A Closer Look at Self-supervised Lightweight Vision Transformers
ICLR 2023(2022)
摘要
Self-supervised learning on large-scale Vision Transformers (ViTs) as pre-training methods has achieved promising downstream performance. Yet, how such pre-training paradigms promote lightweight ViTs' performance is considerably less studied. In this work, we mainly produce recipes for pre-training high-performance lightweight ViTs using masked-image-modeling-based MAE, namely MAE-lite, which achieves 78.4% top-1 accuracy on ImageNet with ViT-Tiny (5.7M). Furthermore, we develop and benchmark other fully-supervised and self-supervised pre-training counterparts, e.g., contrastive-learning-based MoCo-v3, on both ImageNet and other classification tasks. We analyze and clearly show the effect of such pre-training, and reveal that properly-learned lower layers of the pre-trained models matter more than higher ones in data-sufficient downstream tasks. Finally, by further comparing with the pre-trained representations of the up-scaled models, a distillation strategy during pre-training is developed to improve the pre-trained representations as well, leading to further downstream performance improvement. The code and models will be made publicly available.
更多查看译文
关键词
Self-supervised Learning,Vision Transformers,Lightweight Networks
AI 理解论文
溯源树
样例
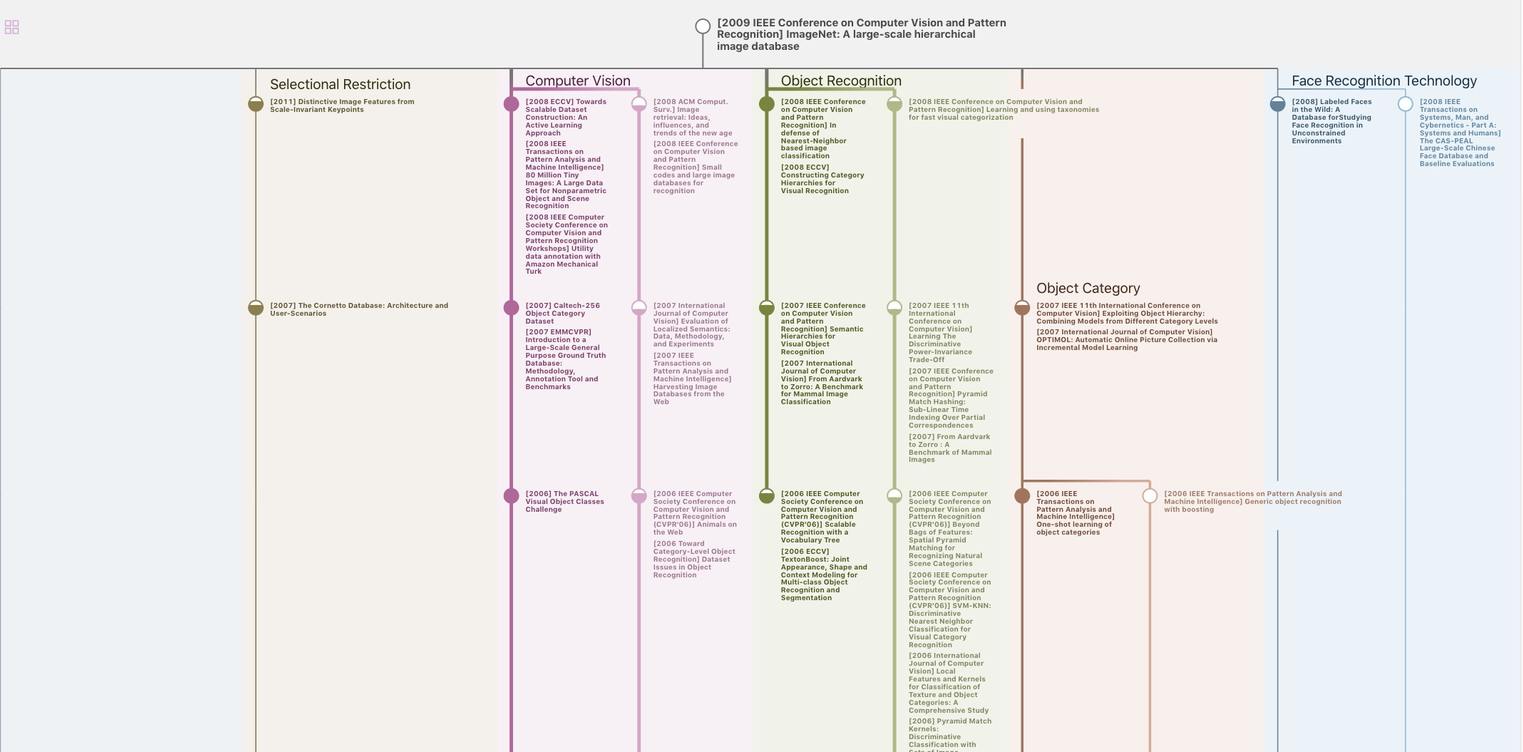
生成溯源树,研究论文发展脉络
Chat Paper
正在生成论文摘要