An unsupervised learning model based on CT radiomics features accurately predicts axillary lymph node metastasis in breast cancer patients-diagnostic study.
International journal of surgery (London, England)(2024)
摘要
BACKGROUND:The accuracy of traditional clinical methods for assessing the metastatic status of axillary lymph nodes is unsatisfactory. In this study, we propose the use of radiomic technology and three-dimensional (3D) visualization technology to develop an unsupervised learning model for predicting axillary lymph node metastasis in patients with breast cancer, aiming to provide a new method for clinical axillary lymph node assessment in patients with this disease.
METHODS:In this study, we retrospectively analyzed the data of 350 patients with invasive breast cancer who underwent lung-enhanced CT and axillary lymph node dissection (ALND) surgery at the Department of Breast Surgery of the XXX Hospital of XXX University. We used 3D visualization technology to create a 3D atlas of axillary lymph nodes and identified the region of interest (ROI) for the lymph nodes. Radiomic features were subsequently extracted and selected, and a prediction model for axillary lymph nodes was constructed using the K-means unsupervised algorithm. To validate the model, we prospectively collected data from 128 breast cancer patients who were clinically evaluated as negative at our center.
RESULTS:Using 3D visualization technology, we extracted and selected a total of 36 CT radiomics features. The unsupervised learning model categorized 1737 unlabeled lymph nodes into two groups, and the analysis of the radiomic features between these groups indicated potential differences in lymph node status. Further validation with 1397 labeled lymph nodes demonstrated that the model had good predictive ability for axillary lymph node status, with an area under the curve (AUC) of 0.847 (0.825-0.869). Additionally, the model's excellent predictive performance was confirmed in the 128 axillary clinical assessment negative cohort (cN0) and the 350 clinical assessment positive (cN+) cohort, for which the correct classification rates (CCR) were 86.72% and 87.43%, respectively, which were significantly greater than those of clinical assessment methods.
CONCLUSIONS:We created an unsupervised learning model that accurately predicts the status of axillary lymph nodes. This approach offers a novel solution for the precise assessment of axillary lymph nodes in patients with breast cancer.
更多查看译文
AI 理解论文
溯源树
样例
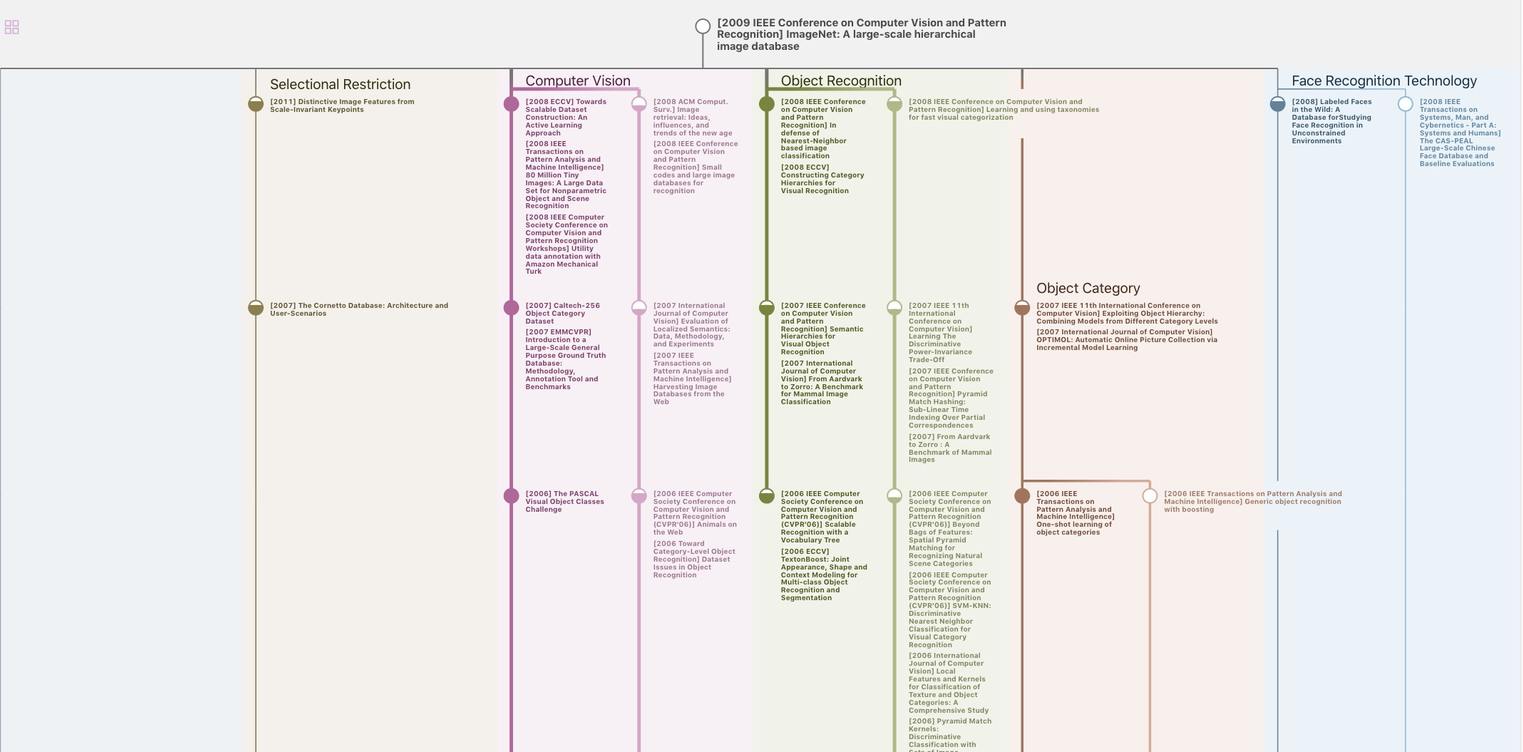
生成溯源树,研究论文发展脉络
Chat Paper
正在生成论文摘要