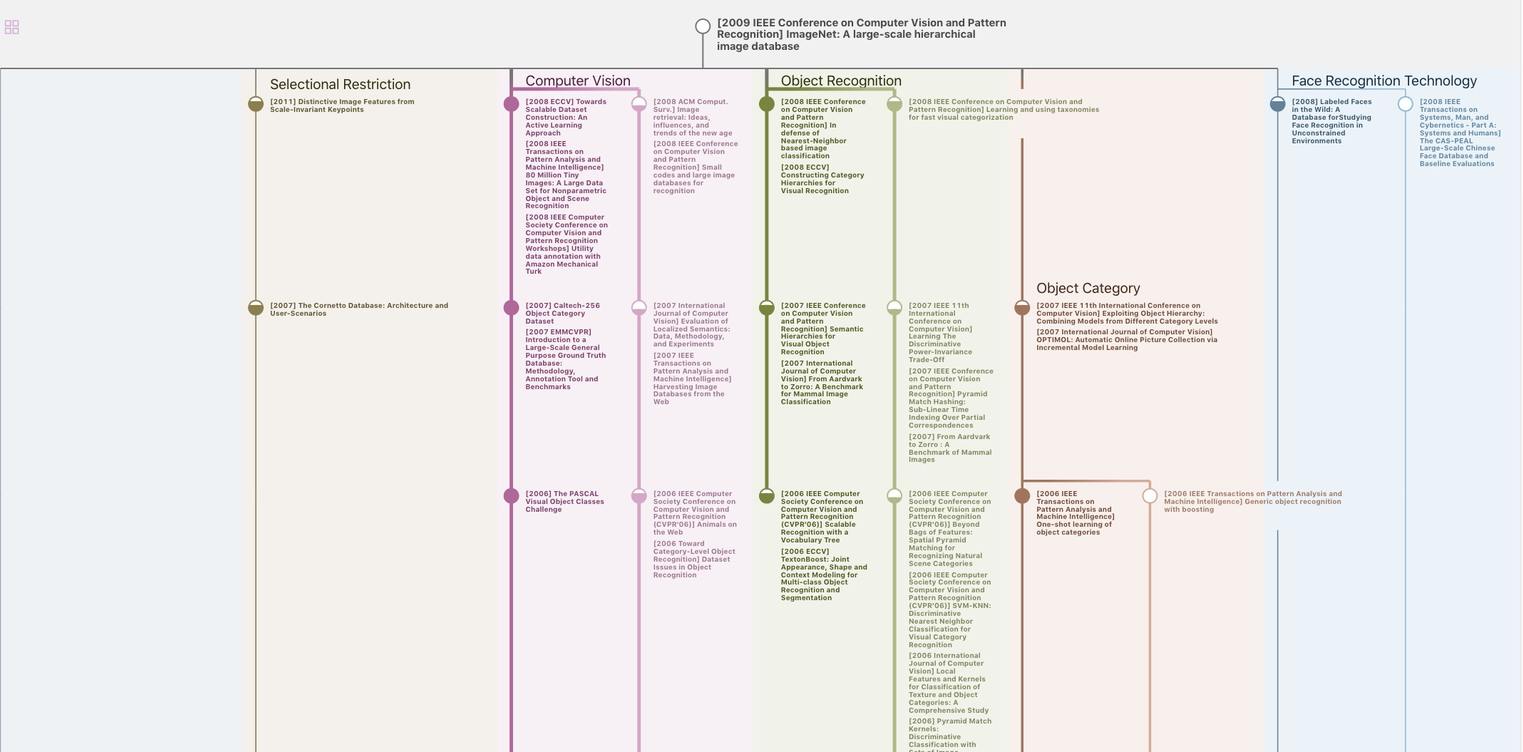
生成溯源树,研究论文发展脉络
Chat Paper
正在生成论文摘要