Distributionally Robust Data Valuation
ICML 2024(2024)
Abstract
Data valuation quantifies the contribution of each data point to the performance of a machine learning model. Existing works typically define the value of data by its improvement of the validation performance of the trained model. However, this approach can be impractical to apply in collaborative machine learning and data marketplace since it is difficult for the parties/buyers to agree on a common validation dataset or determine the exact validation distributiona priori. To address this, we propose adistributionally robust data valuationapproach to perform data valuation without known/fixed validation distributions. Our approach defines the value of data by its improvement of the distributionally robust generalization error (DRGE), thus providing a worst-case performance guaranteewithouta known/fixed validation distribution. However, since computing DRGE directly is infeasible, we propose usingmodel deviationas a proxy for the marginal improvement of DRGE (for kernel regression and neural networks) to compute data values. Furthermore, we identify a notion of uniqueness where low uniqueness characterizes low-value data. We empirically demonstrate that our approach outperforms existing data valuation approaches in data selection and data removal tasks on real-world datasets (e.g., housing price prediction, diabetes hospitalization prediction).
MoreTranslated text
AI Read Science
Must-Reading Tree
Example
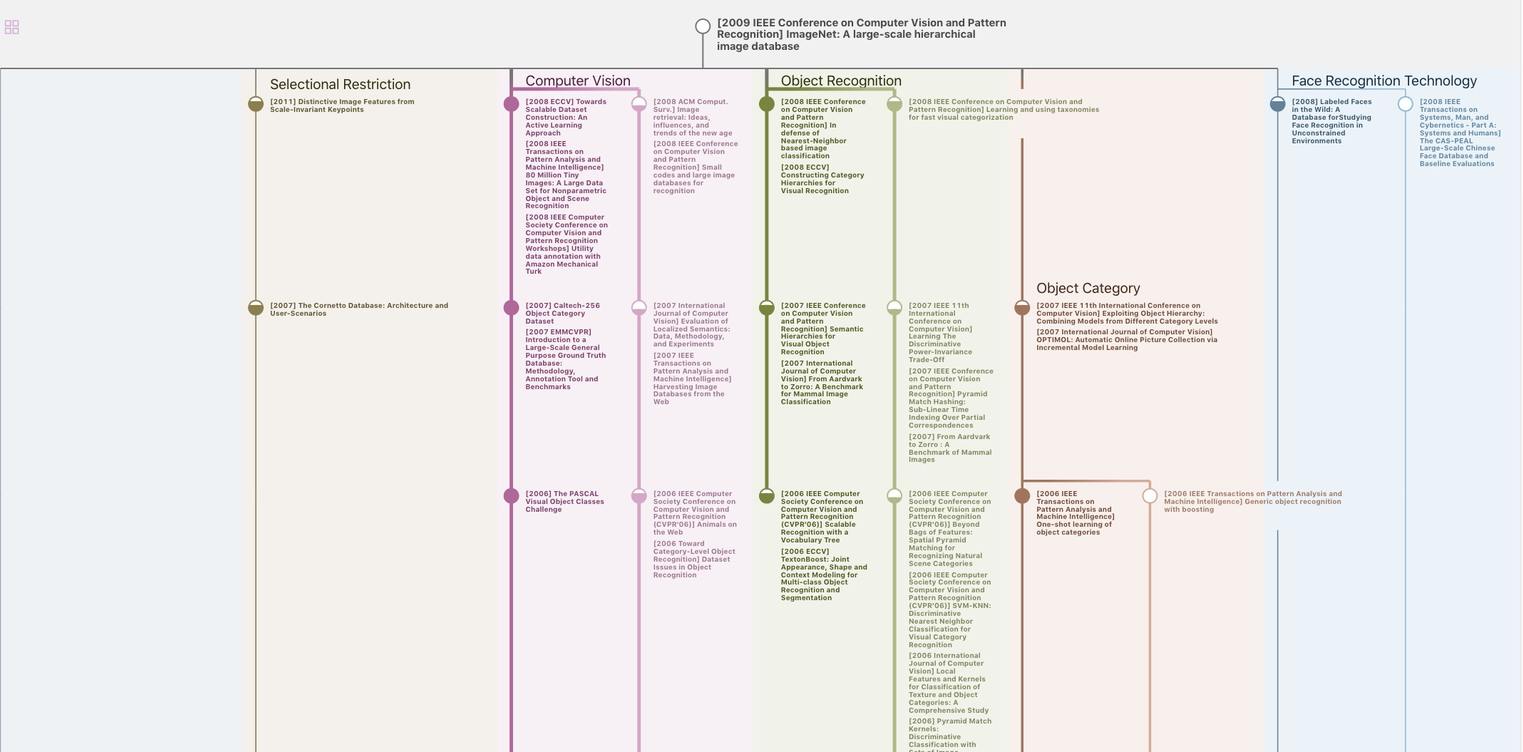
Generate MRT to find the research sequence of this paper
Chat Paper
Summary is being generated by the instructions you defined