False Claims against Model Ownership Resolution
CoRR(2023)
摘要
Deep neural network (DNN) models are valuable intellectual property of model owners, constituting a competitive advantage. Therefore, it is crucial to develop techniques to protect against model theft. Model ownership resolution (MOR) is a class of techniques that can deter model theft. A MOR scheme enables an accuser to assert an ownership claim for a suspect model by presenting evidence, such as a watermark or fingerprint, to show that the suspect model was stolen or derived from a source model owned by the accuser. Most of the existing MOR schemes prioritize robustness against malicious suspects, ensuring that the accuser will win if the suspect model is indeed a stolen model. In this paper, we show that common MOR schemes in the literature are vulnerable to a different, equally important but insufficiently explored, robustness concern: a malicious accuser. We show how malicious accusers can successfully make false claims against independent suspect models that were not stolen. Our core idea is that a malicious accuser can deviate (without detection) from the specified MOR process by finding (transferable) adversarial examples that successfully serve as evidence against independent suspect models. To this end, we first generalize the procedures of common MOR schemes and show that, under this generalization, defending against false claims is as challenging as preventing (transferable) adversarial examples. Via systematic empirical evaluation we demonstrate that our false claim attacks always succeed in all prominent MOR schemes with realistic configurations, including against a real-world model: Amazon's Rekognition API.
更多查看译文
关键词
false claims,ownership,model
AI 理解论文
溯源树
样例
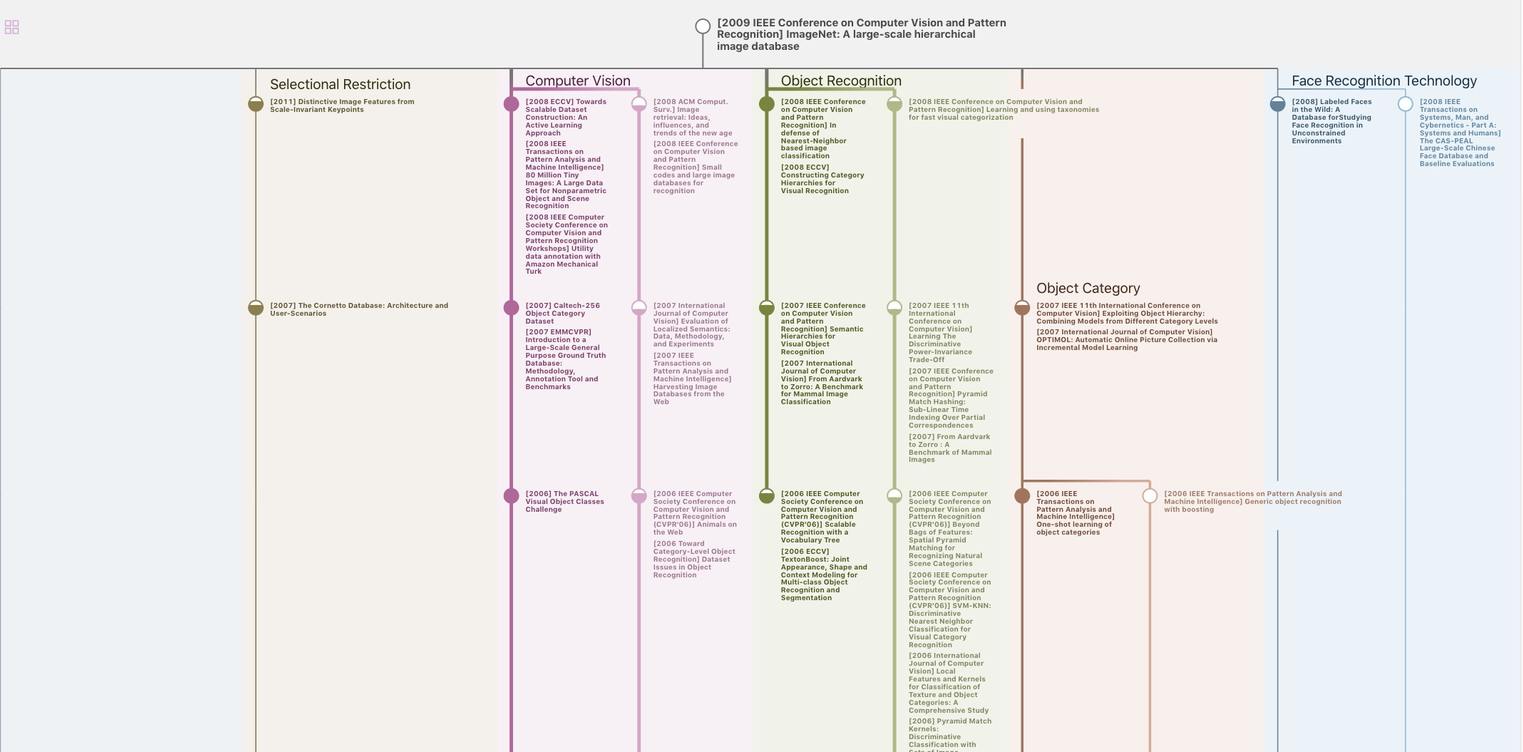
生成溯源树,研究论文发展脉络
Chat Paper
正在生成论文摘要