Accelerating Wireless Federated Learning With Adaptive Scheduling Over Heterogeneous Devices
IEEE INTERNET OF THINGS JOURNAL(2024)
摘要
As the proliferation of sophisticated task models in 5G-empowered digital twin, it yields significant demands on fast and accurate model training over resource-limited wireless networks. It is vital to investigate how to accelerate the training process based on the salient features of practical systems, including heterogeneous data distributions and system resources both across devices and over time. To study the nontrivial coupling between participating device selection and their appropriate training parameters, we first characterize the dependency of convergence performance bound on system parameters, i.e., statistical structure of local data, mini-batch size, and gradient quantization level. Based on the theoretical analysis, a training efficiency optimization problem is formulated subject to heterogeneous communication and computation capabilities among devices. To realize online control of training parameters, we propose an adaptive batch-size-assisted device scheduling strategy, which prioritizes the selection of devices that offer good data utility and dynamically adjust their mini-batch sizes and gradient quantization levels adapting to network conditions. Simulation results demonstrate that our proposed strategy can effectively speed up the training process as compared with benchmark algorithms.
更多查看译文
关键词
Adaptive batch sizes,convergence analysis,device heterogeneity,gradient quantization,wireless federated learning
AI 理解论文
溯源树
样例
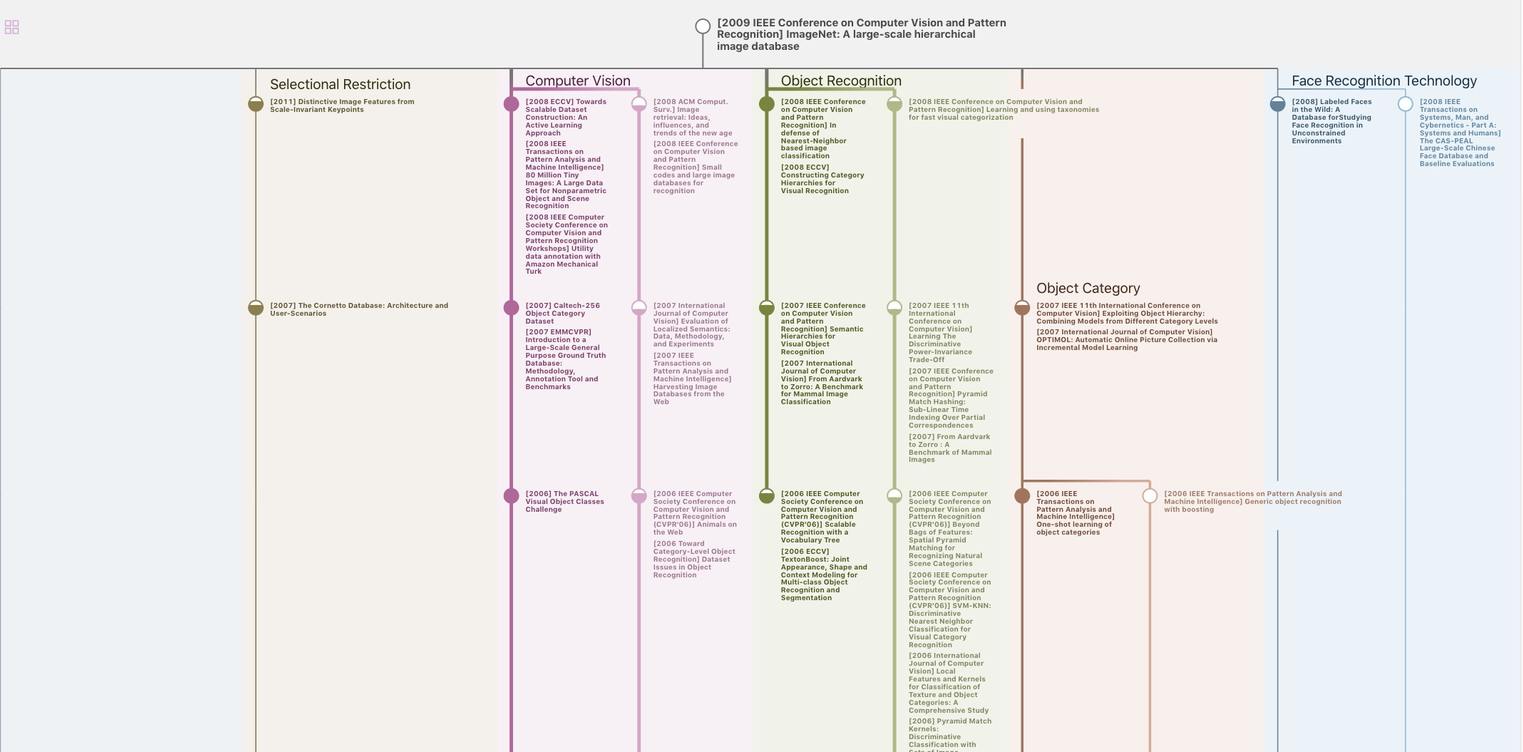
生成溯源树,研究论文发展脉络
Chat Paper
正在生成论文摘要