Deep Autoencoding One-Class time Series Anomaly Detection
ICASSP 2023 - 2023 IEEE International Conference on Acoustics, Speech and Signal Processing (ICASSP)(2023)
摘要
Time-series Anomaly Detection(AD) is widely used in monitoring and security applications in various industries and has become a hot spot in the field of deep learning. Normality-representation-based methods perform well in certain scenarios but may ignore some aspects of the overall normality. Feature-extraction-based methods always take a process of pre-training, whose target differs from AD, leading to a decline in AD performance. In this paper, we propose a new AD method called deep Autoencoding One-Class (AOC), which learns features with AutoEncoder(AE). Meanwhile, the normal context vectors from AE are constrained into a hypersphere small enough, similar to one-class methods. With an objective function that optimizes the two assumptions simultaneously, AOC learns various aspects of normality, which is more effective for AD. Experiments on public datasets show that our method outperforms existing baseline approaches.
更多查看译文
关键词
Time Series Anomaly Detection,Autoen-coder,One-class classification
AI 理解论文
溯源树
样例
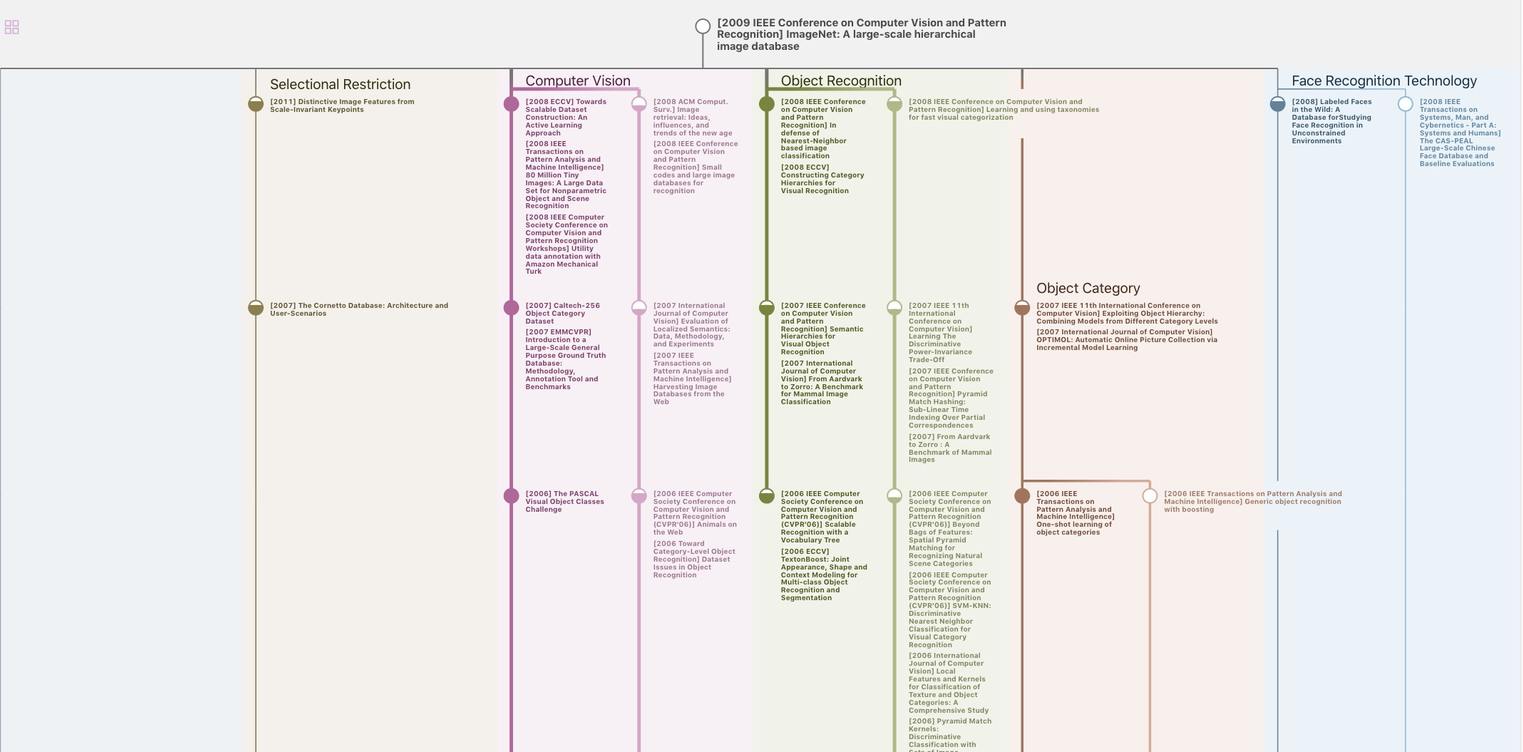
生成溯源树,研究论文发展脉络
Chat Paper
正在生成论文摘要