Causal inference with misspecified exposure mappings: separating definitions and assumptions
arxiv(2023)
摘要
Exposure mappings facilitate investigations of complex causal effects when units interact in experiments. Current methods require experimenters to use the same exposure mappings to define the effect of interest and to impose assumptions on the interference structure. However, the two roles rarely coincide in practice, and experimenters are forced to make the often questionable assumption that their exposures are correctly specified. This paper argues that the two roles exposure mappings currently serve can, and typically should, be separated, so that exposures are used to define effects without necessarily assuming that they are capturing the complete causal structure in the experiment. The paper shows that this approach is practically viable by providing conditions under which exposure effects can be precisely estimated when the exposures are misspecified. Some important questions remain open.
更多查看译文
关键词
exposure
AI 理解论文
溯源树
样例
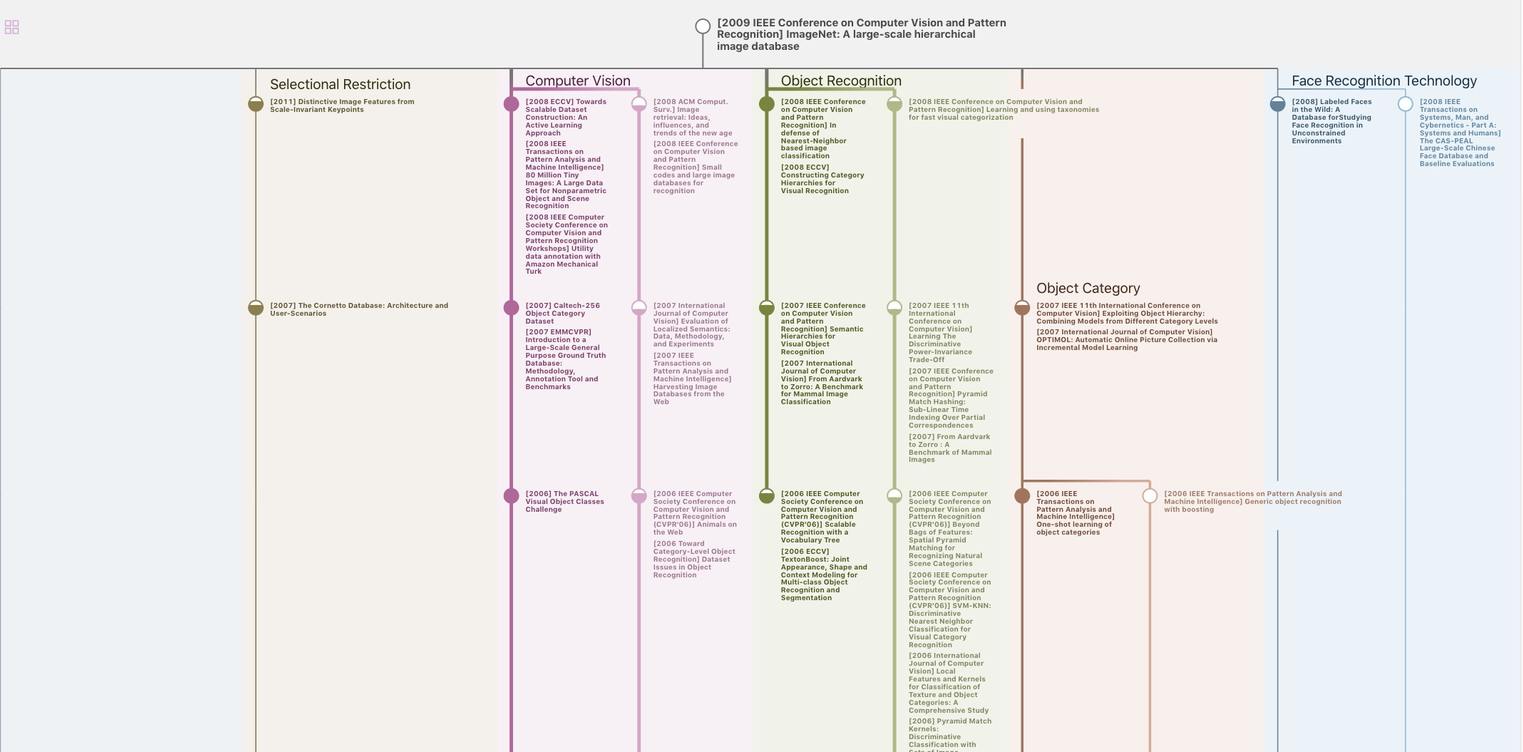
生成溯源树,研究论文发展脉络
Chat Paper
正在生成论文摘要