Robust Black-box Watermarking for Deep Neural Network using Inverse Document Frequency
arxiv(2021)
摘要
Recently, Deep Neural Networks (DNNs), presented exceptional achievement in implementing human-level capabilities for various predicaments, such as Natural Language Processing (NLP), voice recognition, and image processing, etc. Training these models is expensive in terms of computational power and the existence of enough labelled data. Thus, ML-based models such as DNNs establish genuine business value and intellectual property (IP) for their owners. Therefore the trained models need to be protected from any adversary attacks such as illegal redistribution, reproducing, and derivation. Watermarking can be considered as an effective technique for securing a DNN model. However, so far, most of the watermarking algorithms focus on watermarking the DNN by adding noise to an image. To this end, we propose a framework for watermarking a DNN model designed for textual domain. The watermark generation scheme provides a secure watermarking method by combining Term Frequency (TF) and Inverse Document Frequency (IDF) of a particular word. The proposed embedding procedure takes place in the model's training stage, which makes the watermark verification stage straightforward by sending the watermarked document to the trained model. The experimental results show that watermarked models have the same accuracy as the original one, and the proposed framework accurately verifies the ownership of all surrogate models without impairing the performance. The proposed algorithm is robust against well-known attacks such as parameter pruning and brute force attack.
更多查看译文
关键词
deep neuralnetwork,document,black-box
AI 理解论文
溯源树
样例
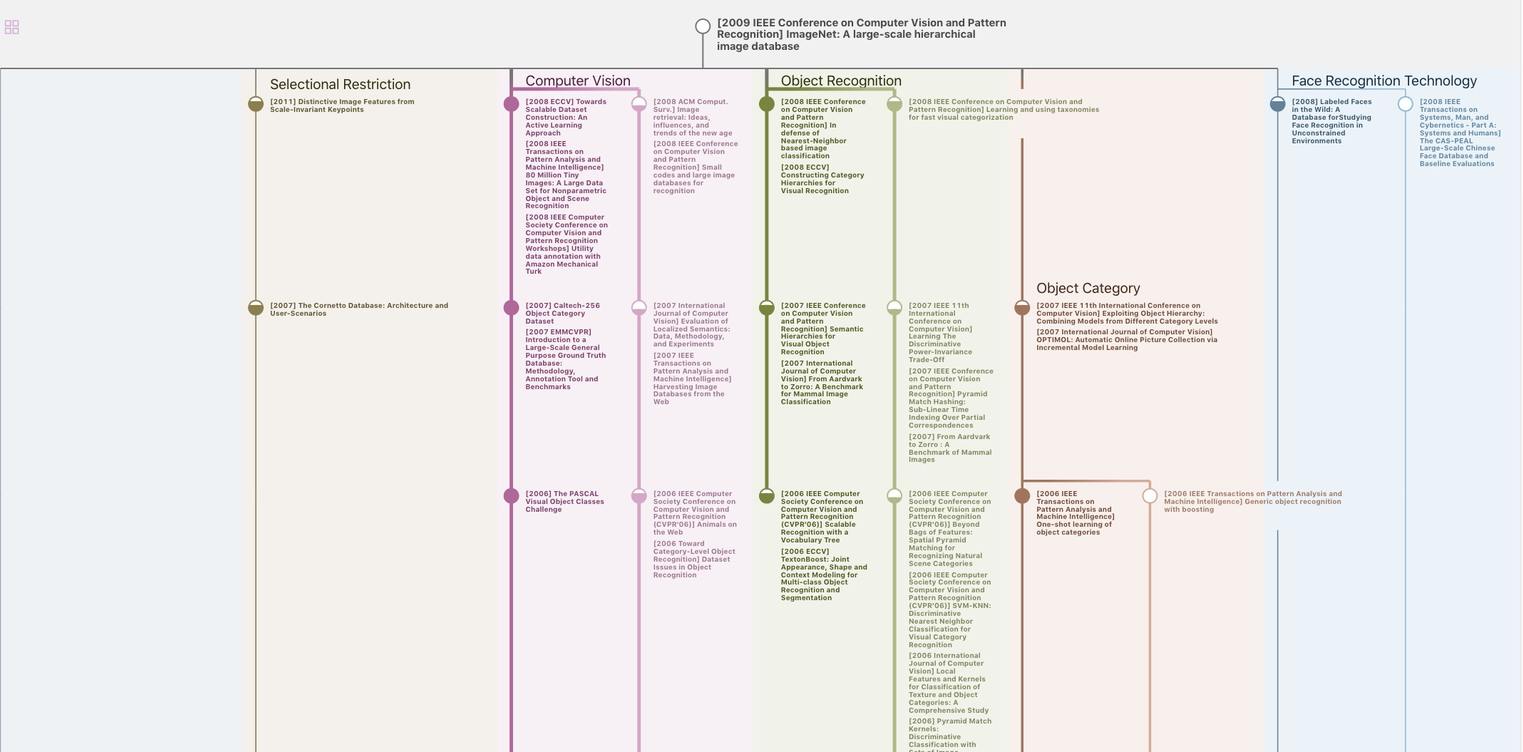
生成溯源树,研究论文发展脉络
Chat Paper
正在生成论文摘要