Enhancing Deep Reinforcement Learning: A Tutorial on Generative Diffusion Models in Network Optimization
IEEE Communications Surveys & Tutorials(2023)
摘要
Generative Diffusion Models (GDMs) have emerged as a transformative force in
the realm of Generative Artificial Intelligence (GenAI), demonstrating their
versatility and efficacy across various applications. The ability to model
complex data distributions and generate high-quality samples has made GDMs
particularly effective in tasks such as image generation and reinforcement
learning. Furthermore, their iterative nature, which involves a series of noise
addition and denoising steps, is a powerful and unique approach to learning and
generating data. This paper serves as a comprehensive tutorial on applying GDMs
in network optimization tasks. We delve into the strengths of GDMs, emphasizing
their wide applicability across various domains, such as vision, text, and
audio generation. We detail how GDMs can be effectively harnessed to solve
complex optimization problems inherent in networks. The paper first provides a
basic background of GDMs and their applications in network optimization. This
is followed by a series of case studies, showcasing the integration of GDMs
with Deep Reinforcement Learning (DRL), incentive mechanism design, Semantic
Communications (SemCom), Internet of Vehicles (IoV) networks, etc. These case
studies underscore the practicality and efficacy of GDMs in real-world
scenarios, offering insights into network design. We conclude with a discussion
on potential future directions for GDM research and applications, providing
major insights into how they can continue to shape the future of network
optimization.
更多查看译文
关键词
Diffusion model,deep reinforcement learning,generative AI,AI-generated content,network optimization
AI 理解论文
溯源树
样例
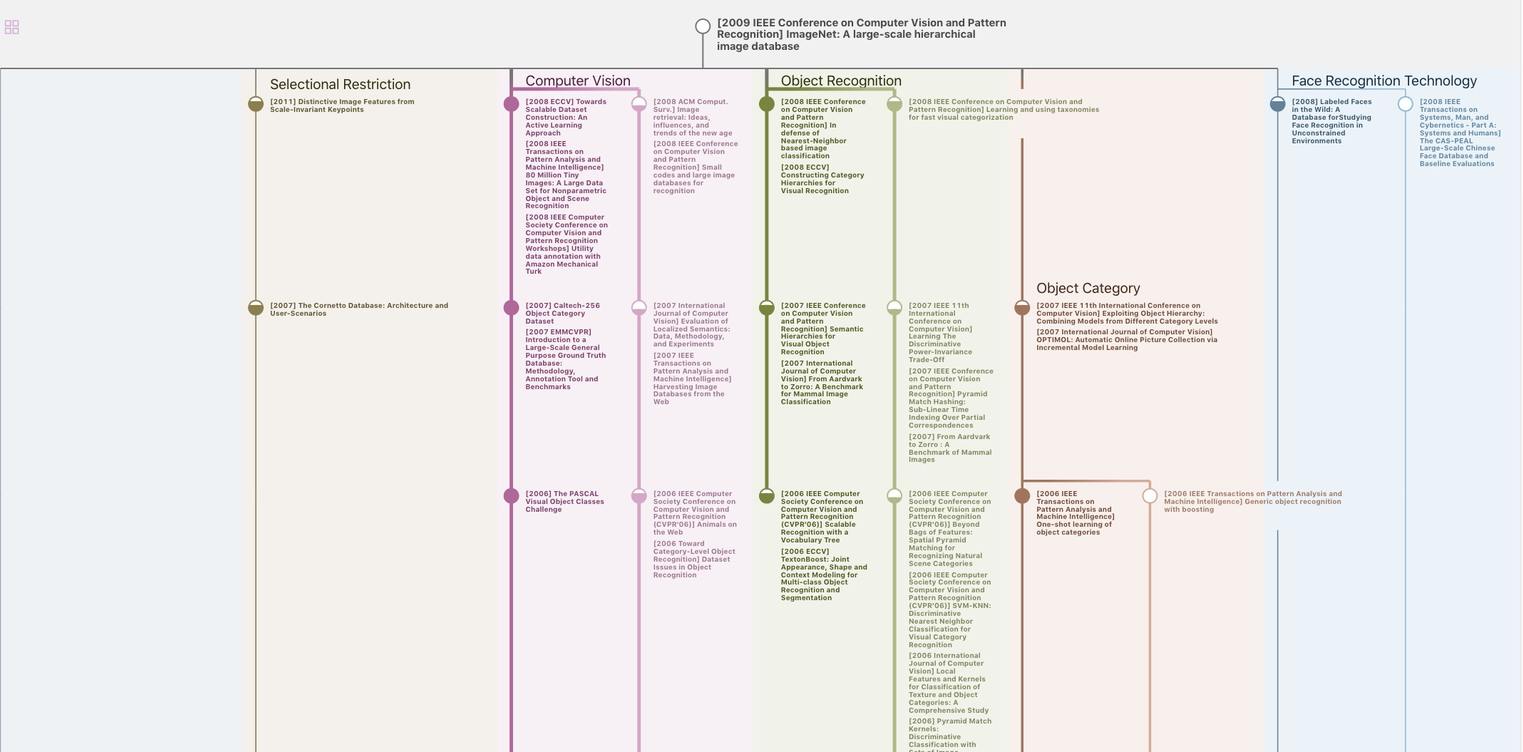
生成溯源树,研究论文发展脉络
Chat Paper
正在生成论文摘要