The Effectiveness of Self-supervised Pre-training for Multi-modal Endometriosis Classification
2023 45TH ANNUAL INTERNATIONAL CONFERENCE OF THE IEEE ENGINEERING IN MEDICINE & BIOLOGY SOCIETY, EMBC(2023)
摘要
Endometriosis is a debilitating condition affecting 5% to 10% of the women worldwide, where early detection and treatment are the best tools to manage the condition. Early detection can be done via surgery, but multi-modal medical imaging is preferable given the simpler and faster process. However, imaging-based endometriosis diagnosis is challenging as 1) there are few capable clinicians; and 2) it is characterised by small lesions unconfined to a specific location. These two issues challenge the development of endometriosis classifiers as the training datasets tend to be small and contain difficult samples, which leads to overfitting. Hence, it is important to consider generalisation techniques to mitigate this problem, particularly self-supervised pre-training methods that have shown outstanding results in computer vision and natural language processing applications. The main goal of this paper is to study the effectiveness of modern self-supervised pre-training techniques to overcome the two issues mentioned above for the classification of endometriosis from multi-modal imaging data. We also introduce a new masking image modelling self-supervised pre-training method that works with 3D multi-modal medical imaging. Furthermore, to the best of our knowledge, this paper presents the first endometriosis classifier, fine-tuned from the pre-trained model above, which works with multimodal (i.e., T1 and T2) magnetic resonance imaging (MRI) data. Our results show that self-supervised pre-training improves endometriosis classification by as much as 31%, when compared with classifiers trained from scratch.
更多查看译文
关键词
Self-supervision,Multi-modal learning,MRI,Endometriosis
AI 理解论文
溯源树
样例
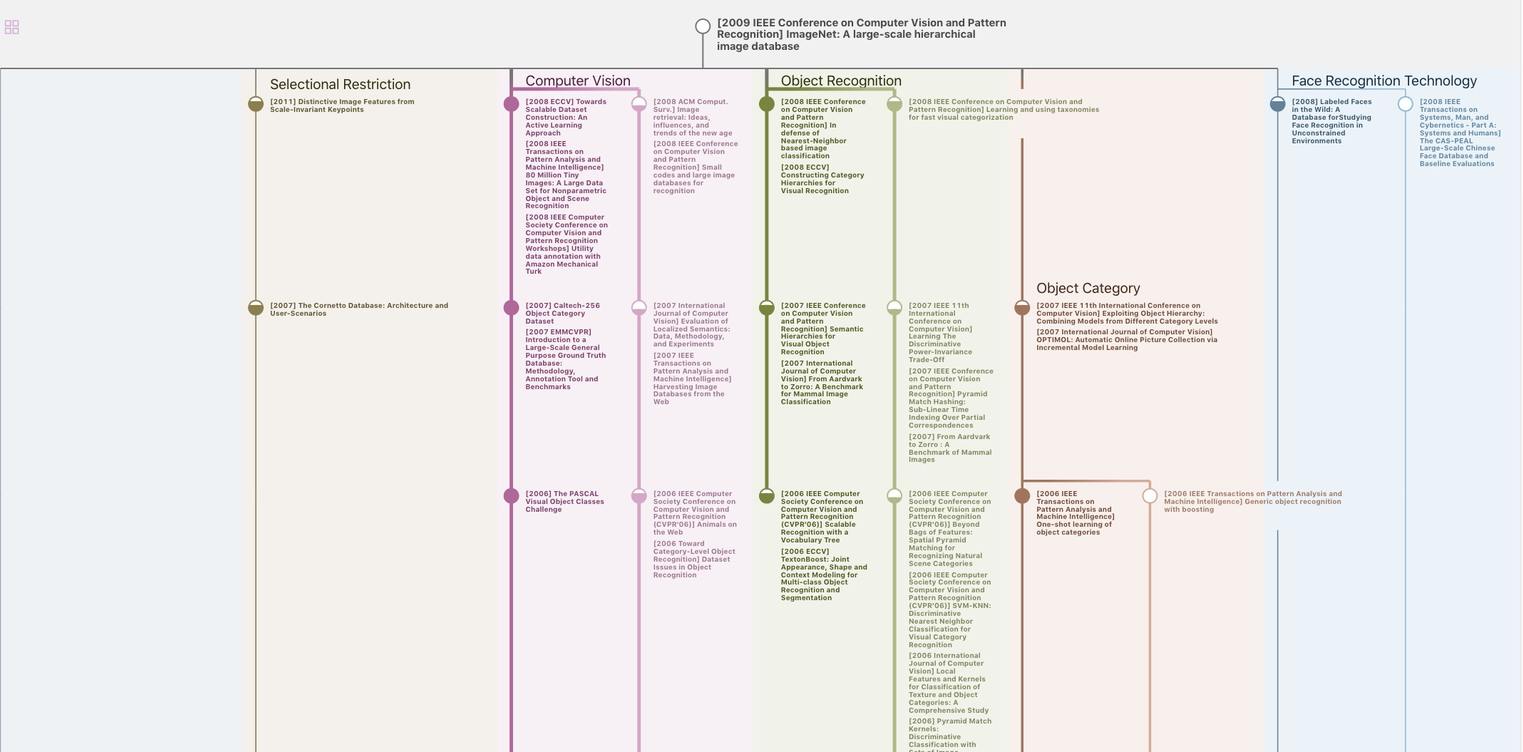
生成溯源树,研究论文发展脉络
Chat Paper
正在生成论文摘要