A New Adaptation Phase for Thresholds in a CBR System Associated to a Region Growing Algorithm to Segment Tumoral Kidneys.
ICCBR(2020)
Abstract
Image segmentation is an abundant topic for computer vision and image processing. Most of the time, segmentation is not fully automated, and a user is required to guide the process in order to obtain correct results. Yet, even with programs, it is a time-consuming process. In a medical context, segmentation can provide a lot of information to surgeons, but since this task is manual, it is rarely executed because of time. Artificial Intelligence (AI) is a powerful approach to create viable solutions for automated treatments. In this paper, we reused a case-based reasoning (CBR) system previously developed to segment renal parenchyma with a region growing algorithm and we completed its adaptation phase allowing a better adjustment of parameters before segmentation. Compared to the previous system, we added an adaptation for the thresholds values in addition to the adaptation of the seeds coordinates. We compared several versions of our new adaptation in order to determine the best and we confronted it with a deep learning approach realized in similar conditions.
MoreTranslated text
Key words
Case-Based Reasoning,Convolution Neural Network,Segmentation,Cancer tumour,Healthcare imaging,Artificial Intelligence
AI Read Science
Must-Reading Tree
Example
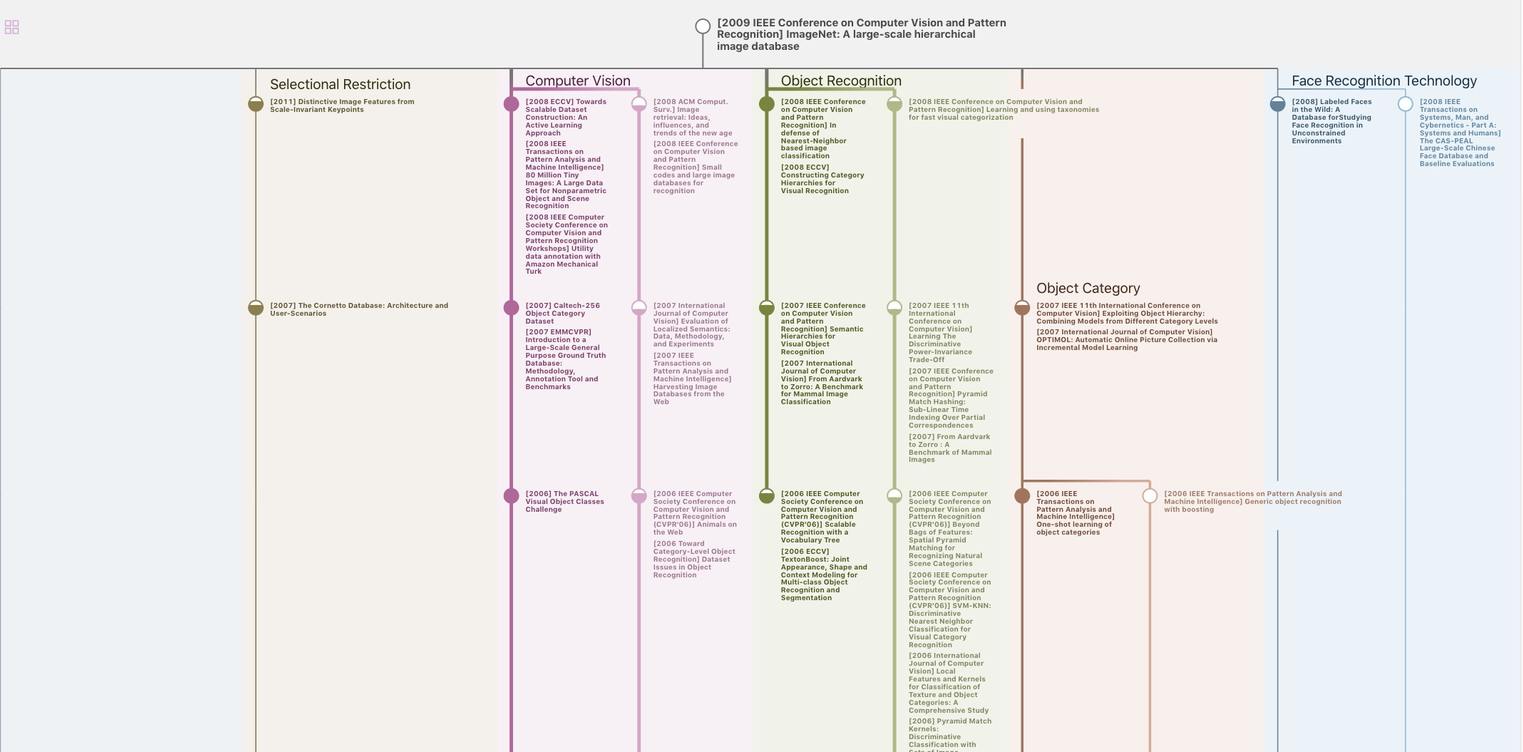
Generate MRT to find the research sequence of this paper
Chat Paper
Summary is being generated by the instructions you defined