Improving Sequential Recommendation with Attribute-Augmented Graph Neural Networks
ADVANCES IN KNOWLEDGE DISCOVERY AND DATA MINING, PAKDD 2021, PT II(2021)
摘要
Many practical recommender systems provide item recommendation for different users only via mining user-item interactions but totally ignoring the rich attribute information of items that users interact with. In this paper, we propose an attribute-augmented graph neural network model named Murzim. Murzim takes as input the graphs constructed from the user-item interaction sequences and corresponding item attribute sequences. By combining the GNNs with node aggregation and an attention network, Murzim can capture user preference patterns, generate embeddings for user-item interaction sequences, and then generate recommendations through next-item prediction. We conduct extensive experiments on multiple datasets. Experimental results show that Murzim outperforms several state-of-the-art methods in terms of recall and MRR, which illustrates that Murzim can make use of item attribute information to produce better recommendations. At present, Murzim has been deployed in MX Player, one of India's largest streaming platforms, and is recommending videos for tens of thousands of users.
更多查看译文
关键词
Recommender system, Deep learning, Graph neural network, Sequential recommendation
AI 理解论文
溯源树
样例
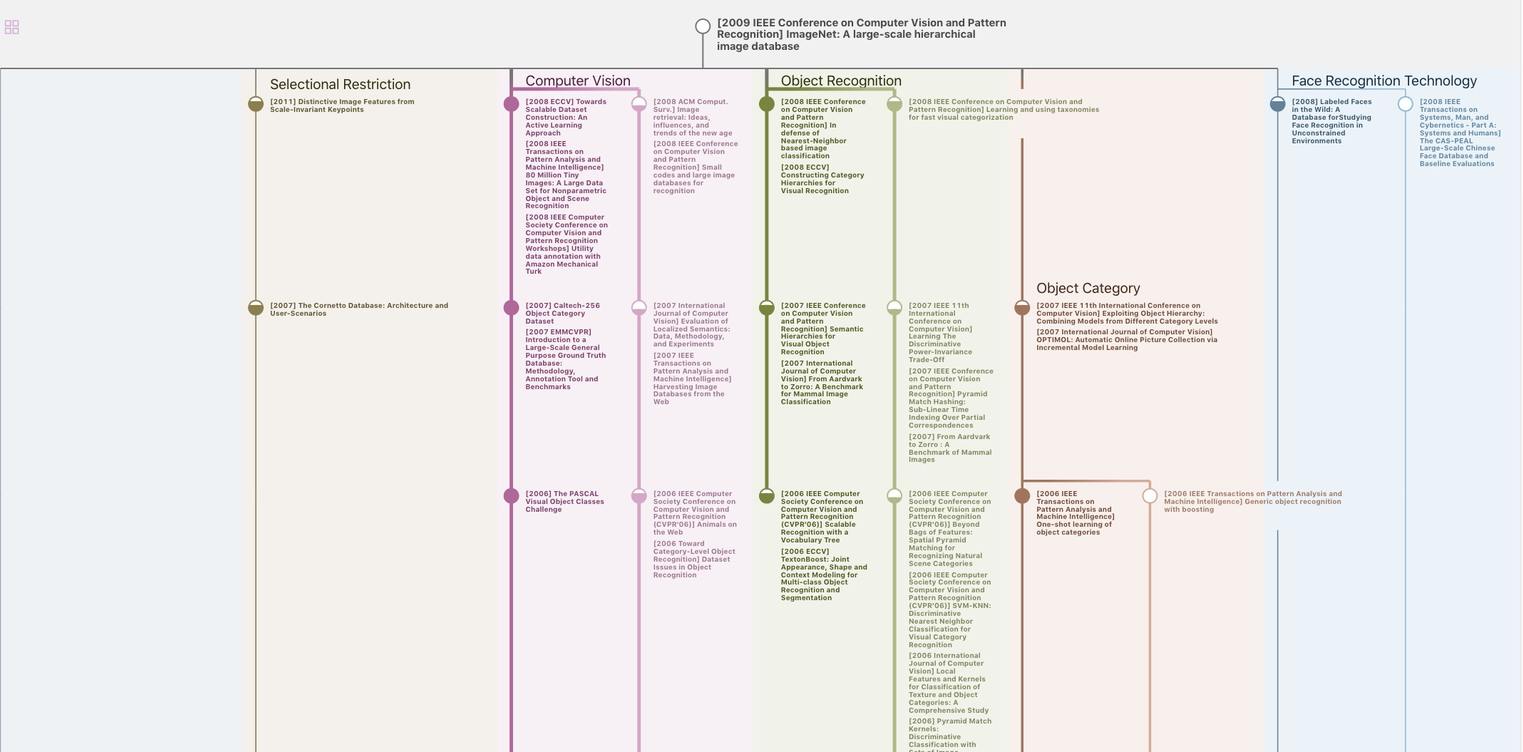
生成溯源树,研究论文发展脉络
Chat Paper
正在生成论文摘要