PromDA: Prompt-based Data Augmentation for Low-Resource NLU Tasks
PROCEEDINGS OF THE 60TH ANNUAL MEETING OF THE ASSOCIATION FOR COMPUTATIONAL LINGUISTICS (ACL 2022), VOL 1: (LONG PAPERS)(2022)
Abstract
This paper focuses on the Data Augmentation for low-resource Natural Language Understanding (NLU) tasks. We propose Prompt-based Data Augmentation model (PromDA) which only trains small-scale Soft Prompt (i.e., a set of trainable vectors) in the frozen Pre-trained Language Models (PLMs). This avoids human effort in collecting unlabeled in-domain data and maintains the quality of generated synthetic data. In addition, PromDA generates synthetic data via two different views and filters out the low-quality data using NLU models. Experiments on four benchmarks show that synthetic data produced by PromDA successfully boost up the performance of NLU models which consistently outperform several competitive baseline models, including a state-of-the-art semi-supervised model using unlabeled in-domain data. The synthetic data from PromDA are also complementary with unlabeled in-domain data. The NLU models can be further improved when they are combined for training.
MoreTranslated text
Key words
data augmentation,promda,prompt-based,low-resource
AI Read Science
Must-Reading Tree
Example
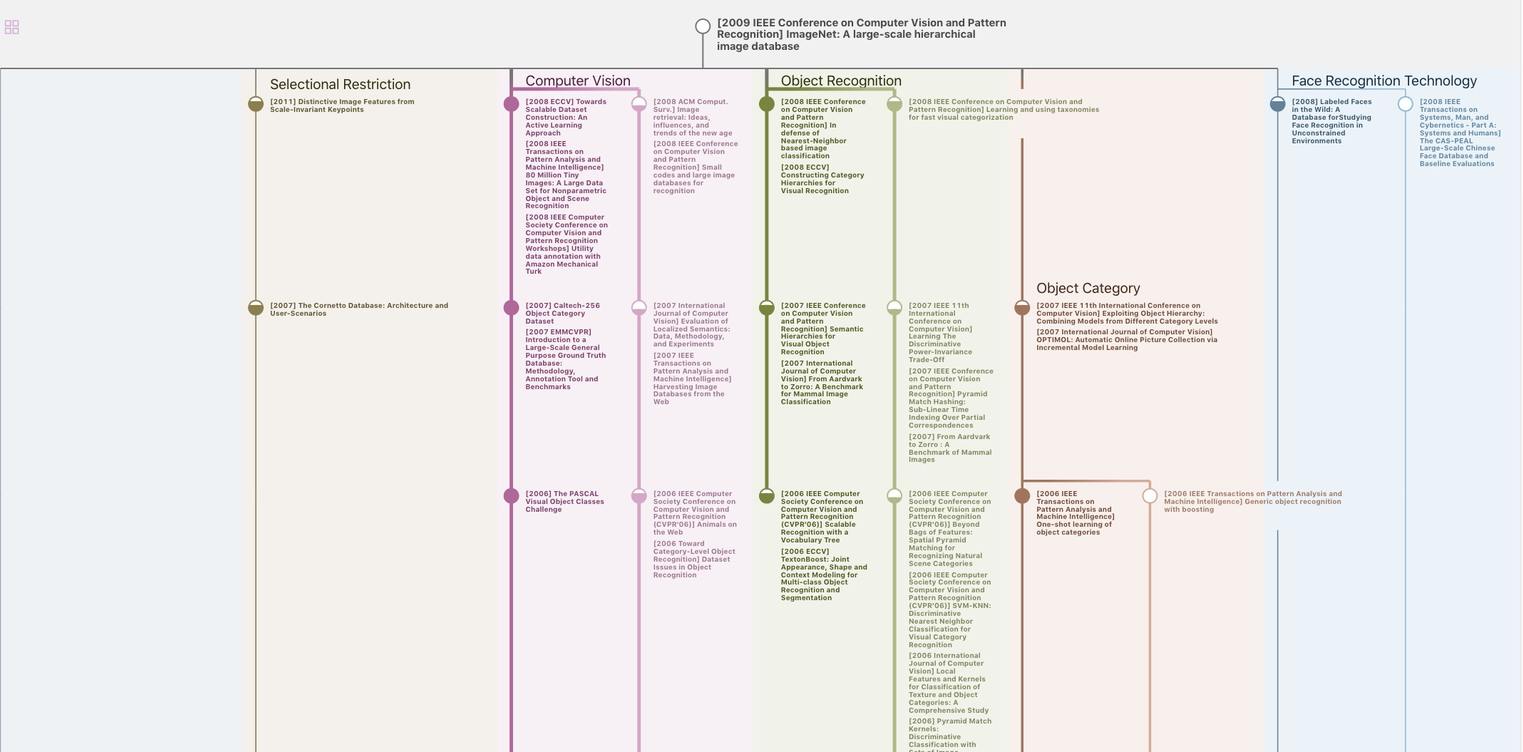
Generate MRT to find the research sequence of this paper
Chat Paper
Summary is being generated by the instructions you defined