A Comparative Approach to Explainable Artificial Intelligence Methods in Application to High-Dimensional Electronic Health Records: Examining the Usability of XAI
arxiv(2021)
摘要
Explainable Artificial Intelligence (XAI) is a rising field in AI. It aims to produce a demonstrative factor of trust, which for human subjects is achieved through communicative means, which Machine Learning (ML) algorithms cannot solely produce, illustrating the necessity of an extra layer producing support to the model output. When approaching the medical field, we can see challenges arise when dealing with the involvement of human-subjects, the ideology behind trusting a machine to tend towards the livelihood of a human poses an ethical conundrum - leaving trust as the basis of the human-expert in acceptance to the machines decision. The aim of this paper is to apply XAI methods to demonstrate the usability of explainable architectures as a tertiary layer for the medical domain supporting ML predictions and human-expert opinion, XAI methods produce visualization of the feature contribution towards a given models output on both a local and global level. The work in this paper uses XAI to determine feature importance towards high-dimensional data-driven questions to inform domain-experts of identifiable trends with a comparison of model-agnostic methods in application to ML algorithms. The performance metrics for a glass-box method is also provided as a comparison against black-box capability for tabular data. Future work will aim to produce a user-study using metrics to evaluate human-expert usability and opinion of the given models.
更多查看译文
关键词
explainable artificial intelligence methods,artificial intelligence,usability,xai,high-dimensional
AI 理解论文
溯源树
样例
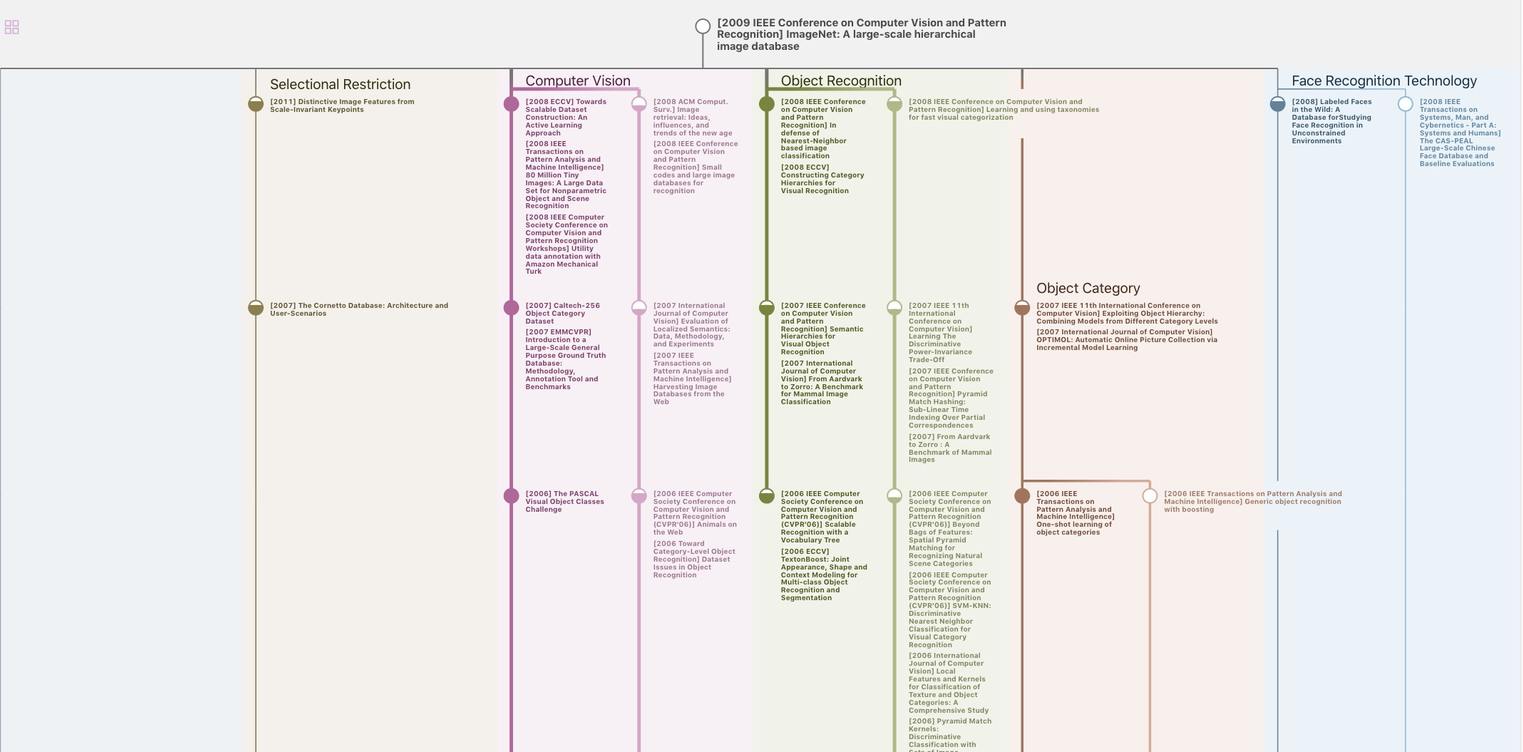
生成溯源树,研究论文发展脉络
Chat Paper
正在生成论文摘要