Inference for Generative Capsule Models
arxiv(2021)
摘要
Capsule networks (see e.g. Hinton et al., 2018) aim to encode knowledge and reason about the relationship between an object and its parts. % In this paper we focus on a clean version of this problem, where data is generated from multiple geometric objects (e.g. triangles, squares) at arbitrary translations, rotations and scales, and the observed datapoints (parts) come from the corners of all objects, without any labelling of the objects. We specify a generative model for this data, and derive a variational algorithm for inferring the transformation of each object and the assignments of points to parts of the objects. Recent work by Kosiorek et al. [2019] has used amortized inference via stacked capsule autoencoders (SCA) to tackle this problem -- our results show that we significantly outperform them. We also investigate inference for this problem using a RANSAC-type algorithm.
更多查看译文
关键词
generative capsule models
AI 理解论文
溯源树
样例
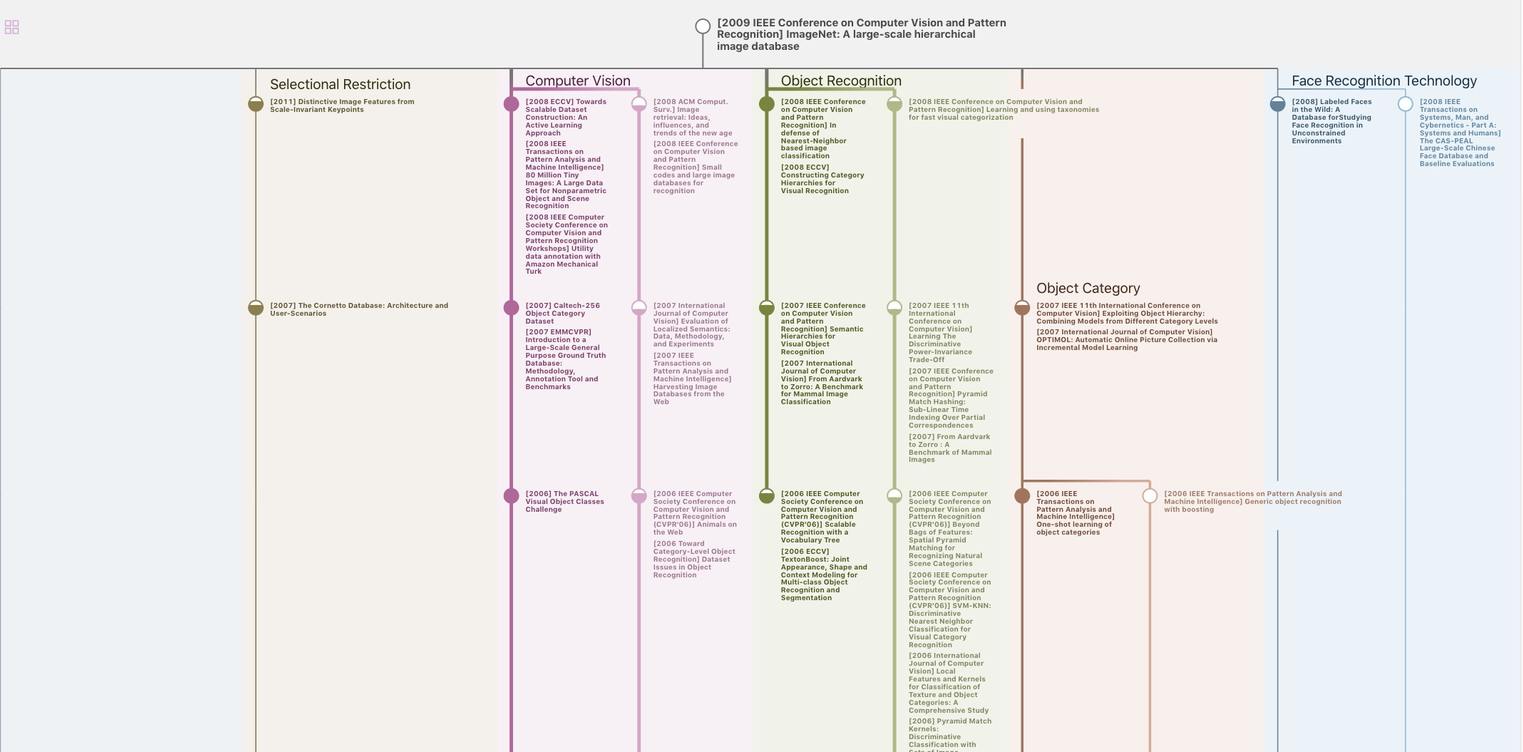
生成溯源树,研究论文发展脉络
Chat Paper
正在生成论文摘要