Deep-Learning-Based Production Decline Curve Analysis in the Gas Reservoir through Sequence Learning Models
CMES-COMPUTER MODELING IN ENGINEERING & SCIENCES(2022)
摘要
Production performance prediction of tight gas reservoirs is crucial to the estimation of ultimate recovery, which has an important impact on gas field development planning and economic evaluation. Owing to the model's simplicity, the decline curve analysis method has been widely used to predict production performance. The advancement of deep-learning methods provides an intelligent way of analyzing production performance in tight gas reservoirs. In this paper, a sequence learning method to improve the accuracy and efficiency of tight gas production forecasting is proposed. The sequence learning methods used in production performance analysis herein include the recurrent neural network (RNN), long short-term memory (LSTM) neural network, and gated recurrent unit (GRU) neural network, and their performance in the tight gas reservoir production prediction is investigated and compared. To further improve the performance of the sequence learning method, the hyperparameters in the sequence learning methods are optimized through a particle swarm optimization algorithm, which can greatly simplify the optimization process of the neural network model in an automated manner. Results show that the optimized GRU and RNN models have more compact neural network structures than the LSTM model and that the GRU is more efficiently trained. The predictive performance of LSTM and GRU is similar, and both are better than the RNN and the decline curve analysis model and thus can be used to predict tight gas production.
更多查看译文
关键词
Tight gas, production forecasting, deep learning, sequence learning models
AI 理解论文
溯源树
样例
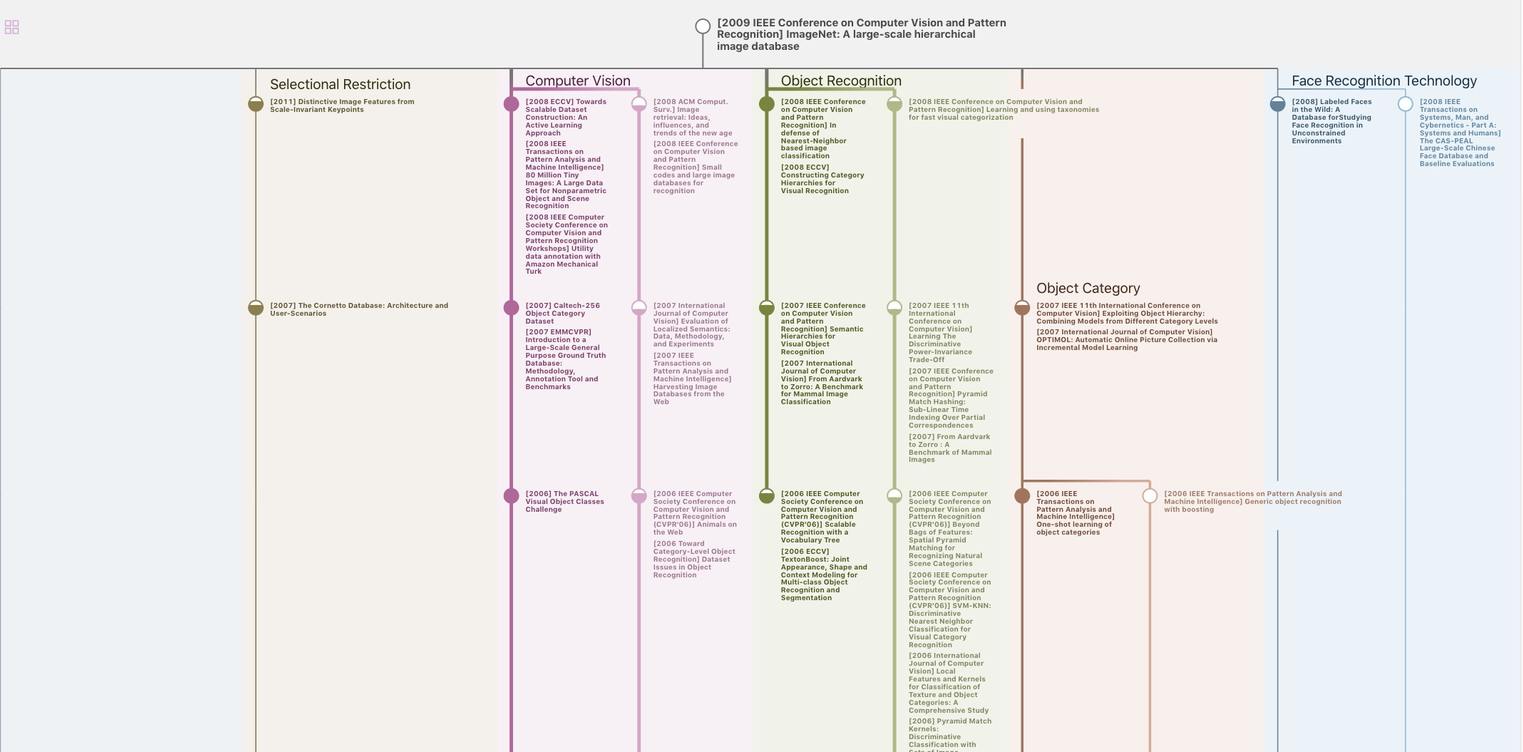
生成溯源树,研究论文发展脉络
Chat Paper
正在生成论文摘要