Hyperspectral Image Denoising via Weighted Multidirectional Low-Rank Tensor Recovery.
IEEE transactions on cybernetics(2023)
摘要
Recently, low-rank tensor recovery methods based on subspace representation have received increased attention in the field of hyperspectral image (HSI) denoising. Unfortunately, those methods usually analyze the prior structural information within different dimensions indiscriminately, ignoring the differences between modes, leaving substantial room for improvement. In this article, we first consider the low-rank properties in the subspace and prove that the structure correlation across the nonlocal self-similarity mode is much stronger than in the spatial sparsity and spectral correlation modes. On that basis, we introduce a new multidirectional low-rank regularization, in which each mode is assigned a different weight to characterize its contribution to estimating the tensor rank. After that, integrating the proposed regularization with the subspace-based tensor recovery framework, an optimization model for HSI mixed noise removal is developed. The proposed model can be addressed efficiently via the alternating minimization algorithm. Extensive experiments implemented with synthetic and real data demonstrate that the proposed method significantly outperforms other state-of-the-art HSI denoising methods, which clearly indicates the effectiveness of the proposed approach in HSI denoising.
更多查看译文
关键词
Hyperspectral image (HSI) denoising,low-dimensional subspace,low-rank tensor approximation (LRTA),mixed noise
AI 理解论文
溯源树
样例
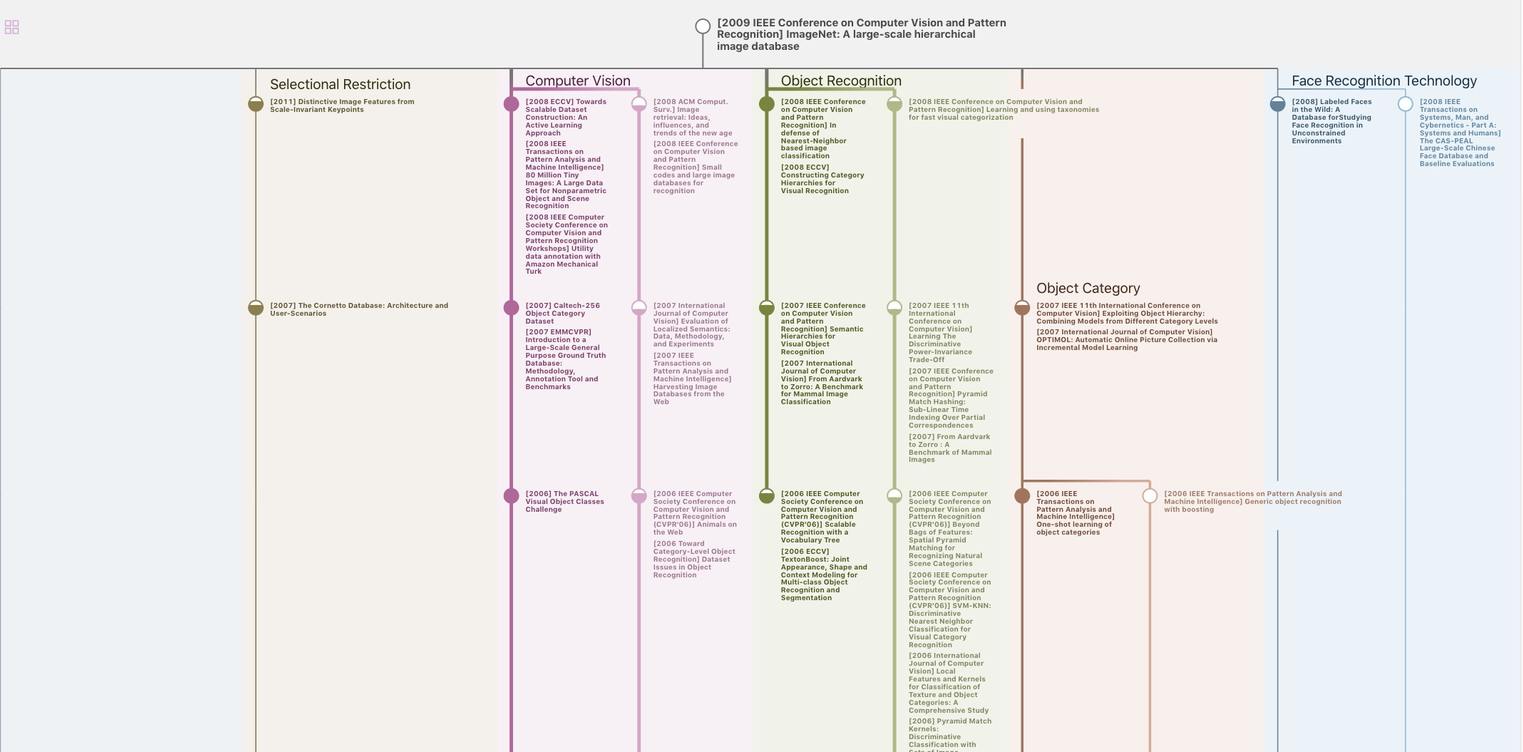
生成溯源树,研究论文发展脉络
Chat Paper
正在生成论文摘要