Design and Analysis of Bipartite Experiments Under a Linear Exposure-response Model
ACM Conference on Economics and Computation (EC)(2022)
摘要
A bipartite experiment consists of one set of units being assigned treatments and another set of units for which we measure outcomes. The two sets of units are connected by a bipartite graph, governing how the treated units can affect the outcome units. In this paper, we consider estimation of the average total treatment effect in the bipartite experimental framework under a linear exposure-response model. We introduce the Exposure Reweighted Linear (ERL) estimator, and show that the estimator is unbiased, consistent and asymptotically normal, provided that the bipartite graph is sufficiently sparse. To facilitate inference, we introduce an unbiased and consistent estimator of the variance of the ERL point estimator. In addition, we introduce a cluster-based design, Exposure-Design, that uses heuristics to increase the precision of the ERL estimator by realizing a desirable exposure distribution.
更多查看译文
关键词
bipartite experiments,exposure-response
AI 理解论文
溯源树
样例
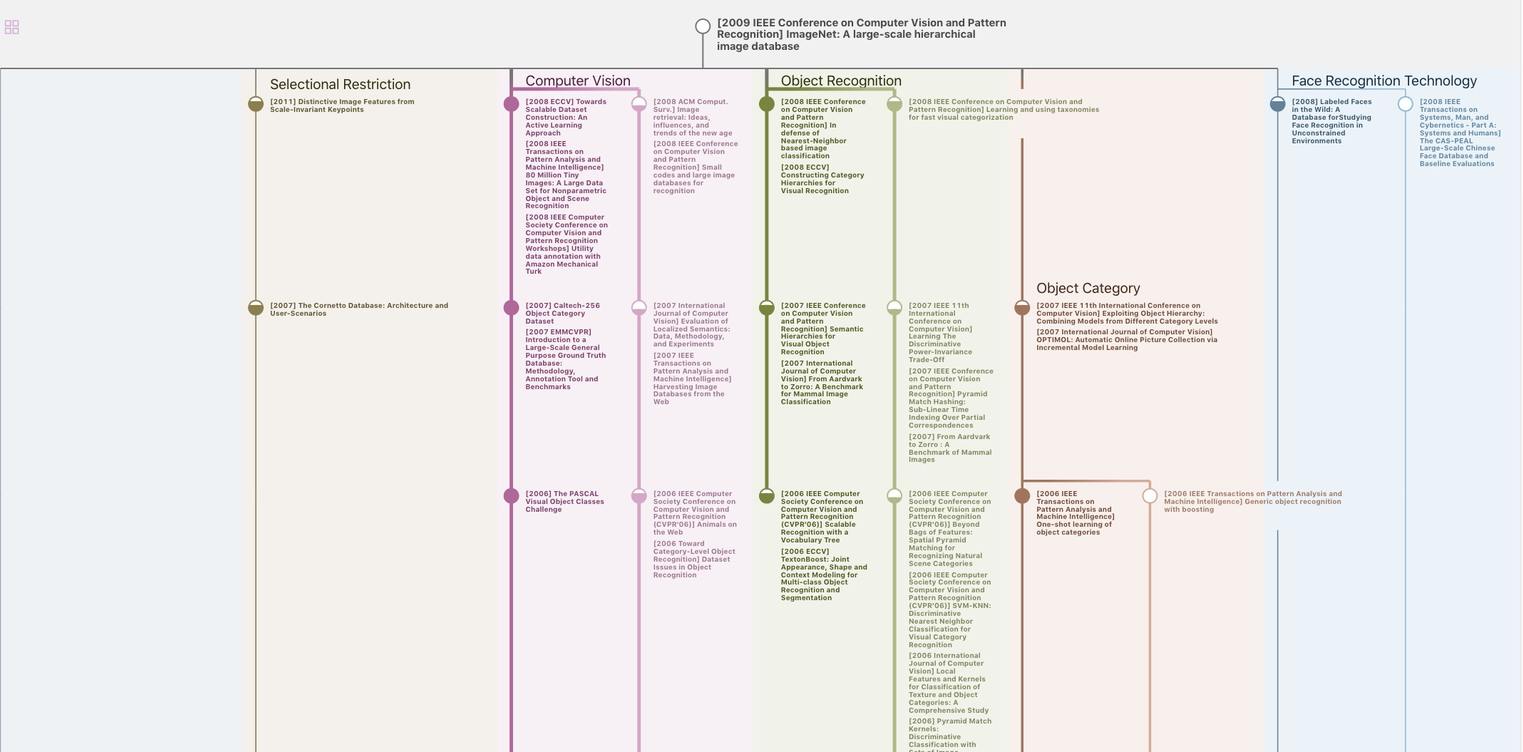
生成溯源树,研究论文发展脉络
Chat Paper
正在生成论文摘要