Deep Graph Matching under Quadratic Constraint
2021 IEEE/CVF CONFERENCE ON COMPUTER VISION AND PATTERN RECOGNITION, CVPR 2021(2021)
摘要
Recently, deep learning based methods have demonstrated promising results on the graph matching problem, by relying on the descriptive capability of deep features extracted on graph nodes. However, one main limitation with existing deep graph matching (DGM) methods lies in their ignorance of explicit constraint of graph structures, which may lead the model to be trapped into local minimum in training. In this paper, we propose to explicitly formulate pairwise graph structures as a quadratic constraint incorporated into the DGM framework. The quadratic constraint minimizes the pairwise structural discrepancy between graphs, which can reduce the ambiguities brought by only using the extracted CNN features. Moreover, we present a differentiable implementation to the quadratic constrained-optimization such that it is compatible with the unconstrained deep learning optimizer. To give more precise and proper supervision, a well-designed false matching loss against class imbalance is proposed, which can better penalize the false negatives and false positives with less overfitting. Exhaustive experiments demonstrate that our method achieves competitive performance on real-world datasets. The code is available at: https://github.com/zerg-Overmind/QC-DGM.
更多查看译文
关键词
extracted CNN features,unconstrained deep learning optimizer,false matching loss,deep learning based methods,graph matching problem,descriptive capability,deep features,graph nodes,deep graph matching methods,explicit constraint,pairwise graph structures,DGM framework,quadratic constraint,pairwise structural discrepancy
AI 理解论文
溯源树
样例
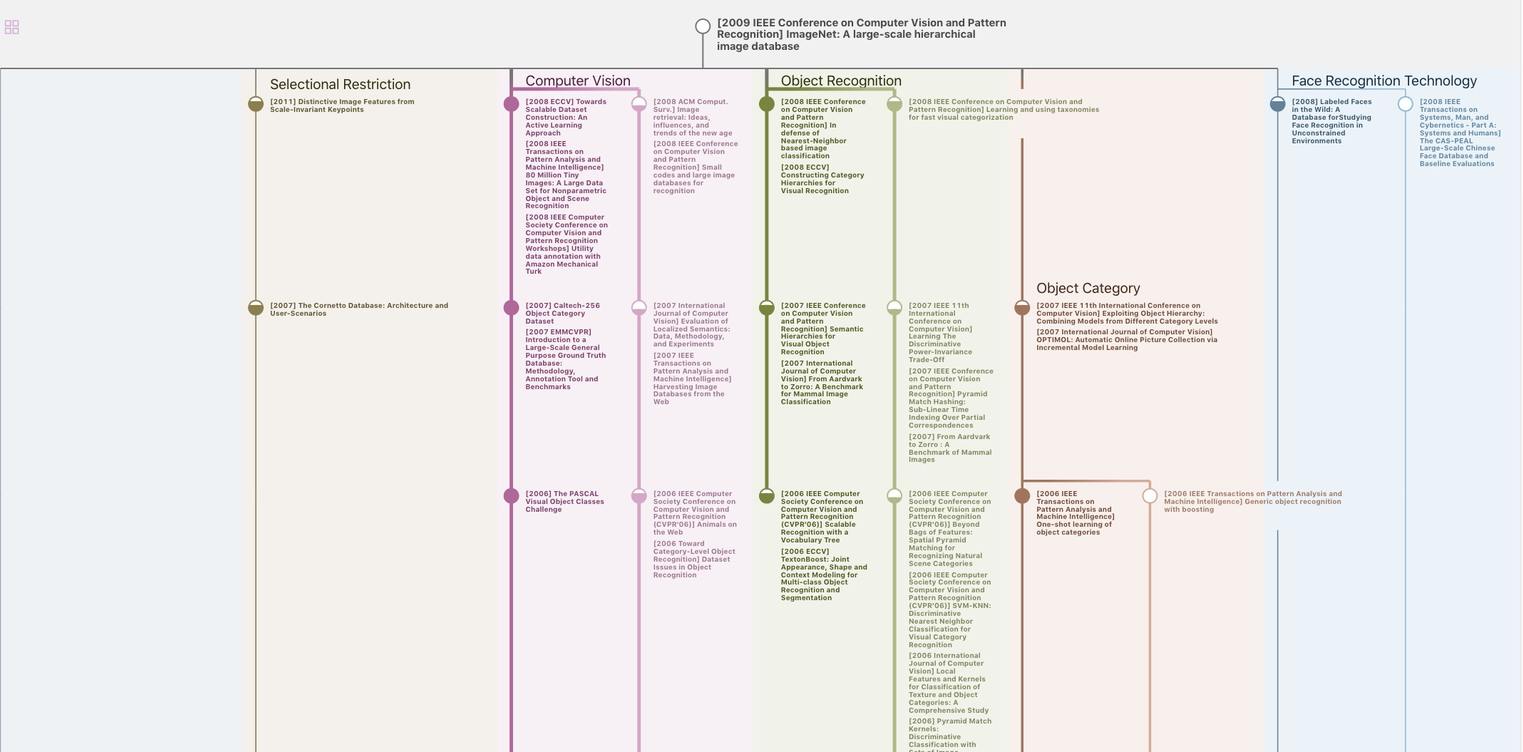
生成溯源树,研究论文发展脉络
Chat Paper
正在生成论文摘要