Machine Learning for Short-Term Mortality in Acute Decompensation of Liver Cirrhosis: Better than MELD Score
DIAGNOSTICS(2024)
Abstract
Prediction of short-term mortality in patients with acute decompensation of liver cirrhosis could be improved. We aimed to develop and validate two machine learning (ML) models for predicting 28-day and 90-day mortality in patients hospitalized with acute decompensated liver cirrhosis. We trained two artificial neural network (ANN)-based ML models using a training sample of 165 out of 290 (56.9%) patients, and then tested their predictive performance against Model of End-stage Liver Disease-Sodium (MELD-Na) and MELD 3.0 scores using a different validation sample of 125 out of 290 (43.1%) patients. The area under the ROC curve (AUC) for predicting 28-day mortality for the ML model was 0.811 (95%CI: 0.714- 0.907; p < 0.001), while the AUC for the MELD-Na score was 0.577 (95%CI: 0.435-0.720; p = 0.226) and for MELD 3.0 was 0.600 (95%CI: 0.462-0.739; p = 0.117). The area under the ROC curve (AUC) for predicting 90-day mortality for the ML model was 0.839 (95%CI: 0.776- 0.884; p < 0.001), while the AUC for the MELD-Na score was 0.682 (95%CI: 0.575-0.790; p = 0.002) and for MELD 3.0 was 0.703 (95%CI: 0.590-0.816; p < 0.001). Our study demonstrates that ML-based models for predicting short-term mortality in patients with acute decompensation of liver cirrhosis perform significantly better than MELD-Na and MELD 3.0 scores in a validation cohort.
MoreTranslated text
Key words
machine learning,mortality prediction,liver cirrhosis,MELD-Na score,MELD 3.0 score,liver transplantation,transplant selection
AI Read Science
Must-Reading Tree
Example
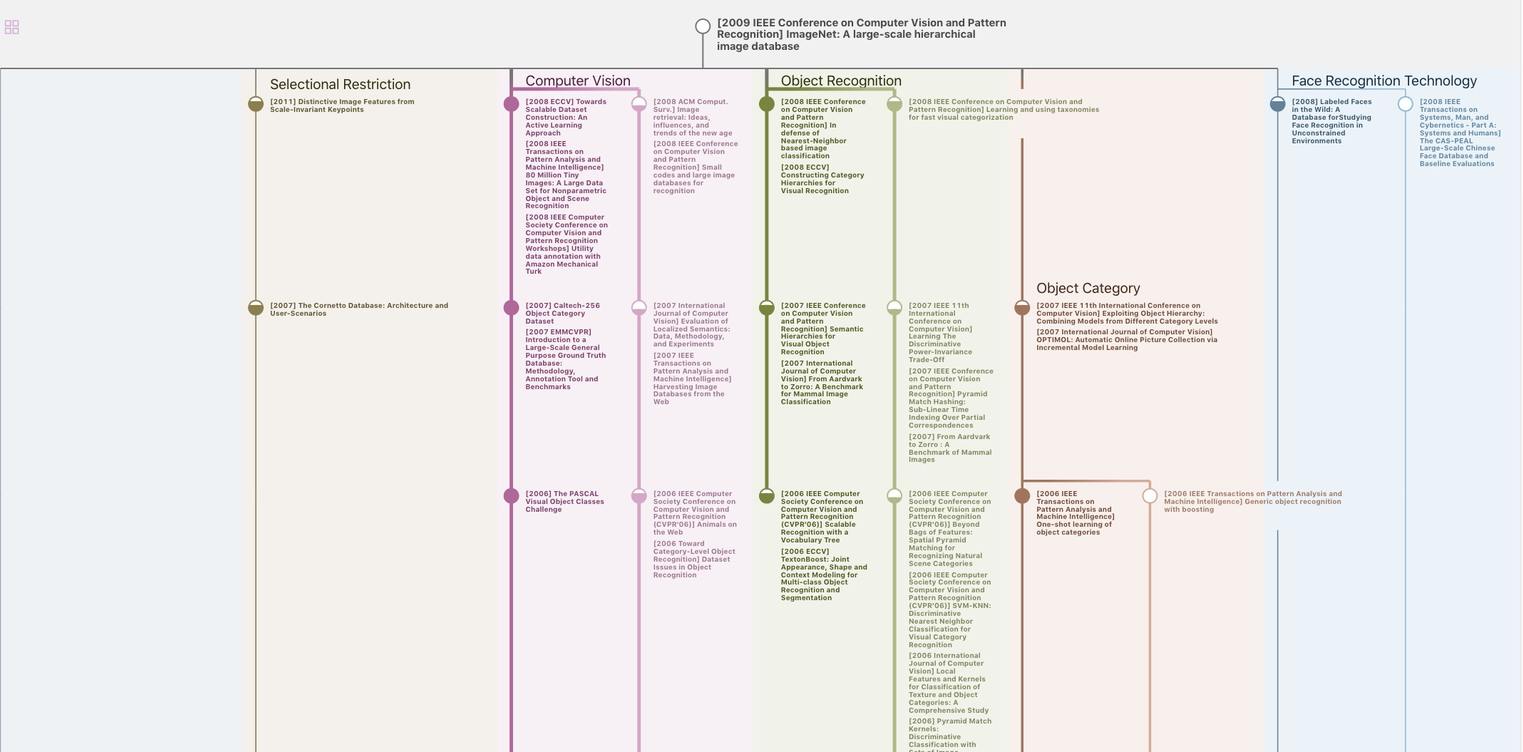
Generate MRT to find the research sequence of this paper
Chat Paper
Summary is being generated by the instructions you defined