Research on reinforcement learning-based safe decision-making methodology for multiple unmanned aerial vehicles
FRONTIERS IN NEUROROBOTICS(2023)
Abstract
A system with multiple cooperating unmanned aerial vehicles (multi-UAVs) can use its advantages to accomplish complicated tasks. Recent developments in deep reinforcement learning (DRL) offer good prospects for decision-making for multi-UAV systems. However, the safety and training efficiencies of DRL still need to be improved before practical use. This study presents a transfer-safe soft actor-critic (TSSAC) for multi-UAV decision-making. Decision-making by each UAV is modeled with a constrained Markov decision process (CMDP), in which safety is constrained to maximize the return. The soft actor-critic-Lagrangian (SAC-Lagrangian) algorithm is combined with a modified Lagrangian multiplier in the CMDP model. Moreover, parameter-based transfer learning is used to enable cooperative and efficient training of the tasks to the multi-UAVs. Simulation experiments indicate that the proposed method can improve the safety and training efficiencies and allow the UAVs to adapt to a dynamic scenario.
MoreTranslated text
Key words
multi-UAV,constrained Markov decision process,SAC-Lagrangian,transfer learning,reinforcement learning
AI Read Science
Must-Reading Tree
Example
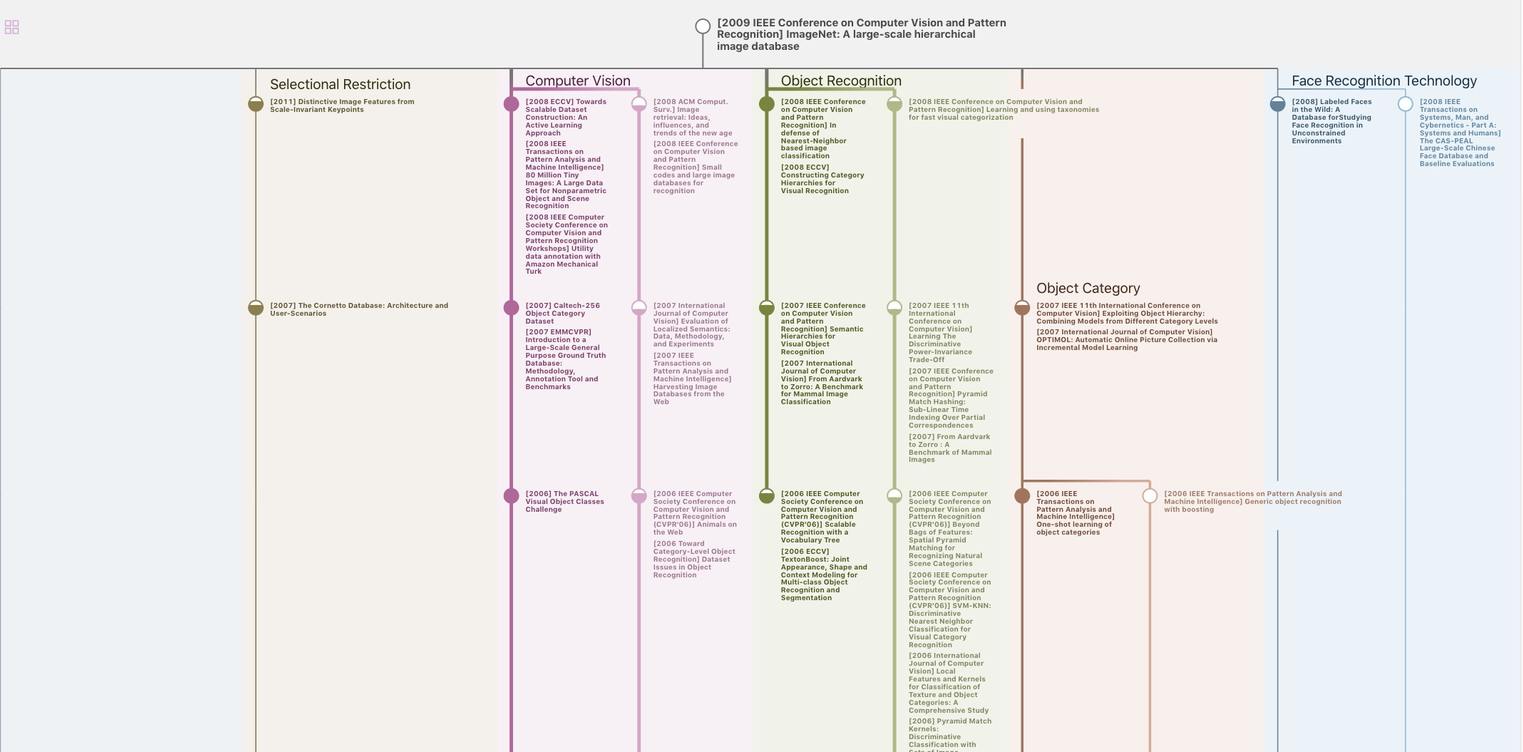
Generate MRT to find the research sequence of this paper
Chat Paper
Summary is being generated by the instructions you defined