Machine-learning-assisted high-temperature reservoir thermal energy storage optimization
RENEWABLE ENERGY(2022)
Abstract
High-temperature reservoir thermal energy storage (HT-RTES) has the potential to become an indispensable component in achieving the goal of the net-zero carbon economy, given its capability to balance the intermittent nature of renewable energy generation. In this study, a machine-learning-assisted computational framework is presented to identify HT-RTES site with optimal performance metrics by combining physics-based simulation with stochastic hydrogeologic formation and thermal energy storage operation parameters, artificial neural network regression of the simulation data, and genetic algorithm-enabled multi-objective optimization. A doublet well configuration with a layered (aquitard-aquifer-aquitard) generic reservoir is simulated for cases of continuous operation and seasonal-cycle operation scenarios. Neural network-based surrogate models are developed for the two scenarios and applied to generate the Pareto fronts of the HT-RTES performance for four potential HT-RTES sites. The developed Pareto optimal solutions indicate the performance of HT-RTES is operation-scenario (i.e., fluid cycle) and reservoir-site dependent, and the performance metrics have competing effects for a given site and a given fluid cycle. The developed neural network models can be applied to identify suitable sites for HT-RTES, and the proposed framework sheds light on the design of resilient HT-RTES systems.
MoreTranslated text
Key words
Reservoir thermal energy storage,Multi-objective optimization,Machine learning,Pareto front,Neural network
AI Read Science
Must-Reading Tree
Example
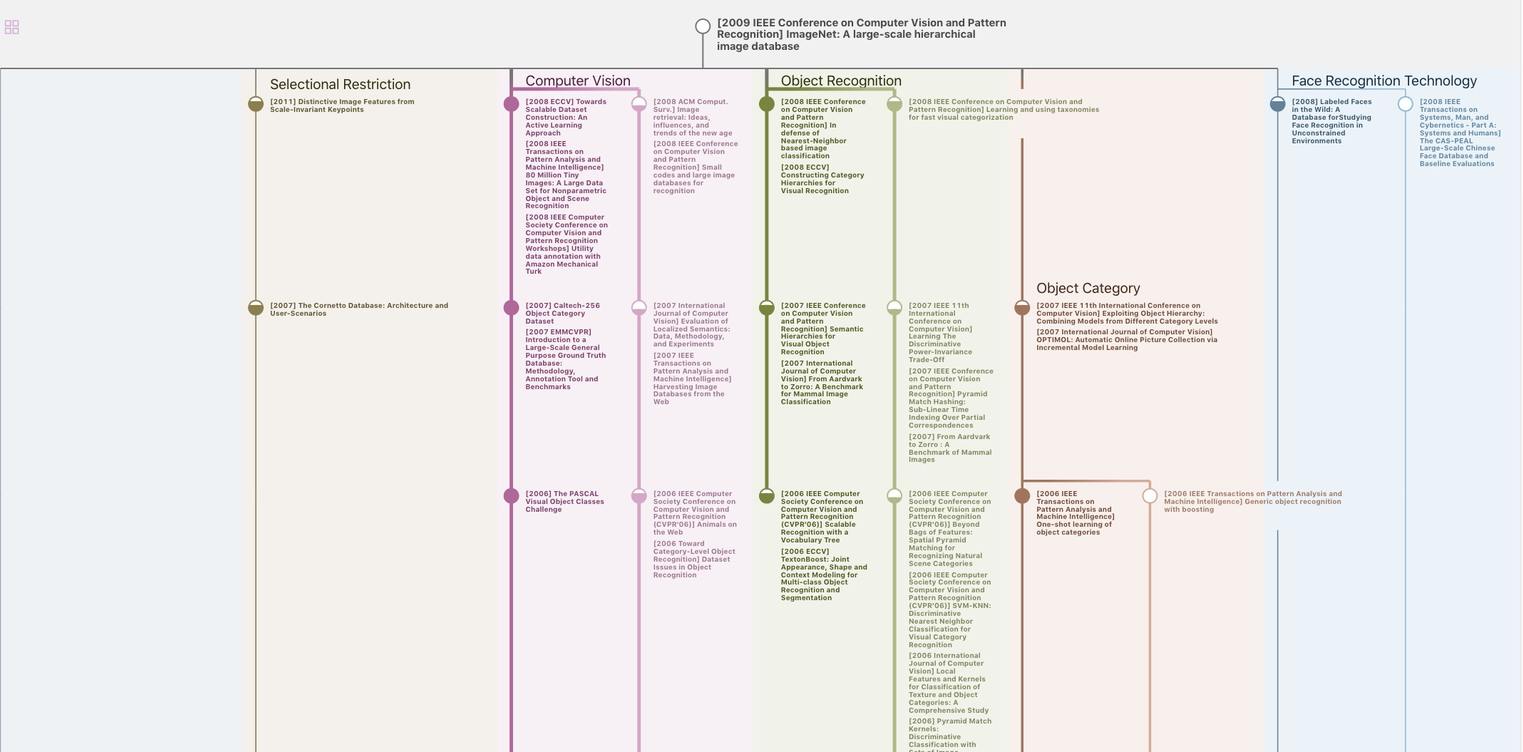
Generate MRT to find the research sequence of this paper
Chat Paper
Summary is being generated by the instructions you defined