Ant colony optimization with multi-agent evolution for detecting functional modules in protein-protein interaction networks
ICICA (LNCS)(2012)
Abstract
Functional module identification in a Protein-Protein Interaction (PPI) network is one of the most important and challenging tasks in computational biology. For detecting functional modules, it is difficult to solve the problem directly and always results in a low accuracy and a large discard rate. In this paper, we present a novel algorithm of ant colony optimization with multi-agent evolution for detecting functional modules. The proposed ACO-MAE algorithm enhances the performance of ant colony optimization (ACO) by incorporating multi-agent evolution (MAE). First, the ant colony optimization for solving Traveling Salesman Problems (TSP) is conducted to construct primary clustering results. Then, the multi-agent evolutionary process is performed to move out of local optima. From simulation results, it is shown that the proposed ACO-MAE algorithm has superior performance when compared to other existing algorithms.
MoreTranslated text
Key words
existing algorithm,multi-agent evolution,ant colony optimization,protein-protein interaction network,protein-protein interaction,functional module,proposed aco-mae algorithm,multi-agent evolutionary process,novel algorithm,superior performance,functional module identification
AI Read Science
Must-Reading Tree
Example
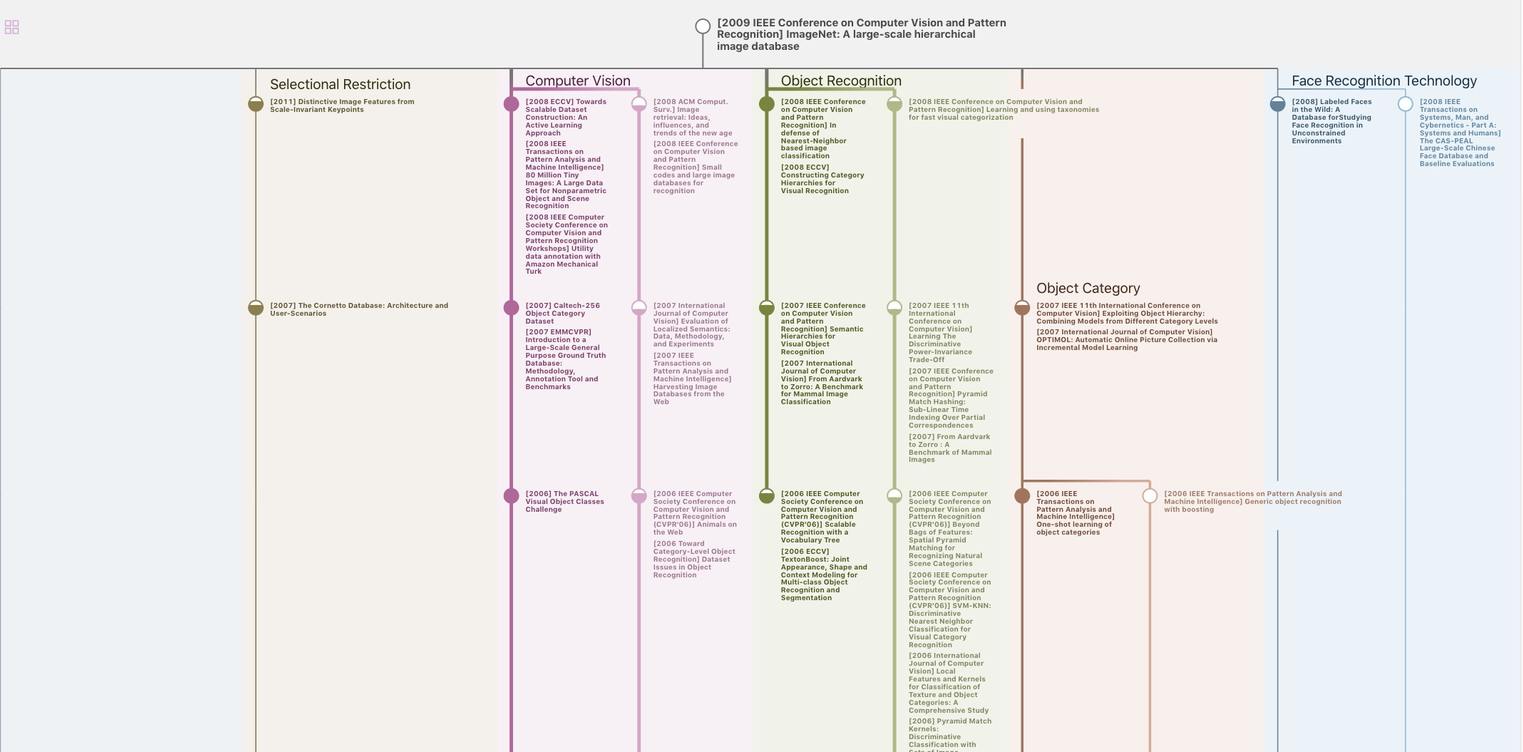
Generate MRT to find the research sequence of this paper
Chat Paper
Summary is being generated by the instructions you defined