Data Preprocessing Technique for Neural Networks Based on Image Represented by a Fuzzy Function
IEEE Transactions on Fuzzy Systems(2020)
Abstract
Although data preprocessing is a universal technique that can be widely used in neural networks (NNs), most research in this area is focused on designing new NN architectures. This paper, we propose a preprocessing technique that enriches the original image data using local intensity information; this technique is motivated by human perception. To encode this information into an image, we introduce a new image structure named image represented by a fuzzy function. When using this structure, a crisp intensity value of each pixel is replaced by a fuzzy set given by a membership function constructed with the usage of extremal values from the particular neighborhood of that pixel. We describe this structure and its properties and propose a way in which it can be used as an input into existing NNs without any modifications. Based on our benchmark consisting of three well-known datasets and five NN architectures, we show that the proposed preprocessing can, in most cases, decrease classification error compared with a baseline and two other preprocessing methods. To support our claim, we have also selected several publicly available projects and tested the impact of the preprocessing with a positive result.
MoreTranslated text
Key words
Artificial neural networks,Fuzzy sets,Computer architecture,Data preprocessing,Standards,Task analysis
AI Read Science
Must-Reading Tree
Example
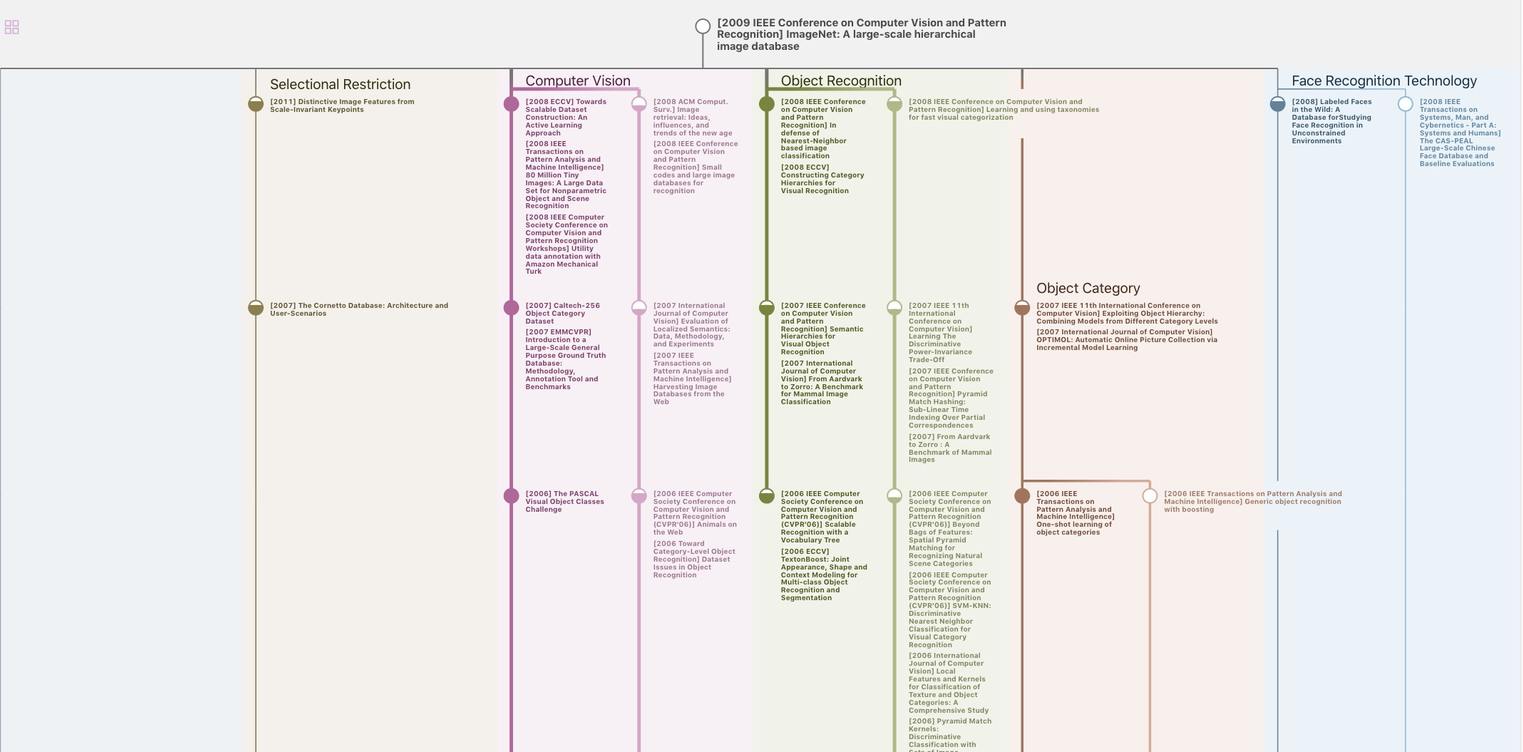
Generate MRT to find the research sequence of this paper
Chat Paper
Summary is being generated by the instructions you defined