Conversational Answer Generation and Factuality for Reading Comprehension Question-Answering
CoRR(2021)
Abstract
Question answering (QA) is an important use case on voice assistants. A popular approach to QA is extractive reading comprehension (RC) which finds an answer span in a text passage. However, extractive answers are often unnatural in a conversational context which results in suboptimal user experience. In this work, we investigate conversational answer generation for QA. We propose AnswerBART, an end-to-end generative RC model which combines answer generation from multiple passages with passage ranking and answerability. Moreover, a hurdle in applying generative RC are hallucinations where the answer is factually inconsistent with the passage text. We leverage recent work from summarization to evaluate factuality. Experiments show that AnswerBART significantly improves over previous best published results on MS MARCO 2.1 NLGEN by 2.5 ROUGE-L and NarrativeQA by 9.4 ROUGE-L.
MoreTranslated text
AI Read Science
Must-Reading Tree
Example
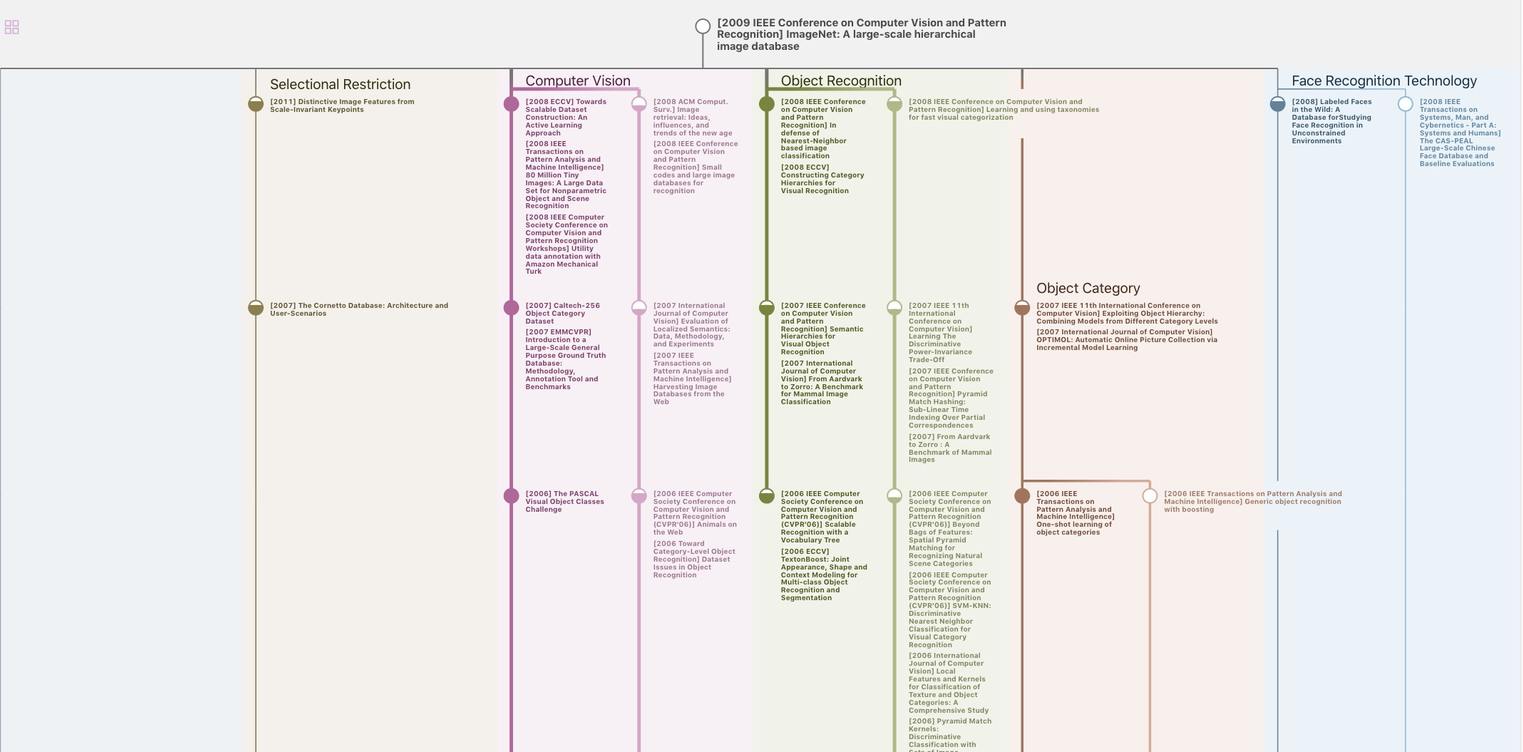
Generate MRT to find the research sequence of this paper
Chat Paper
Summary is being generated by the instructions you defined